Discovering Attribute Shades of Meaning with the Crowd
International Journal of Computer Vision(2015)
摘要
To learn semantic attributes, existing methods typically train one discriminative model for each word in a vocabulary of nameable properties. However, this “one model per word” assumption is problematic: while a word might have a precise linguistic definition, it need not have a precise visual definition. We propose to discover shades of attribute meaning. Given an attribute name, we use crowdsourced image labels to discover the latent factors underlying how different annotators perceive the named concept. We show that structure in those latent factors helps reveal shades, that is, interpretations for the attribute shared by some group of annotators. Using these shades, we train classifiers to capture the primary (often subtle) variants of the attribute. The resulting models are both semantic and visually precise. By catering to users’ interpretations, they improve attribute prediction accuracy on novel images. Shades also enable more successful attribute-based image search, by providing robust personalized models for retrieving multi-attribute query results. They are widely applicable to tasks that involve describing visual content, such as zero-shot category learning and organization of photo collections.
更多查看译文
关键词
Attribute learning and perception,Vision and language,Attribute discovery
AI 理解论文
溯源树
样例
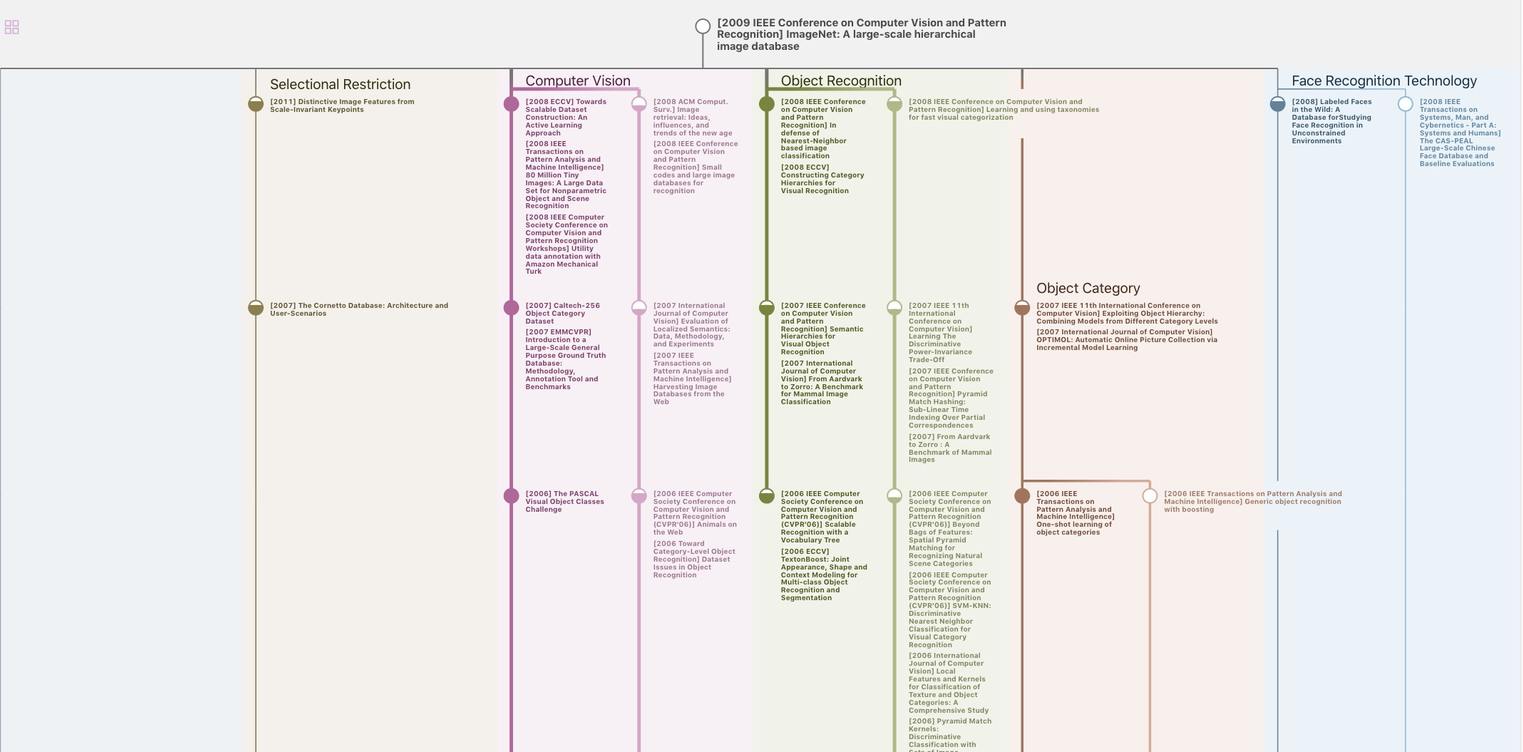
生成溯源树,研究论文发展脉络
Chat Paper
正在生成论文摘要