ESammon: A Computationaly Enhanced Sammon Mapping based on Data Density.
2016 International Conference on Computing, Networking and Communications (ICNC)(2016)
摘要
Sammon mapping is a widely used visualization technique to display complex data from high- to low-dimensional space. However, its extensive computational cost may pose potential computational challenges to big data visualization. This paper proposes a computationally-enhanced Sammon mapping (ESammon) by leveraging the characteristics of spatial data density. Unlike the conventional Sammon, ESammon preserves critical pairwise distances between data points in the process of projection, instead of all distances. Specifically, we integrated the Directed-Acyclic-Graph (DAG) based data density characterization method to select the critical distances. The numerical results demonstrated that our ESammon can achieve comparable projection results as the conventional Sammon mapping while reducing the computational cost from () ().
更多查看译文
关键词
Multidimensional scaling (MDS),Sammon mapping,data density
AI 理解论文
溯源树
样例
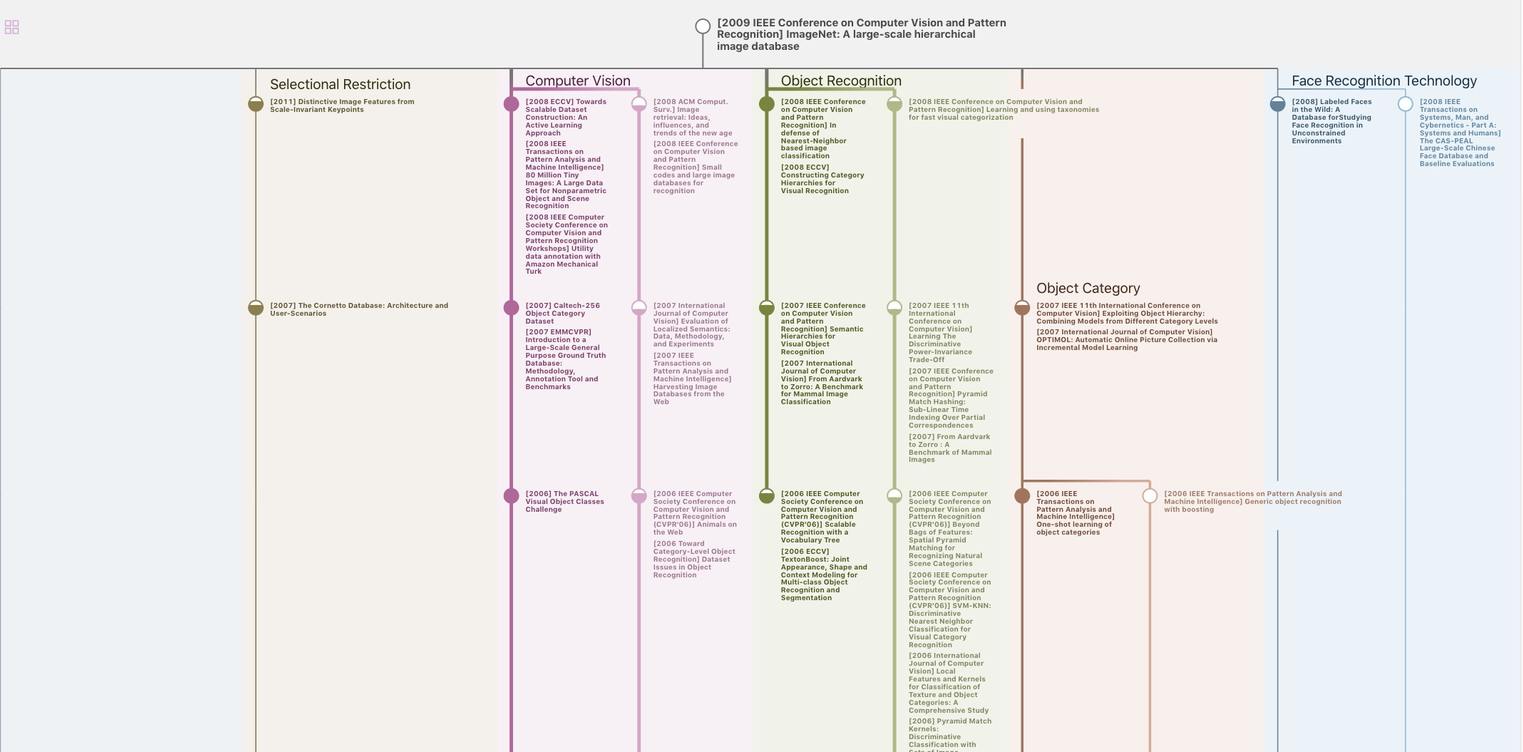
生成溯源树,研究论文发展脉络
Chat Paper
正在生成论文摘要