Dynamic community detection in evolving networks using locality modularity optimization
Social Netw. Analys. Mining(2016)
摘要
The amount and the variety of data generated by today’s online social and telecommunication network services are changing the way researchers analyze social networks. Facing fast evolving networks with millions of nodes and edges are, among other factors, its main challenge. Community detection algorithms in these conditions have also to be updated or improved. Previous state-of-the-art algorithms based on the modularity optimization (i.e. Louvain algorithm), provide fast, efficient and robust community detection on large static networks. Nonetheless, due to the high computing complexity of these algorithms, the use of batch techniques in dynamic networks requires to perform network community detection for the whole network in each one of the evolution steps. This fact reveals to be computationally expensive and unstable in terms of tracking of communities. Our contribution is a novel technique that maintains the community structure always up-to-date following the addition or removal of nodes and edges. The proposed algorithm performs a local modularity optimization that maximizes the modularity gain function only for those communities where the editing of nodes and edges was performed, keeping the rest of the network unchanged. The effectiveness of our algorithm is demonstrated with the comparison to other state-of-the-art community detection algorithms with respect to Newman’s Modularity, Modularity with Split Penalty, Modularity Density, number of detected communities and running time.
更多查看译文
关键词
Dynamic community detection, Large-scale networks analysis, Modularity, Evolving networks
AI 理解论文
溯源树
样例
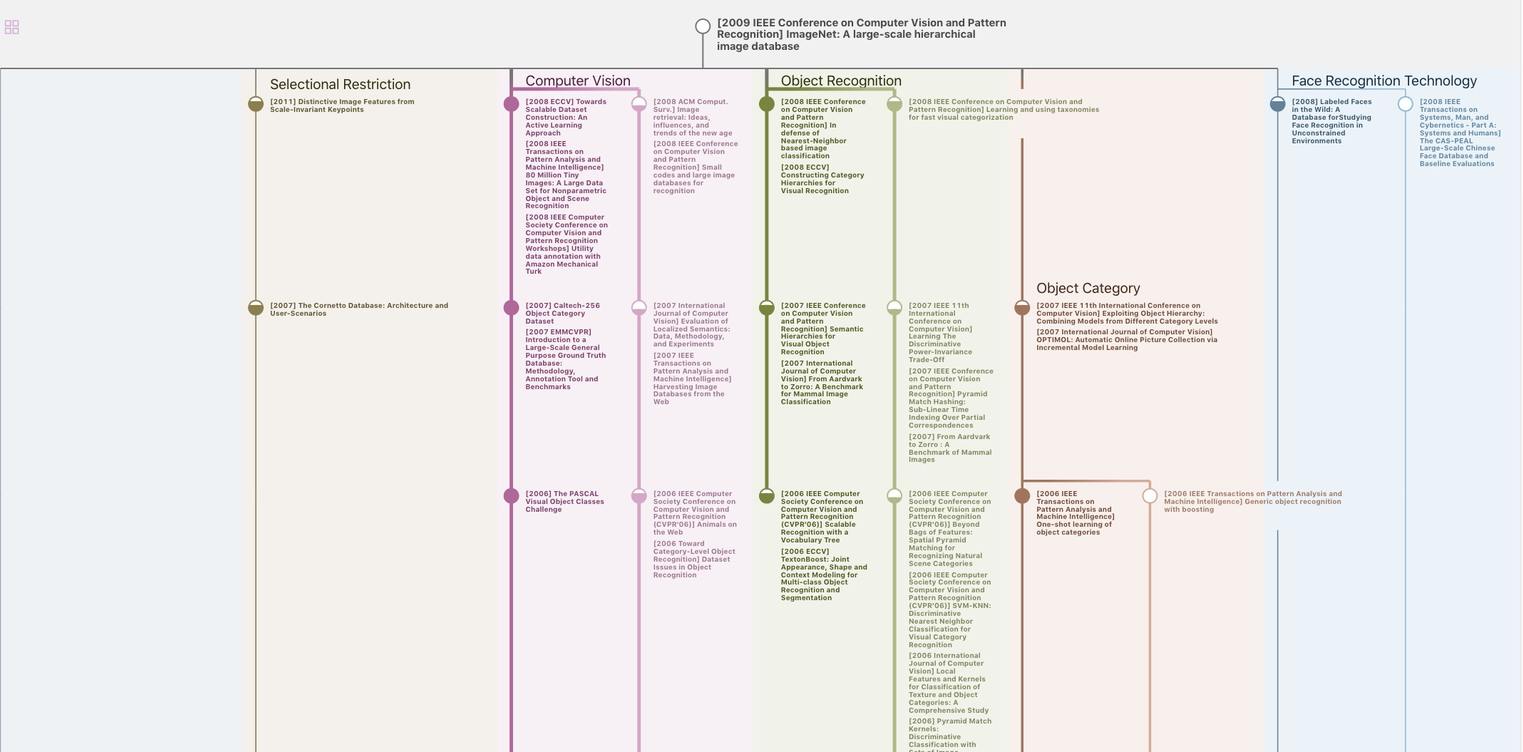
生成溯源树,研究论文发展脉络
Chat Paper
正在生成论文摘要