Combination of global and local contexts for text/non-text classification in heterogeneous online handwritten documents
Pattern Recognition(2016)
摘要
The task of text/non-text classification in online handwritten documents is crucially important to text recognition, text search, and diagram interpretation. It, however, is a challenging problem because of the large amount of variation and lack of prior knowledge. In order to solve this problem, we propose to use global and local contexts to build a high-performance classifier. The classifier assigns a text or non-text label to each stroke in a stroke sequence of a digital ink document. First, a neural network architecture is used to acquire the complete global context of the sequence of strokes. Then, a simple but effective model based on a marginal distribution is used for the local temporal context of adjacent strokes in order to improve the sequence labeling result. The results of experiments on available heterogeneous online handwritten document databases demonstrate the superiority and effectiveness of our context combination approach. Our method achieved classification rates of 99.04% and 98.30% on the Kondate (written in Japanese) and IAMonDo (written in English) heterogeneous document databases. These results are significantly better than others reported in the literature. We present a method to combine global and local contexts for text/non-text classification in online handwritten documents.Global context refers to the feature vector sequence of an entire document and local context refers to the prediction of directly adjacent strokes.Global and local contexts are integrated by using a simple marginal distribution and basic combination rules.We propose multiple classifier combination strategies for combining global and local contexts to improve classification accuracy.
更多查看译文
关键词
Text/non-text classification,Ink stroke classification,Online handwritten documents,Heterogeneous documents,Recurrent neural networks,Long short-term memory
AI 理解论文
溯源树
样例
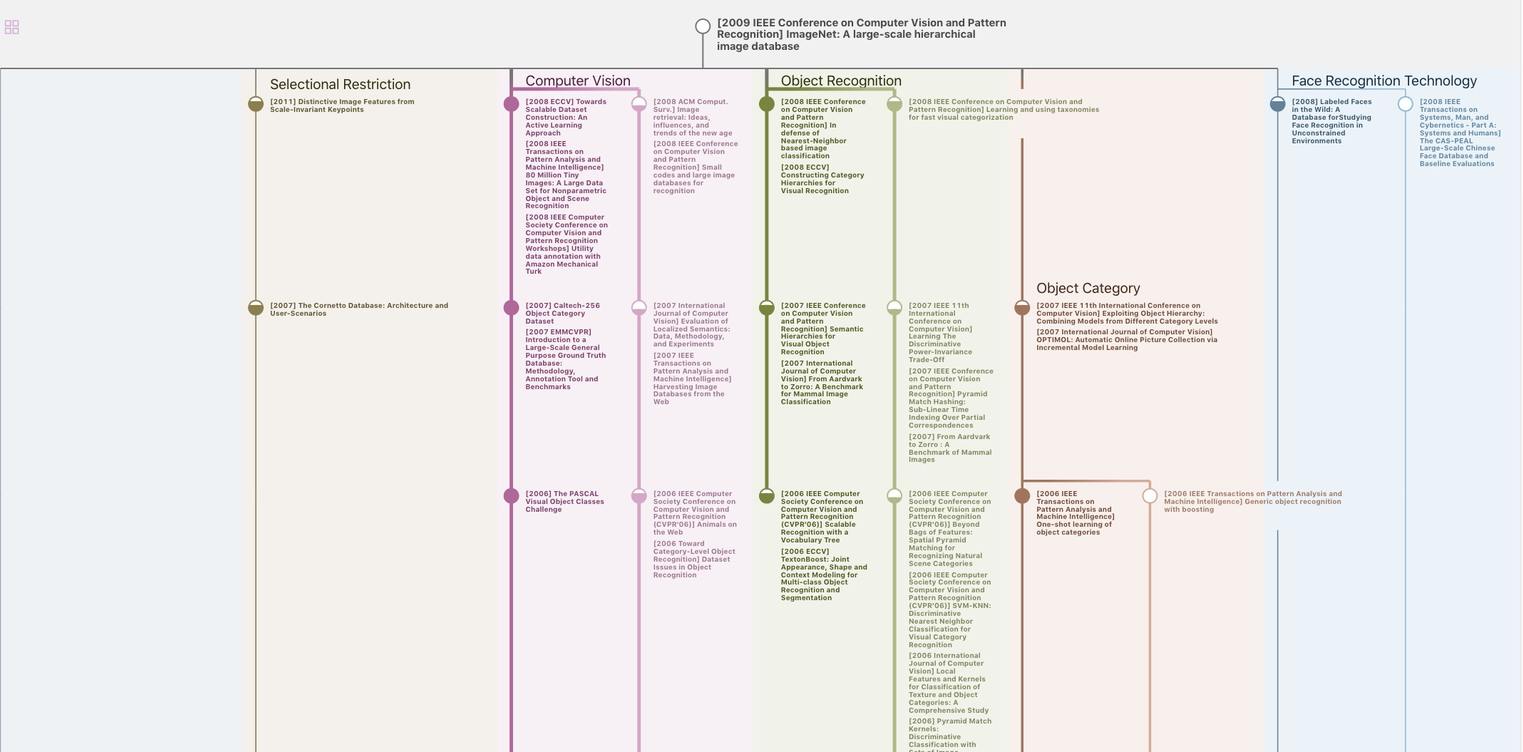
生成溯源树,研究论文发展脉络
Chat Paper
正在生成论文摘要