Performance evaluation of classification algorithms by k -fold and leave-one-out cross validation
Pattern Recognition(2015)
摘要
Classification is an essential task for predicting the class values of new instances. Both k-fold and leave-one-out cross validation are very popular for evaluating the performance of classification algorithms. Many data mining literatures introduce the operations for these two kinds of cross validation and the statistical methods that can be used to analyze the resulting accuracies of algorithms, while those contents are generally not all consistent. Analysts can therefore be confused in performing a cross validation procedure. In this paper, the independence assumptions in cross validation are introduced, and the circumstances that satisfy the assumptions are also addressed. The independence assumptions are then used to derive the sampling distributions of the point estimators for k-fold and leave-one-out cross validation. The cross validation procedure to have such sampling distributions is discussed to provide new insights in evaluating the performance of classification algorithms.
更多查看译文
关键词
Classification,Independence,k-Fold cross validation,Leave-one-out cross validation,Sampling distribution
AI 理解论文
溯源树
样例
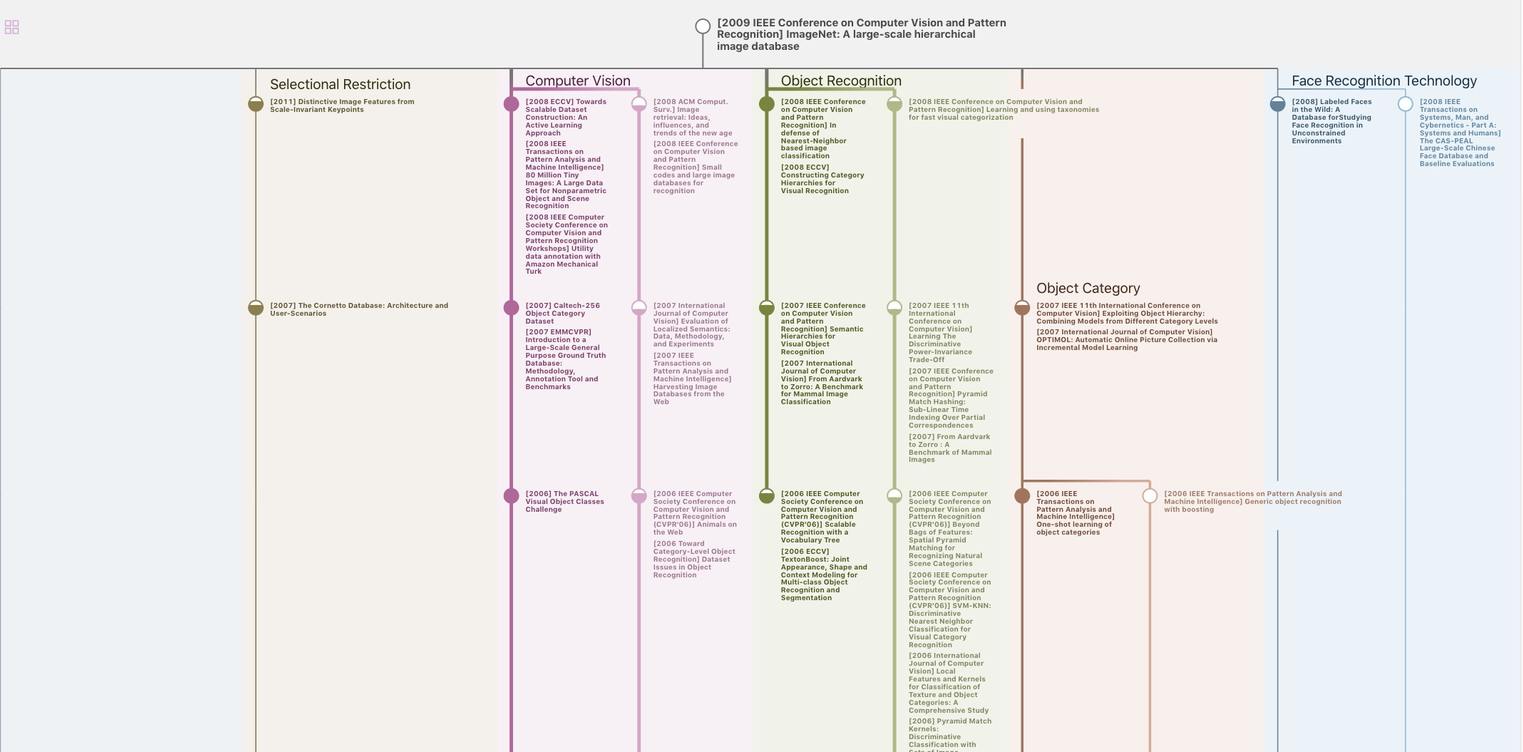
生成溯源树,研究论文发展脉络
Chat Paper
正在生成论文摘要