Data Quality Matters in Recommender Systems.
RECSYS(2015)
摘要
ABSTRACTAlthough data quality has been recognized as an important factor in the broad information systems research, it has received little attention in recommender systems. Data quality matters are typically addressed in recommenders by ad-hoc cleansing methods, which prune noisy or unreliable records from the data. However, the setting of the cleansing parameters is often done arbitrarily, without thorough consideration of the data characteristics. In this work, we turn to two central data quality problems in recommender systems: sparsity and redundancy. We devise models for setting data-dependent thresholds and sampling levels, and evaluate these using a collection of public and proprietary datasets. We observe that the models accurately predict data cleansing parameters, while having minor effect on the accuracy of the generated recommendations.
更多查看译文
AI 理解论文
溯源树
样例
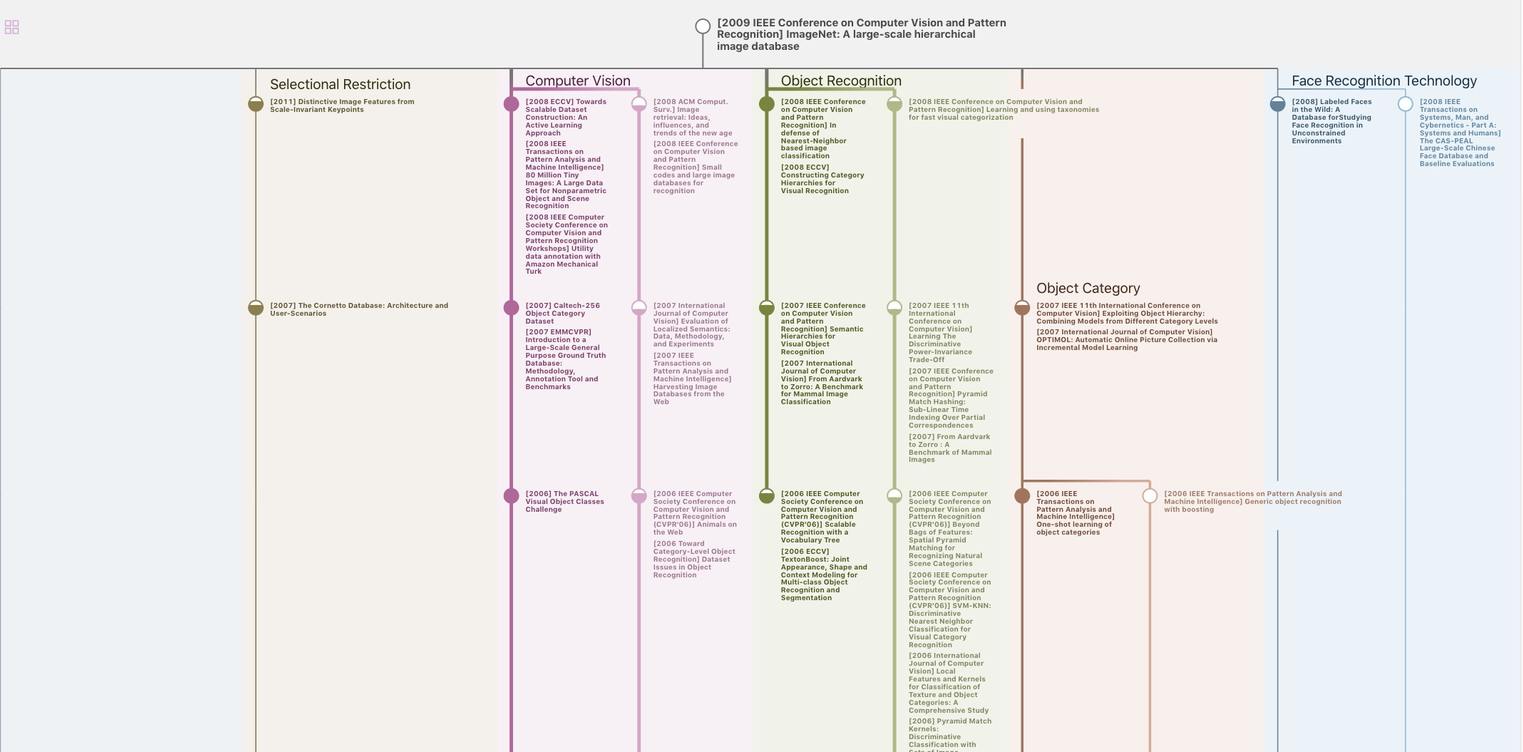
生成溯源树,研究论文发展脉络
Chat Paper
正在生成论文摘要