Multi-class LSTMSVM based on optimal directed acyclic graph and shuffled frog leaping algorithm
International Journal of Machine Learning and Cybernetics(2015)
摘要
Although TWSVM always achieves good performance for data classification, it does not take full advantage of the statistical information of the training data. Recently proposed twin mahalanobis distance-based support vector machine (TMSVM) modifies the standard TWSVM by constructing a pair of Mahalanobis distance-based kernels according to the covariance matrices of two classes of training data, which improves the generalization ability. However, TMSVW solves two dual quadratic programming problems. Moreover, it is proposed to deal with binary classification problems, while most of pattern recognition problems are problems of multi-class classification. In order to enhance the performance of TMSVM, in this paper, we formulate a fast least squares version of TMSVM which solves two modified primal problems instead of two dual problems. The solution of two modified primal problems can easily be obtained by solving a set of linear equations in the primal space. Then we propose a new multiclass classification algorithm, named DAG-LSTMSVM for multi-class classification, by combining least squares TMSVM and directed acyclic graph (DAG). A mahalanobis distance-based distance measure is designed as the class separability criterion to construct the optimal DAG structure. A modified shuffled frog leaping algorithm-based model selection for DAG-LSTMSVM is suggested for parameter selection. The experimental results on artificial dataset and UCI datasets show that the proposed algorithm obtains high classification accuracy and good generalization ability.
更多查看译文
关键词
Twin support vector machine,Multi-class classification,Mahalanobis distance,Directed acyclic graph,Shuffled frog leaping algorithm
AI 理解论文
溯源树
样例
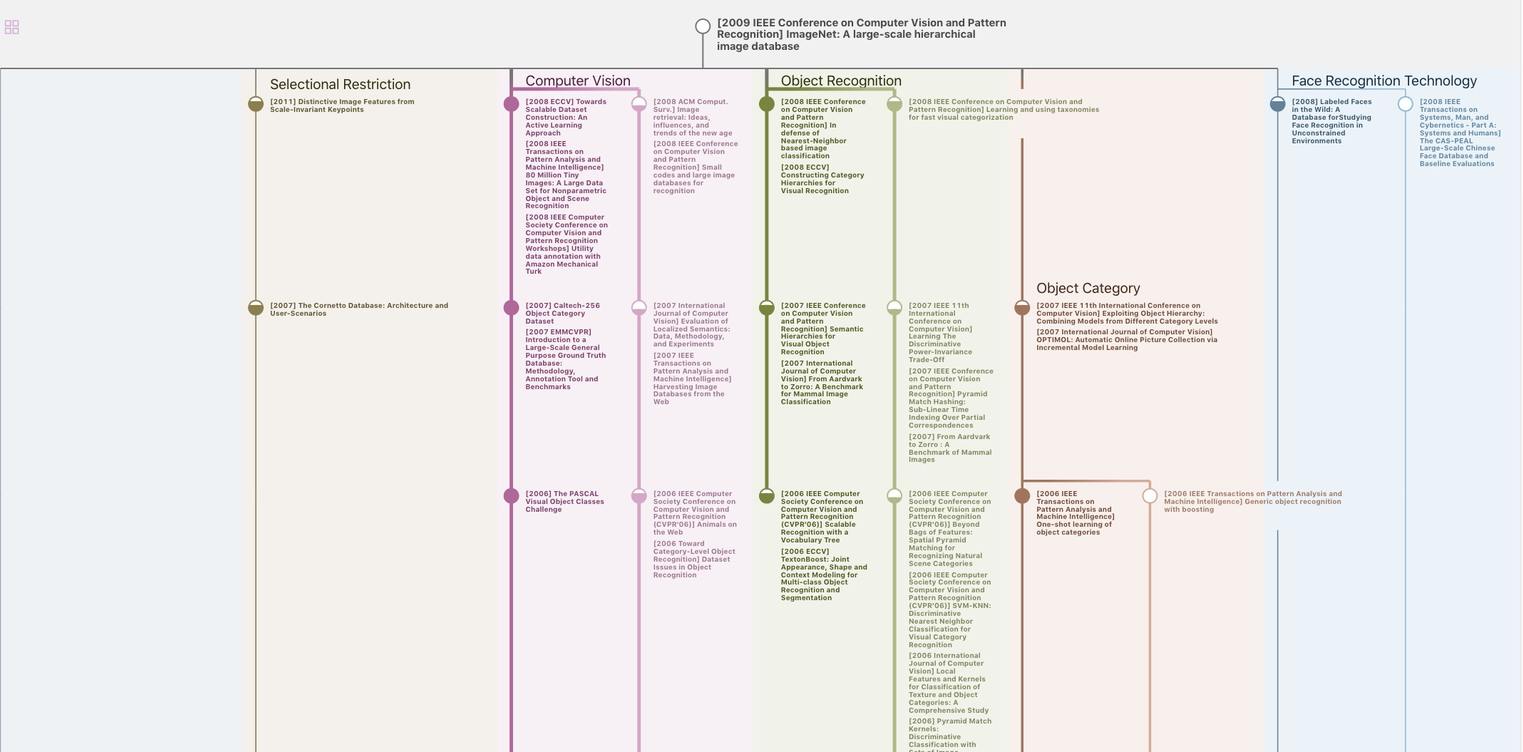
生成溯源树,研究论文发展脉络
Chat Paper
正在生成论文摘要