Deep learning based FACS Action Unit occurrence and intensity estimation
2015 11th IEEE International Conference and Workshops on Automatic Face and Gesture Recognition (FG)(2015)
摘要
Ground truth annotation of the occurrence and intensity of FACS Action Unit (AU) activation requires great amount of attention. The efforts towards achieving a common platform for AU evaluation have been addressed in the FG 2015 Facial Expression Recognition and Analysis challenge (FERA 2015). Participants are invited to estimate AU occurrence and intensity on a common benchmark dataset. Conventional approaches towards achieving automated methods are to train multiclass classifiers or to use regression models. In this paper, we propose a novel application of a deep convolutional neural network (CNN) to recognize AUs as part of FERA 2015 challenge. The 7 layer network is composed of 3 convolutional layers and a max-pooling layer. The final fully connected layers provide the classification output. For the selected tasks of the challenge, we have trained two different networks for the two different datasets, where one focuses on the AU occurrences and the other on both occurrences and intensities of the AUs. The occurrence and intensity of AU activation are estimated using specific neuron activations of the output layer. This way, we are able to create a single network architecture that could simultaneously be trained to produce binary and continuous classification output.
更多查看译文
关键词
deep learning,FACS action unit occurrence,intensity estimation,ground truth annotation,FACS AU activation,AU occurrence estimation,multiclass classifiers,regression models,deep convolutional neural network,CNN,convolutional layers,max-pooling layer,neuron activations,single network architecture,binary classification output,continuous classification output,FG 2015 Facial Expression Recognition and Analysis challenge
AI 理解论文
溯源树
样例
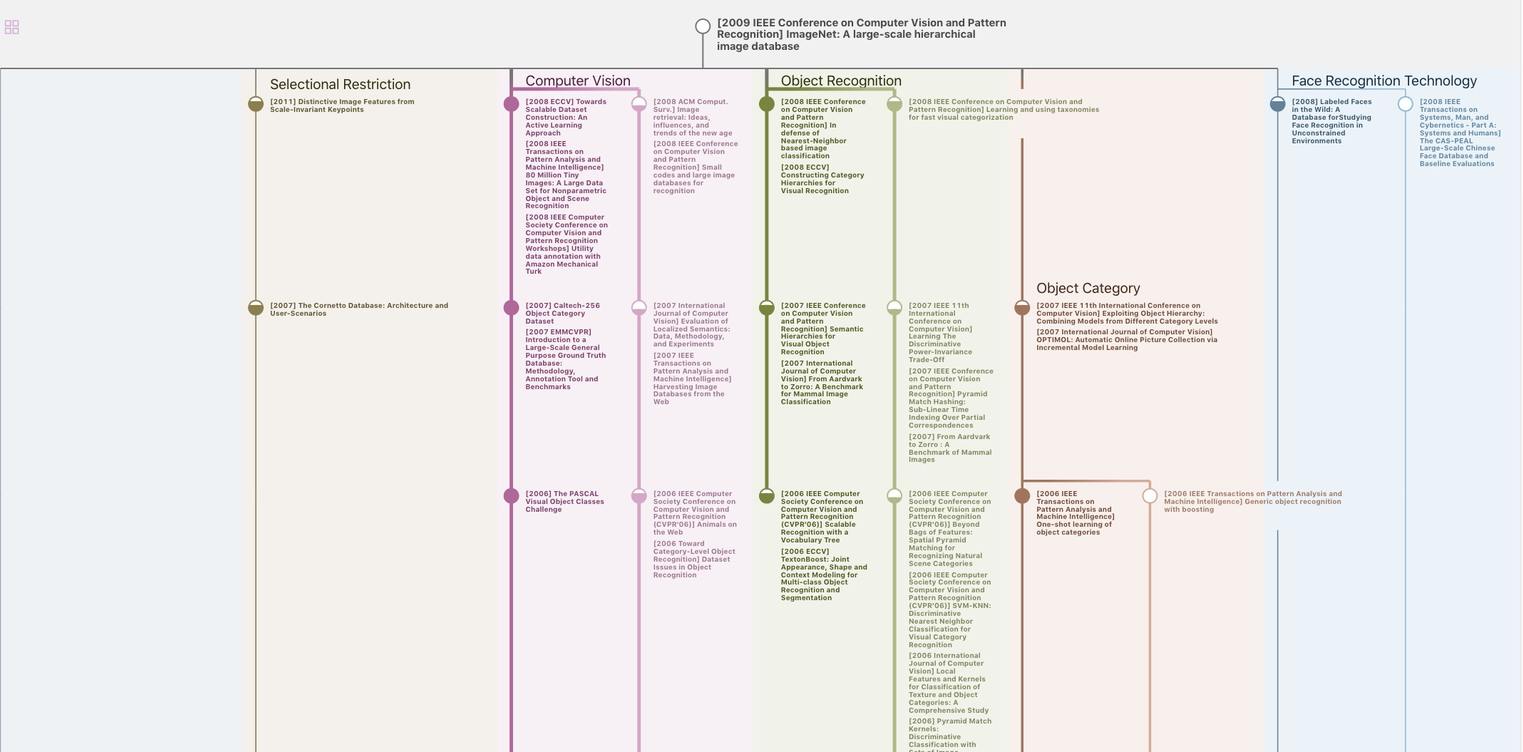
生成溯源树,研究论文发展脉络
Chat Paper
正在生成论文摘要