Hyperrectangles Selection for Monotonic Classification by Using Evolutionary Algorithms
INTERNATIONAL JOURNAL OF COMPUTATIONAL INTELLIGENCE SYSTEMS(2016)
摘要
In supervised learning, some real problems require the response attribute to represent ordinal values that should increase with some of the explaining attributes. They are called classification problems with monotonicity constraints. Hyperrectangles can be viewed as storing objects in ℝ n which can be used to learn concepts combining instance-based classification with the axis-parallel rectangle mainly used in rule induction systems. This hybrid paradigm is known as nested generalized exemplar learning. In this paper, we propose the selection of the most effective hyperrectangles by means of evolutionary algorithms to tackle monotonic classification. The model proposed is compared through an exhaustive experimental analysis involving a large number of data sets coming from real classification and regression problems. The results reported show that our evolutionary proposal outperforms other instance-based and rule learning models, such as OLM, OSDL, k -NN and MID; in accuracy and mean absolute error, requiring a fewer number of hyperrectangles.
更多查看译文
关键词
Monotonic Classification,Nested Generalized Examples,Evolutionary Algorithms,Rule Induction,Instance-based Learning
AI 理解论文
溯源树
样例
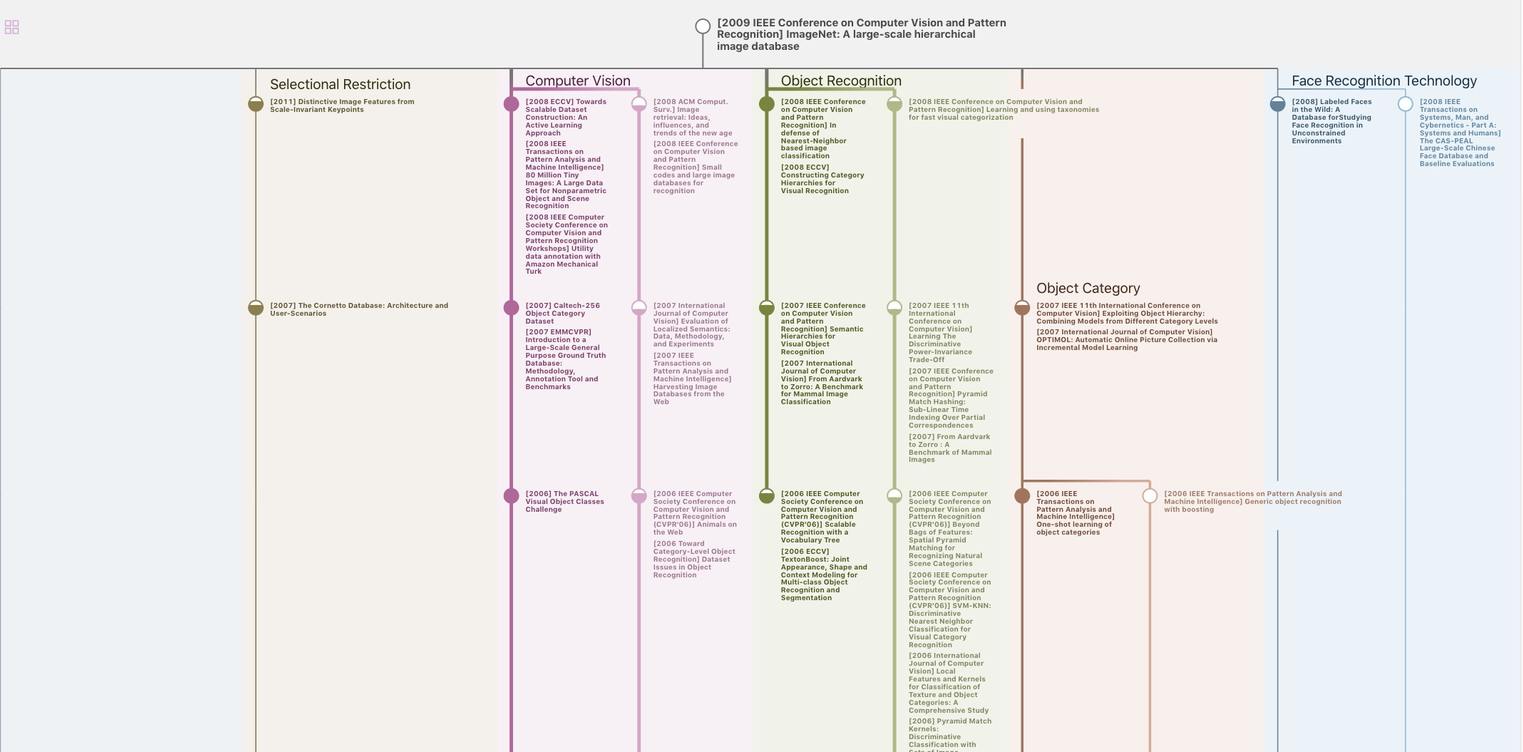
生成溯源树,研究论文发展脉络
Chat Paper
正在生成论文摘要