Enhancing Shift-Reduce Constituent Parsing with Action N-Gram Model.
ACM Trans. Asian & Low-Resource Lang. Inf. Process.(2016)
摘要
Current shift-reduce parsers “understand” the context by embodying a large number of binary indicator features with a discriminative model. In this article, we propose the action n-gram model, which utilizes the action sequence to help parsing disambiguation. The action n-gram model is trained on action sequences produced by parsers with the n-gram estimation method, which gives a smoothed maximum likelihood estimation of the action probability given a specific action history. We show that incorporating action n-gram models into a state-of-the-art parsing framework could achieve parsing accuracy improvements on three datasets across two languages.
更多查看译文
关键词
Languages,Experiments,Shift-reduce constituent parsing,action history,action n-gram model
AI 理解论文
溯源树
样例
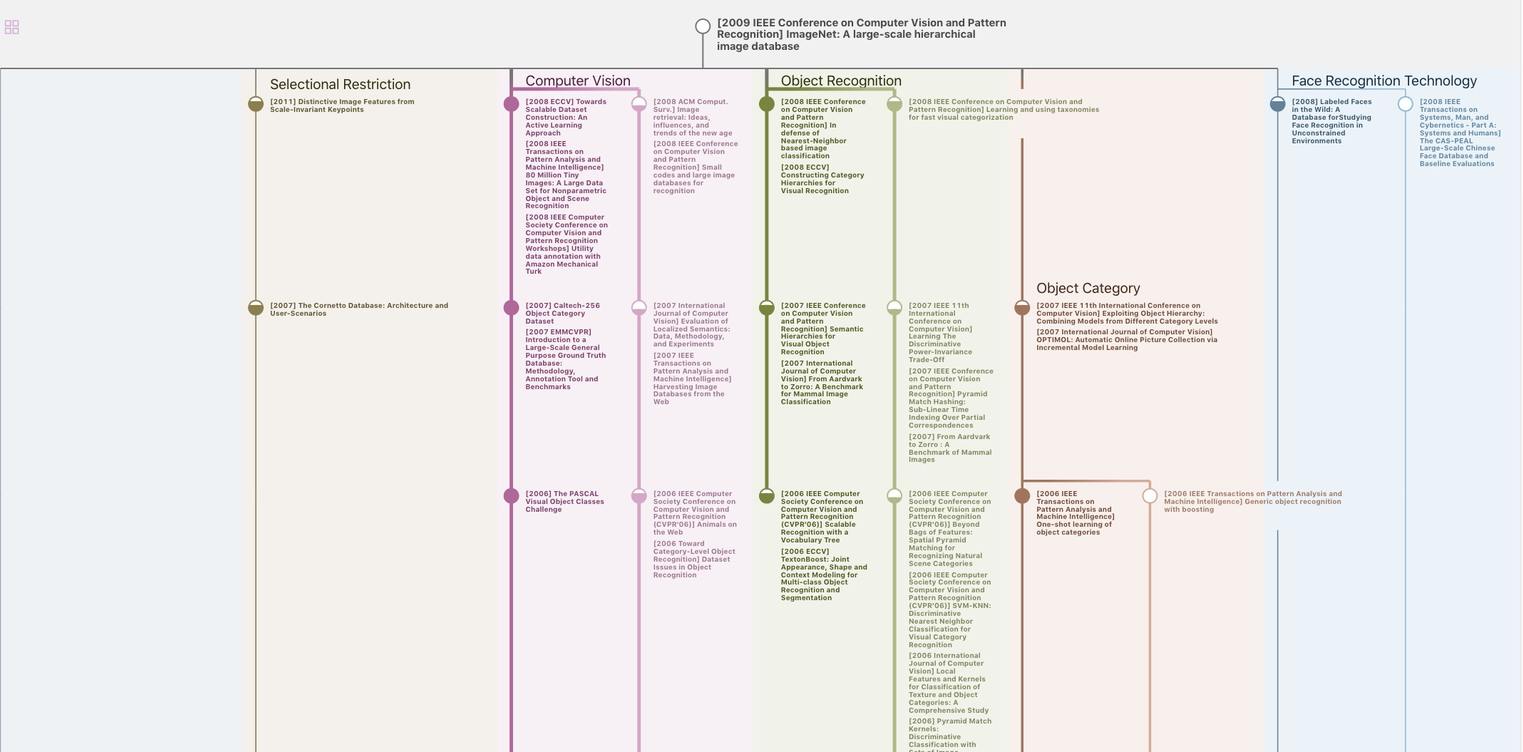
生成溯源树,研究论文发展脉络
Chat Paper
正在生成论文摘要