Non Spontaneous Saccadic Movements Identification in Clinical Electrooculography Using Machine Learning
ADVANCES IN COMPUTATIONAL INTELLIGENCE, PT II(2015)
摘要
In this paper we evaluate the use of the machine learning algorithms Support Vector Machines (SVM), K-Nearest Neighbors (KNN), Classification and Regression Trees (CART) and Naive Bayes (NB) to identify non spontaneous saccades in clinical electrooculography tests. Our approach tries to solve problems like the use of manually established thresholds present in classical methods like identification by velocity threshold (I-VT) or identification by dispersion threshold (I-DT). We propose a modification to an adaptive threshold estimation algorithm for detecting signal impulses without the need of any user input. Also, a set of features were selected to take advantage of intrinsic characteristics of clinical electrooculography tests. The models were evaluated with signals recorded to subjects affected by Spinocerebellar Ataxia type 2 (SCA2). Results obtained by the algorithm show accuracies over 97%, recalls over 97% and precisions over 91% for the four models evaluated.
更多查看译文
关键词
Saccade identification,Clinical electrooculography,Classification
AI 理解论文
溯源树
样例
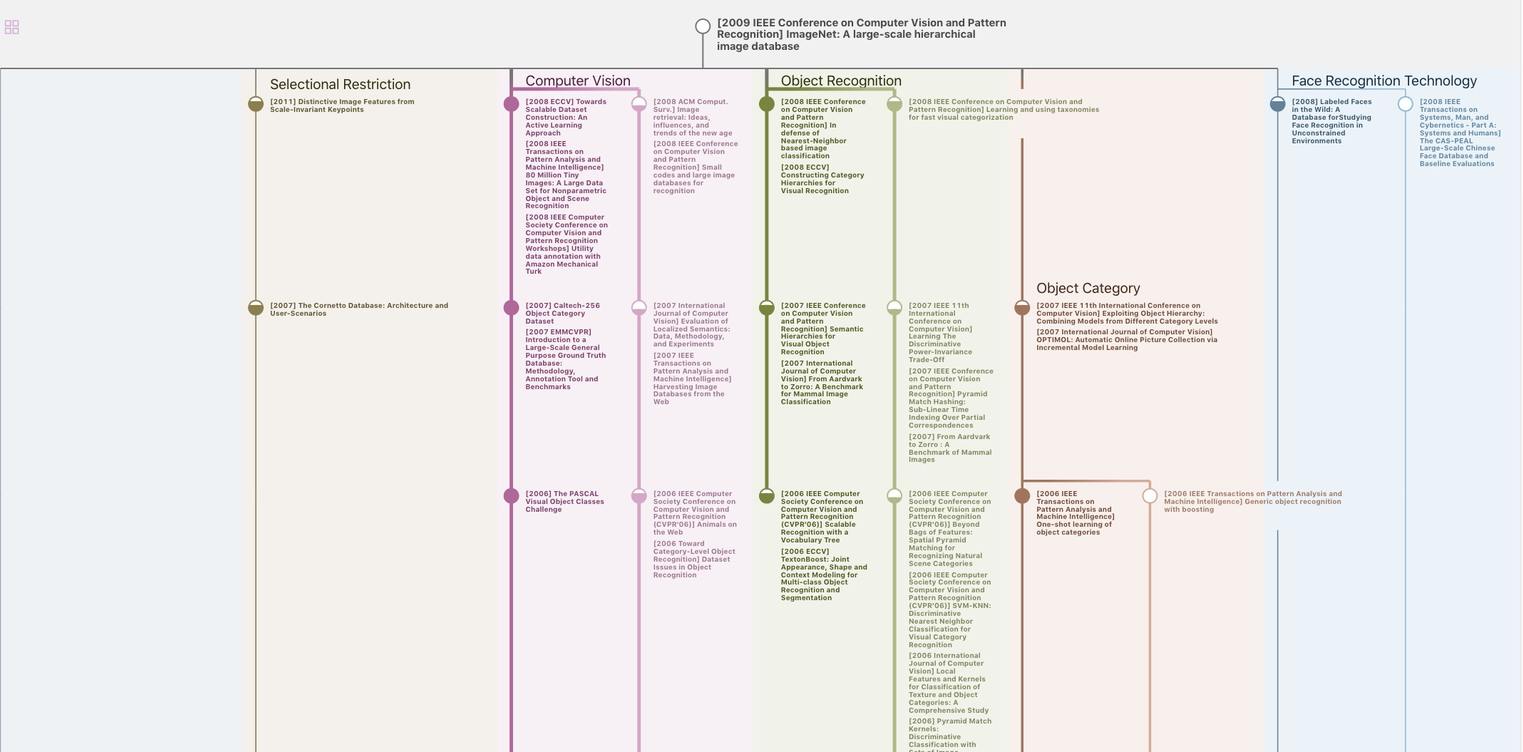
生成溯源树,研究论文发展脉络
Chat Paper
正在生成论文摘要