An Improved Classifier Chain Algorithm For Multi-Label Classification Of Big Data Analysis
2015 IEEE 17th International Conference on High Performance Computing and Communications, 2015 IEEE 7th International Symposium on Cyberspace Safety and Security, and 2015 IEEE 12th International Conference on Embedded Software and Systems(2015)
摘要
The widely known classifier chains method for multi-label classification, which is based on the binary relevance (BR) method, overcomes the disadvantages of BR and achieves higher predictive performance, but still retains important advantages of BR, most importantly low time complexity. Nevertheless, despite its advantages, it is clear that a randomly arranged chain can be poorly ordered. We overcome this issue with a different strategy: Several times K-means algorithms are employed to get the correlations between labels and to confirm the order of binary classifiers. The algorithm ensure the right correlations be transmitted persistently as great as possible by improve the earlier predictions accuracy. The experimental results on the Reuters-21578 text chat data set and image data set show that the approach is efficient and appealing in most cases.
更多查看译文
关键词
improved classifier chain algorithm,multilabel classification,Big Data analysis,binary relevance method,BR method,low time complexity,K-means algorithms,Reuters-21578 text chat data set,image data set
AI 理解论文
溯源树
样例
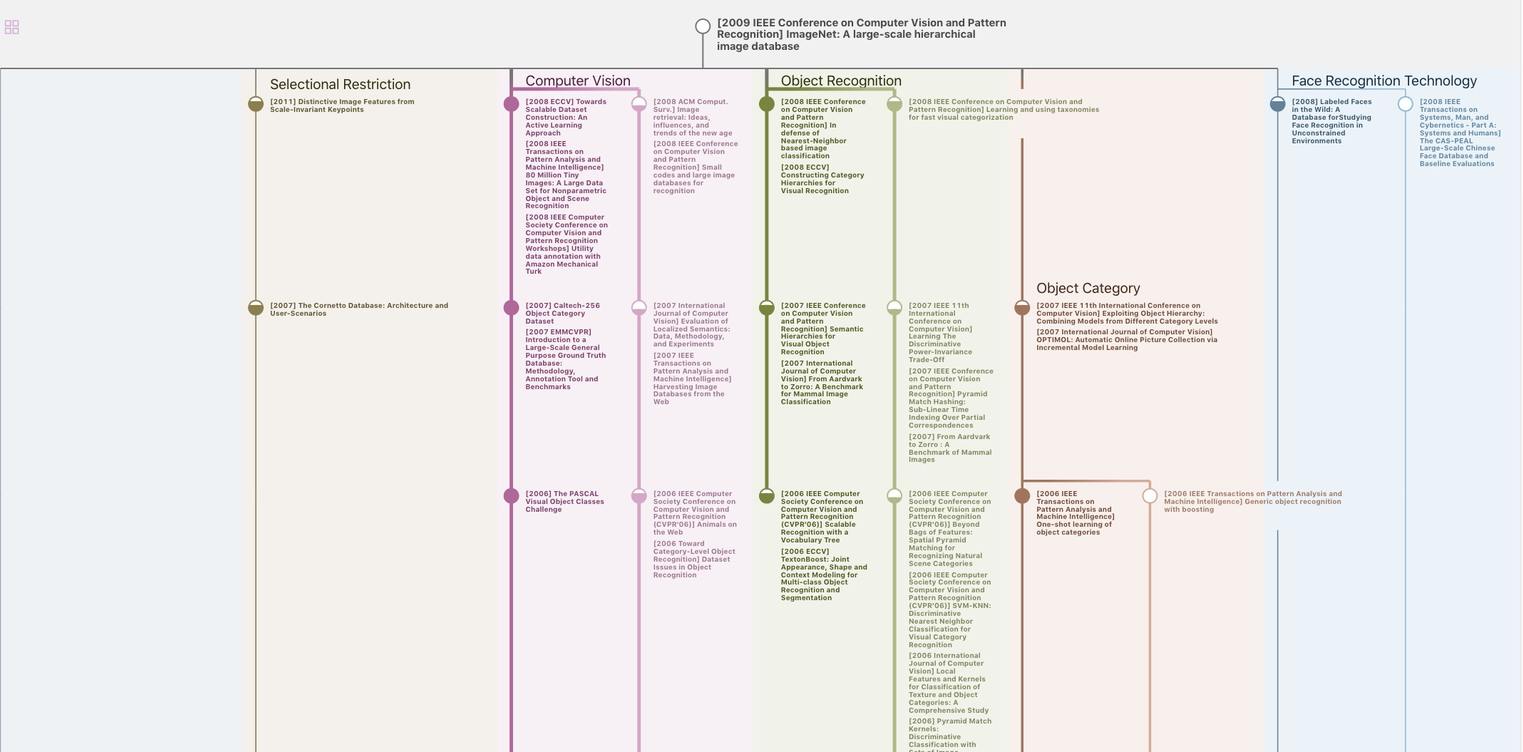
生成溯源树,研究论文发展脉络
Chat Paper
正在生成论文摘要