Parallel selective sampling method for imbalanced and large data classification
Pattern Recognition Letters(2015)
摘要
We proposed a new algorithm to preprocess huge and imbalanced data.This algorithm, based on distance calculations, reduce both size and imbalance.The selective sampling method was conceived for parallel and distributed computing.It was combined with SVM obtaining optimized classification performances.Synthetic and real data sets were used to evaluate the classifiers performances. Several applications aim to identify rare events from very large data sets. Classification algorithms may present great limitations on large data sets and show a performance degradation due to class imbalance. Many solutions have been presented in literature to deal with the problem of huge amount of data or imbalancing separately. In this paper we assessed the performances of a novel method, Parallel Selective Sampling (PSS), able to select data from the majority class to reduce imbalance in large data sets. PSS was combined with the Support Vector Machine (SVM) classification. PSS-SVM showed excellent performances on synthetic data sets, much better than SVM. Moreover, we showed that on real data sets PSS-SVM classifiers had performances slightly better than those of SVM and RUSBoost classifiers with reduced processing times. In fact, the proposed strategy was conceived and designed for parallel and distributed computing. In conclusion, PSS-SVM is a valuable alternative to SVM and RUSBoost for the problem of classification by huge and imbalanced data, due to its accurate statistical predictions and low computational complexity.
更多查看译文
关键词
Imbalanced learning,Classification,Support vector machine,Selective sampling methods
AI 理解论文
溯源树
样例
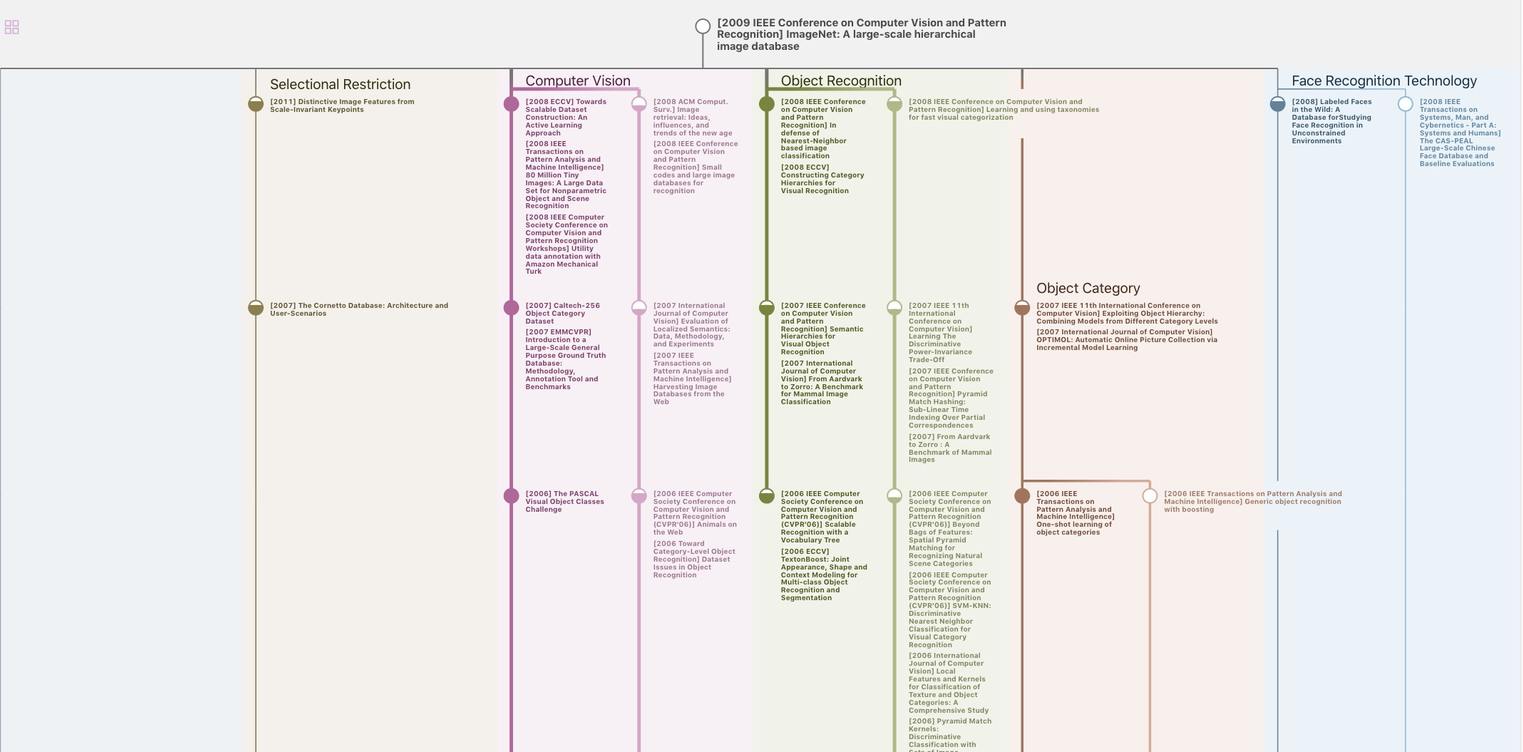
生成溯源树,研究论文发展脉络
Chat Paper
正在生成论文摘要