Supervised Latent Factor Analysis for Process Data Regression Modeling and Soft Sensor Application.
IEEE Trans. Contr. Sys. Techn.(2016)
摘要
This brief proposed a new supervised latent factor analysis (FA) method for process data regression modeling. Different from the traditional principal component analysis/regression model, the new model can successfully estimate heterogeneous variances from different process variables, which is more practical. Under the same probabilistic modeling framework, the single supervised latent FA model is further extended to the mixture form. Efficient expectation-maximization algorithms are developed for parameter learning in both single and mixture supervised latent FA models. Based on the regression modeling between easy-to-measure and difficult-to-measure process variables, two soft sensors are built for quality prediction in the process. Two case studies are provided to evaluate the modeling and performances of the new methods.
更多查看译文
关键词
Data models,Analytical models,Noise,Probabilistic logic,Noise measurement,Load modeling,Monitoring
AI 理解论文
溯源树
样例
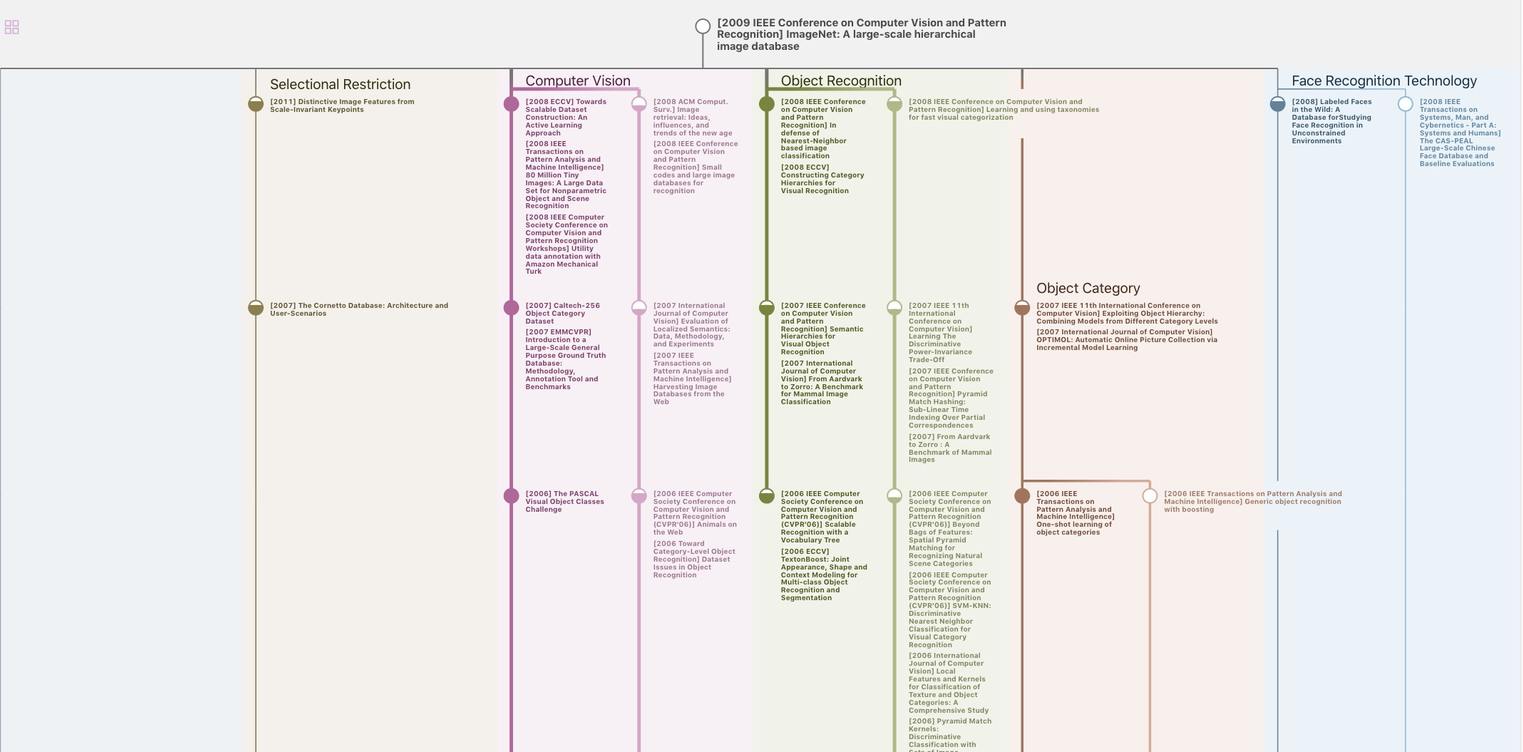
生成溯源树,研究论文发展脉络
Chat Paper
正在生成论文摘要