A Bayesian approach for comparing cross-validated algorithms on multiple data sets
Machine Learning(2015)
摘要
We present a Bayesian approach for making statistical inference about the accuracy (or any other score) of two competing algorithms which have been assessed via cross-validation on multiple data sets. The approach is constituted by two pieces. The first is a novel correlated Bayesian t test for the analysis of the cross-validation results on a single data set which accounts for the correlation due to the overlapping training sets. The second piece merges the posterior probabilities computed by the Bayesian correlated t test on the different data sets to make inference on multiple data sets. It does so by adopting a Poisson-binomial model. The inferences on multiple data sets account for the different uncertainty of the cross-validation results on the different data sets. It is the first test able to achieve this goal. It is generally more powerful than the signed-rank test if ten runs of cross-validation are performed, as it is anyway generally recommended.
更多查看译文
关键词
Bayesian hypothesis tests,Signed-rank test,Cross-validation,Poisson-binomial,Hypothesis test,Evaluation of classifiers
AI 理解论文
溯源树
样例
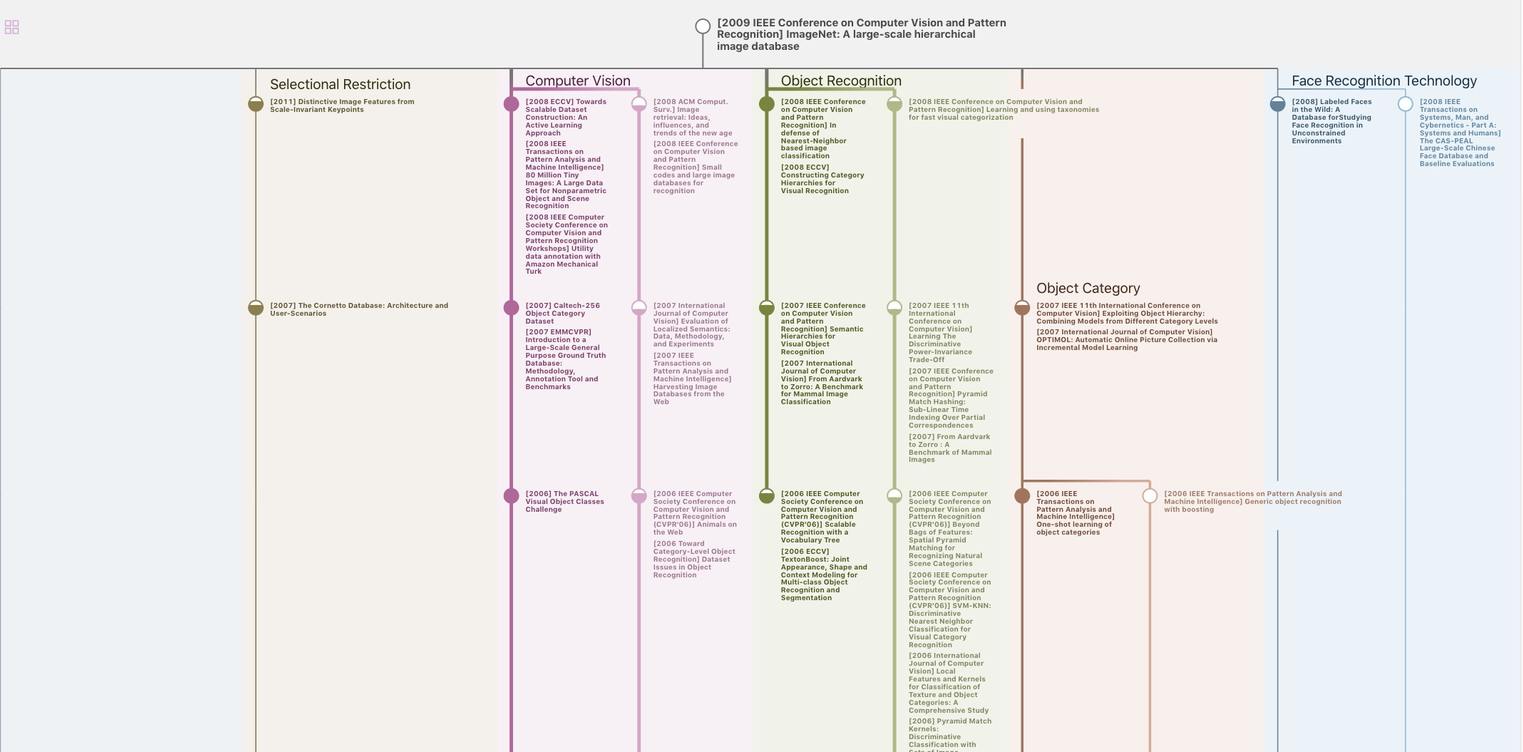
生成溯源树,研究论文发展脉络
Chat Paper
正在生成论文摘要