CRF Learning with CNN Features for Image Segmentation
arXiv (Cornell University)(2015)
摘要
Conditional Random Rields (CRF) have been widely applied in image segmentations. While most studies rely on hand-crafted features, we here propose to exploit a pre-trained large convolutional neural network (CNN) to generate deep features for CRF learning. The deep CNN is trained on the ImageNet dataset and transferred to image segmentations here for constructing potentials of superpixels. Then the CRF parameters are learnt using a structured support vector machine (SSVM). To fully exploit context information in inference, we construct spatially related co-occurrence pairwise potentials and incorporate them into the energy function. This prefers labelling of object pairs that frequently co-occur in a certain spatial layout and at the same time avoids implausible labellings during the inference. Extensive experiments on binary and multi-class segmentation benchmarks demonstrate the promise of the proposed method. We thus provide new baselines for the segmentation performance on the Weizmann horse, Graz-02, MSRC-21, Stanford Background and PASCAL VOC 2011 datasets.
更多查看译文
关键词
Conditional random field (CRF),Convolutional neural network (CNN),Structured support vector machine (SSVM),Co-occurrence
AI 理解论文
溯源树
样例
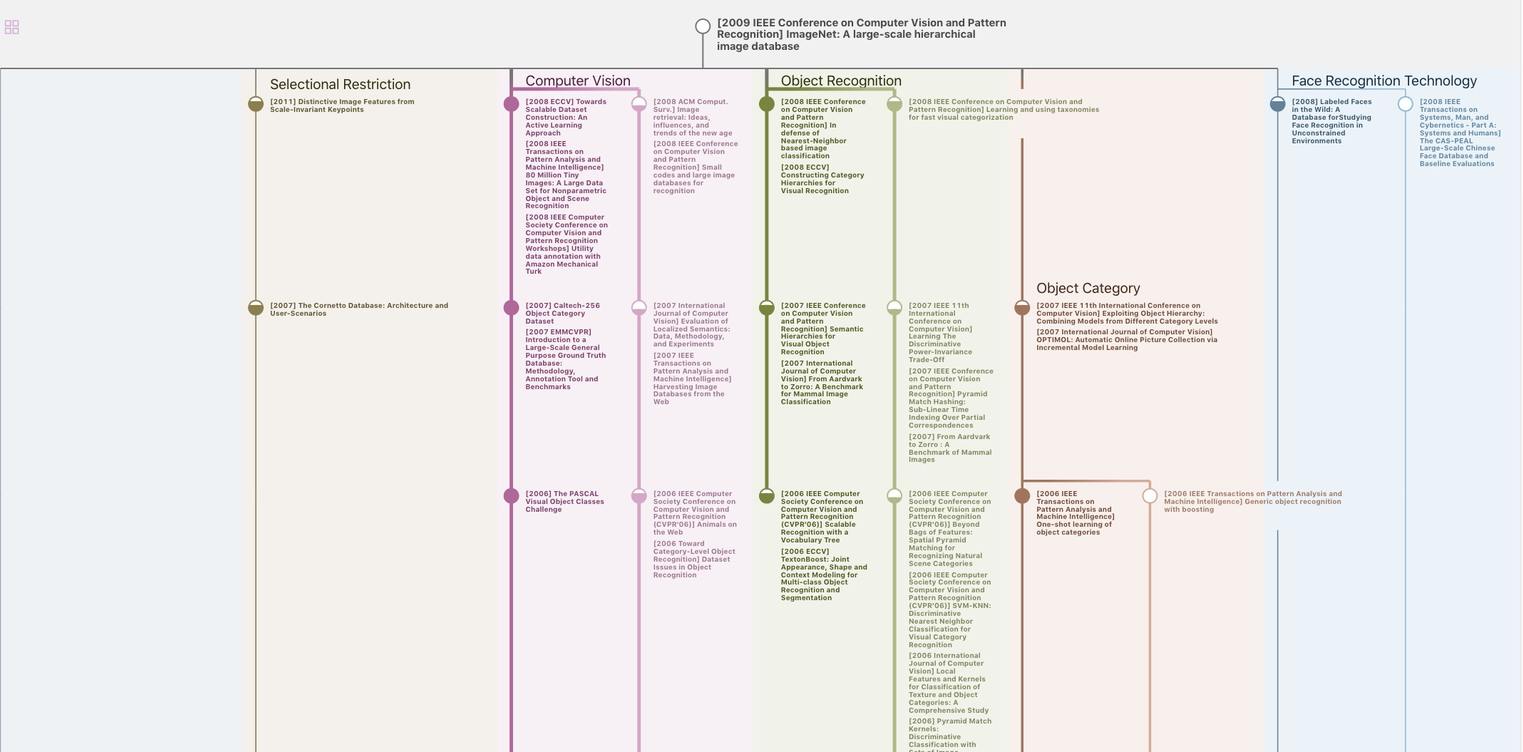
生成溯源树,研究论文发展脉络
Chat Paper
正在生成论文摘要