Learning speech emotion features by joint disentangling-discrimination
ACII(2015)
摘要
Speech plays an important part in human-computer interaction. As a major branch of speech processing, speech emotion recognition (SER) has drawn much attention of researchers. Excellent discriminant features are of great importance in SER. However, emotion-specific features are commonly mixed with some other features. In this paper, we introduce an approach to pull apart these two parts of features as much as possible. First we employ an unsupervised feature learning framework to achieve some rough features. Then these rough features are further fed into a semi-supervised feature learning framework. In this phase, efforts are made to disentangle the emotion-specific features and some other features by using a novel loss function, which combines reconstruction penalty, orthogonal penalty, discriminative penalty and verification penalty. Orthogonal penalty is utilized to disentangle emotion-specific features and other features. The discriminative penalty enlarges inter-emotion variations, while the verification penalty reduces the intra-emotion variations. Evaluations on the FAU Aibo emotion database show that our approach can improve the speech emotion classification performance.
更多查看译文
关键词
speech emotion recognition, feature learning, FAU Aibo
AI 理解论文
溯源树
样例
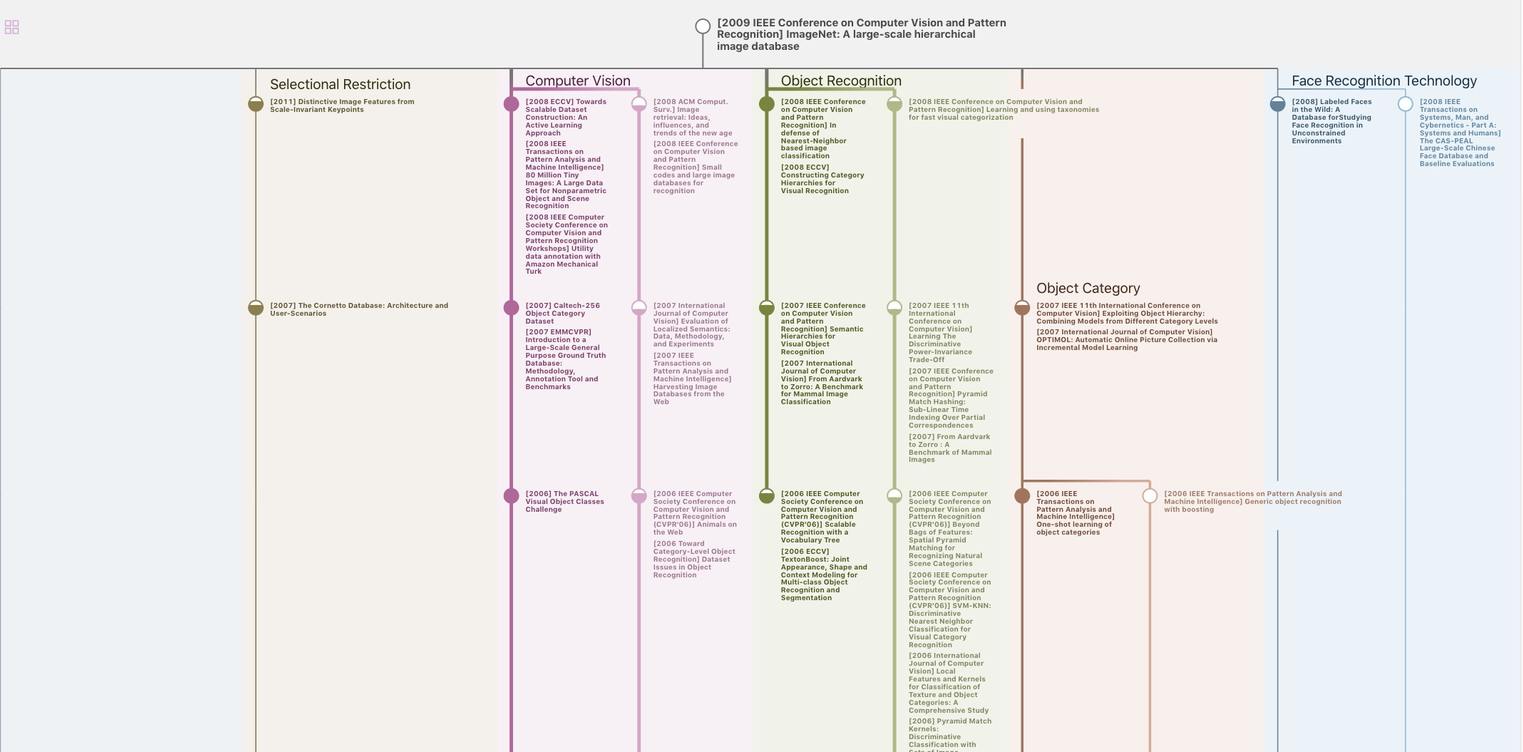
生成溯源树,研究论文发展脉络
Chat Paper
正在生成论文摘要