A Nearest Hyperrectangle Monotonic Learning Method
Hybrid Artificial Intelligent Systems(2016)
摘要
We can find real prediction learning problems whose class attribute is represented by ordinal values that should increase with some of the explaining attributes. They are known as classification problems with monotonicity constraints. In this contribution, our goal is to formalize the nearest hyperrectangle learning approach to manage monotonicity constraints. The idea behind it is to retain objects in R-n, which can be either single points or hyperrectangles or rules into a combined model. The approach is checked with experimental analysis involving wide range of monotonic data sets. The results reported, verified by nonparametric statistical tests, show that our approach is very competitive with wellknown techniques for monotonic classification.
更多查看译文
关键词
Monotonic classification, Instance-based learning, Rule induction, Nested generalized examples
AI 理解论文
溯源树
样例
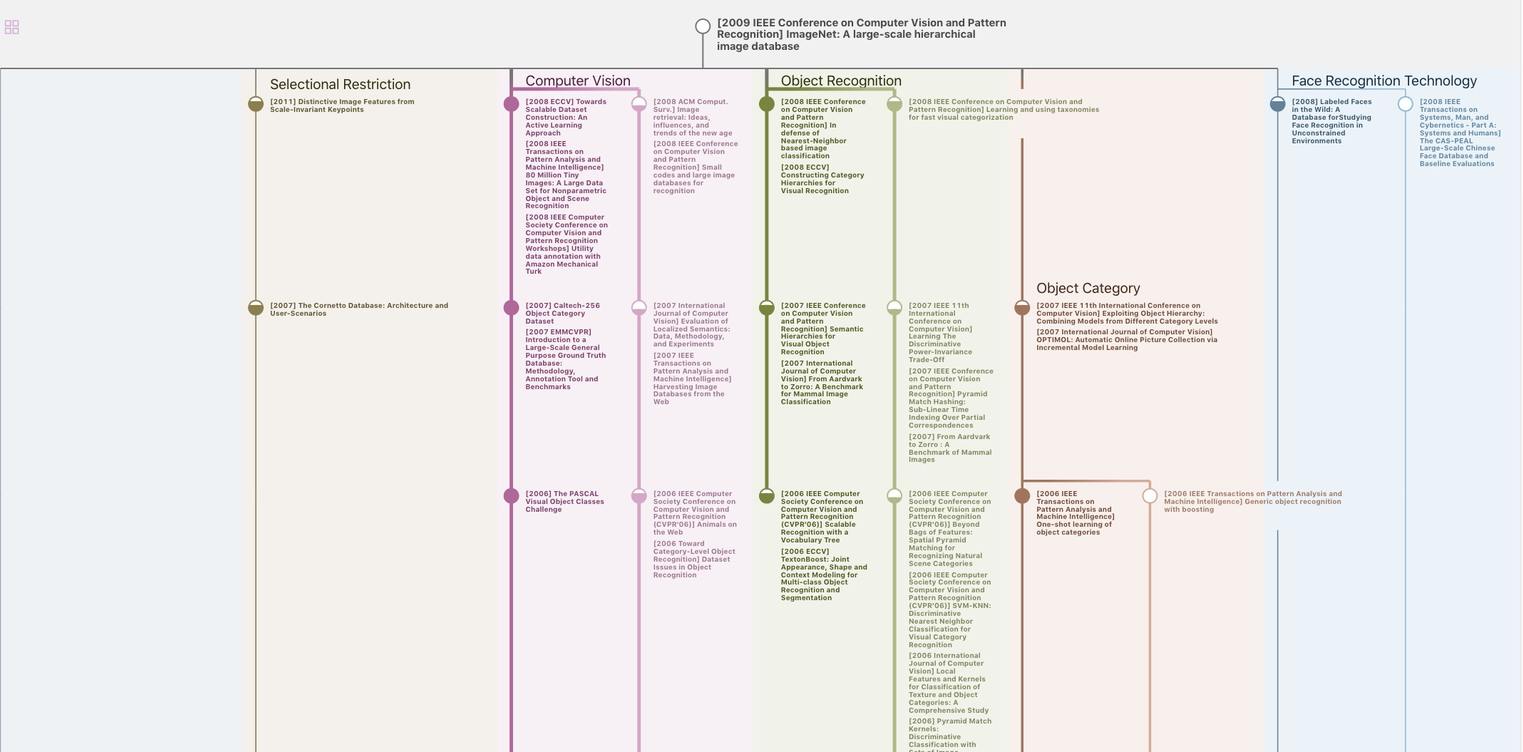
生成溯源树,研究论文发展脉络
Chat Paper
正在生成论文摘要