Fast And Accurate Workload Characterization Using Locality Sensitive Hashing
2015 IEEE 17th International Conference on High Performance Computing and Communications, 2015 IEEE 7th International Symposium on Cyberspace Safety and Security, and 2015 IEEE 12th International Conference on Embedded Software and Systems(2015)
摘要
Embedded applications are increasingly offloading their computations to a cloud data center. Determining an incoming application's sensitivity toward various shared resources is a major challenge. To this end, previous research attempts to characterize an incoming application's sensitivity toward interference on various resources (Source of Interference or SoI, for short) of a cloud system. Due to time constraints, the application's sensitivity is profiled in detail for only a small number of SoI, and the sensitivities for the remaining SoI are approximated by capitalizing on knowledge about some of the applications (i.e. training set) currently running in the system. A key drawback of previous approaches is that they have attempted to minimize the total error of the estimated sensitivities; however, various SoI do not behave the same as each other. For example, a 10% error in the estimate of SoI A may dramatically effect the QoS of an application whereas a 10% error in the estimate of SoI B may have a marginal effect. In this paper, we present a new method for workload characterization that considers these important issues. First, we compute an acceptable error for each SoI based on its effect on QoS, and our goal is to characterize an application so as to maximize the number of SoI that satisfy this acceptable error. Then we present a new technique for workload characterization based on Locality Sensitive Hashing (LSH). Our approach performs better than a state-of-the-art technique in terms of error rate (1.33 times better).
更多查看译文
关键词
Application characterization,data center,locality sensitive hashing
AI 理解论文
溯源树
样例
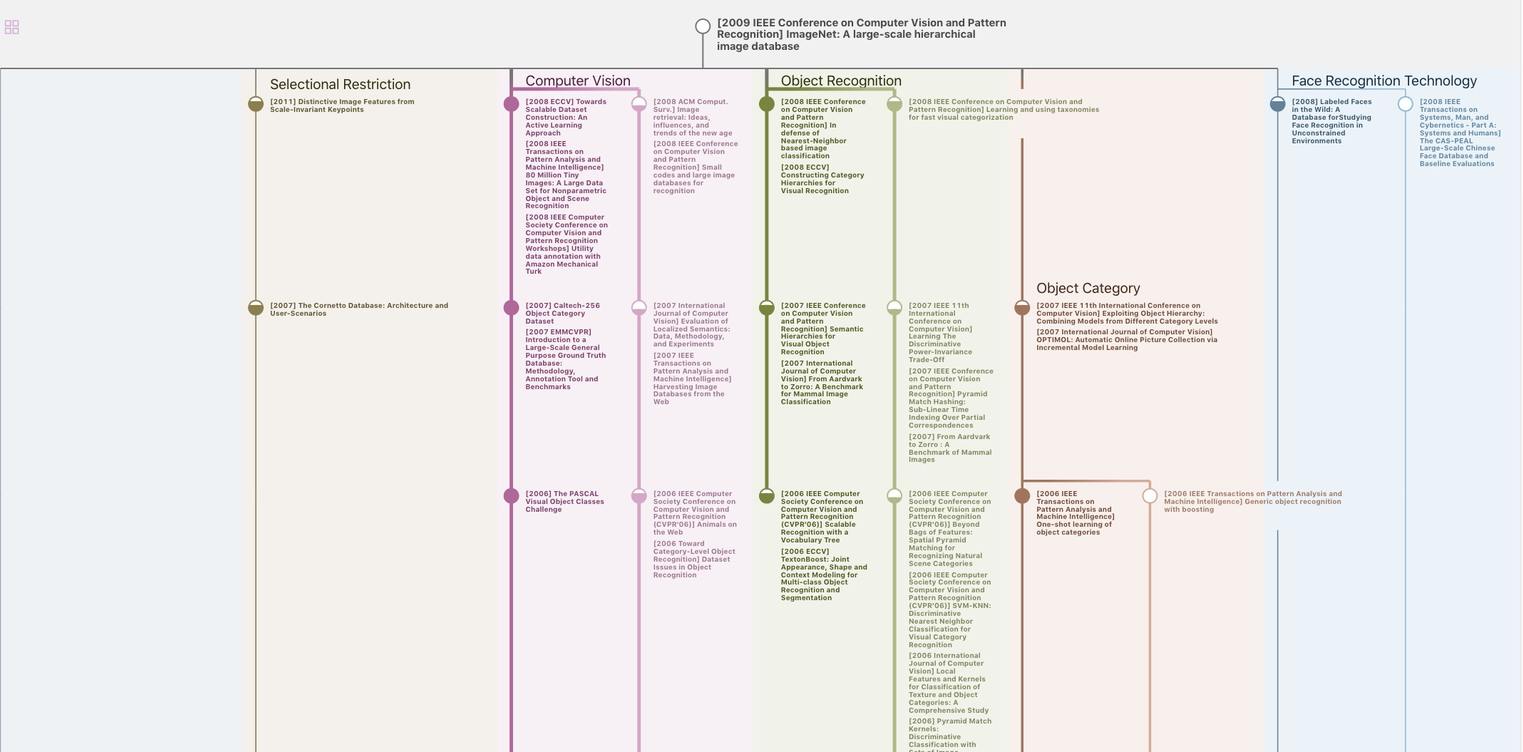
生成溯源树,研究论文发展脉络
Chat Paper
正在生成论文摘要