Segugio: Efficient Behavior-Based Tracking of Malware-Control Domains in Large ISP Networks
The International Conference on Dependable Systems and Networks(2015)
摘要
In this paper, we propose Segugio, a novel defense system that allows for efficiently tracking the occurrence of new malware-control domain names in very large ISP networks. Segugio passively monitors the DNS traffic to build a machine-domain bipartite graph representing who is querying what. After labelling nodes in this query behavior graph that are known to be either benign or malware-related, we propose a novel approach to accurately detect previously unknown malware-control domains. We implemented a proof-of-concept version of Segugio and deployed it in large ISP networks that serve millions of users. Our experimental results show that Segugio can track the occurrence of new malware-control domains with up to 94% true positives (TPs) at less than 0.1% false positives (FPs). In addition, we provide the following results: (1) we show that Segugio can also detect control domains related to new, previously unseen malware families, with 85% TPs at 0.1% FPs, (2) Segugio's detection models learned on traffic from a given ISP network can be deployed into a different ISP network and still achieve very high detection accuracy, (3) new malware-control domains can be detected days or even weeks before they appear in a large commercial domain name blacklist, and (4) we show that Segugio clearly outperforms Notos, a previously proposed domain name reputation system.
更多查看译文
关键词
Malware-control Domains,DNS,Graph Learning,Behavioral Learning,Large-scale Data Analysis
AI 理解论文
溯源树
样例
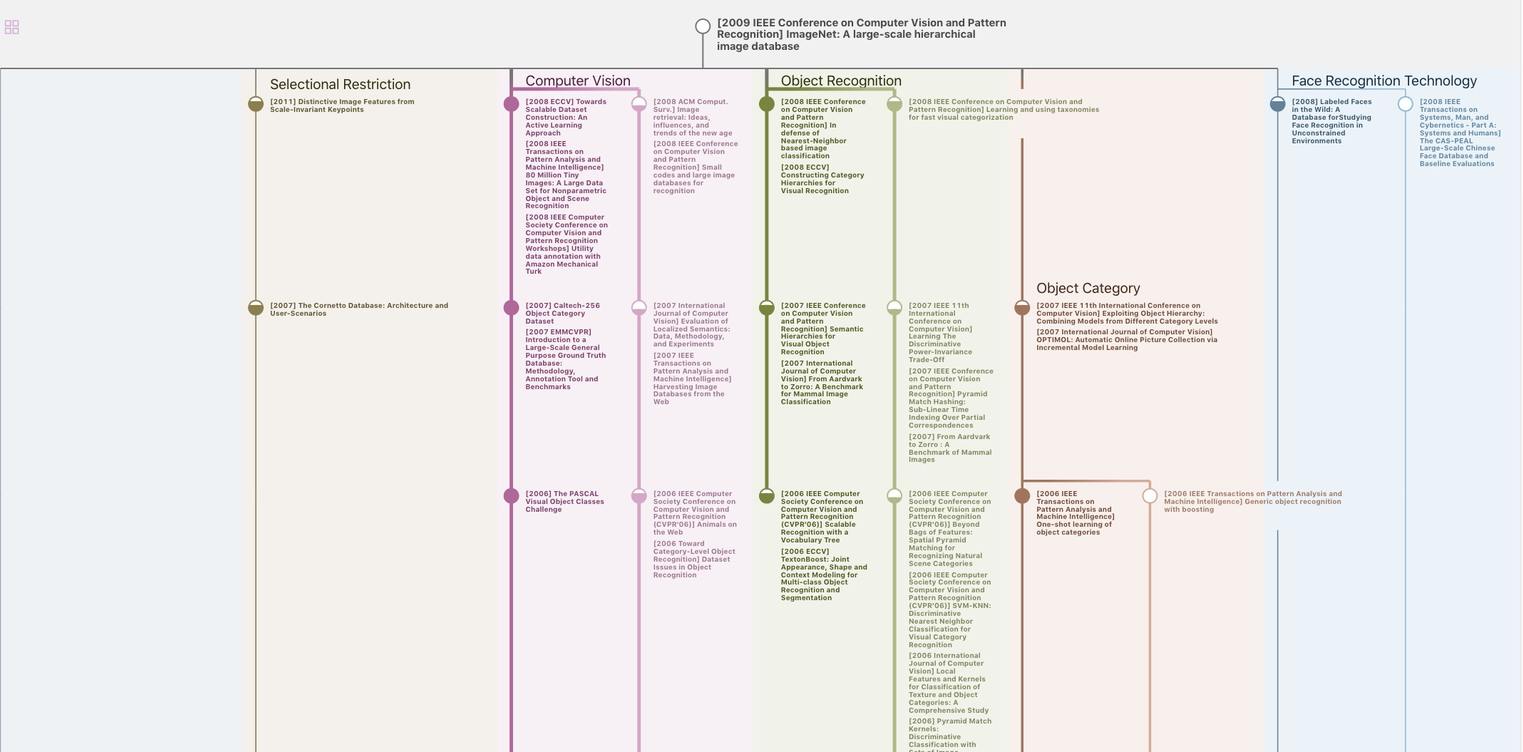
生成溯源树,研究论文发展脉络
Chat Paper
正在生成论文摘要