Mining moving patterns for predicting next location
Information Systems(2015)
摘要
Next location prediction has been an essential task for many location based applications such as targeted advertising. In this paper, we present three basic models to tackle the problem of predicting next locations: the Global Markov Model that uses all available trajectories to discover global behaviors, the Personal Markov Model that focuses on mining the individual patterns of each moving object, and the Regional Markov Model that clusters the trajectories to mine the similar movement patterns. The three models are integrated with linear regression in different ways. We then seek to further improve the accuracy of prediction by considering the time factor, with a focus on clustering the trajectories in different time periods, and present three methods to train the time-aware models to mine periodic patterns. Therefore, our proposed models have the following advantages: (1) we consider both individual and collective movement patterns in making prediction, (2) we consider the similarity between different trajectories, (3) we consider the time factor and build models that are suited to different time periods. We have conducted extensive experiments on a real dataset, and the results demonstrate the superiority of our proposed models over existing methods. HighlightsWe propose three models (PMM, GMM and RMM) and combine them in different ways to obtain new models to predict the next location of a moving object.GMM uses all available trajectories to discover collective patterns; PMM models the individual patterns of each moving object using its own past trajectories; RMM clusters the trajectories to mine the movement patterns.Based on the observation that the movement patterns often change over time, we propose methods that can capture the relationships between the patterns in different time periods, and use this knowledge to build more refined models.To the best of our knowledge, the proposed models are the first ones that take a holistic approach and consider individual, collective movement patterns and the similarity between trajectories in making prediction.We conduct extensive experiments using a real dataset and the results demonstrate the effectiveness of the proposed models.
更多查看译文
关键词
Moving patterns mining,Next location prediction,Time factor
AI 理解论文
溯源树
样例
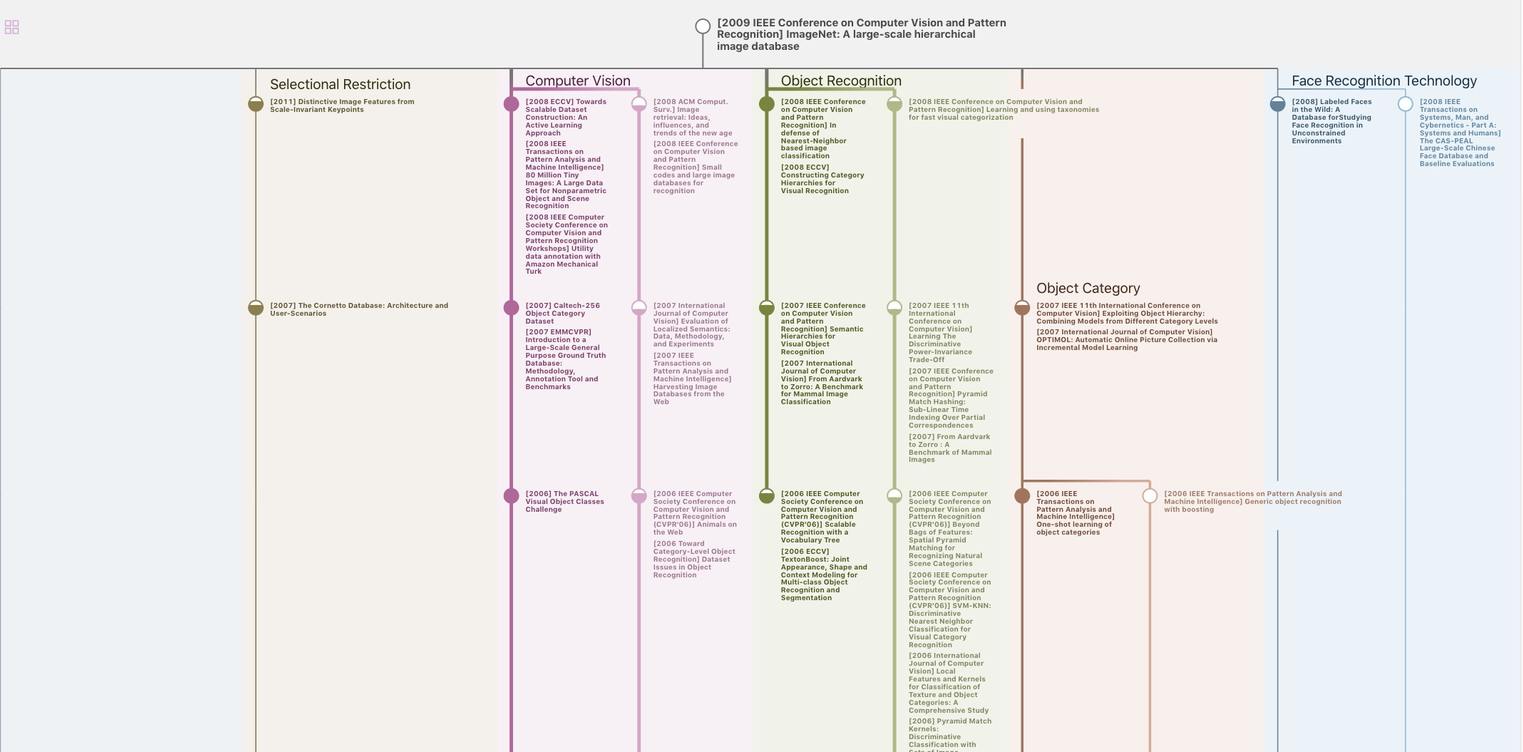
生成溯源树,研究论文发展脉络
Chat Paper
正在生成论文摘要