An Algorithm For The Segmentation Of Highly Abnormal Hearts Using A Generic Statistical Shape Model
IEEE transactions on medical imaging(2016)
摘要
Statistical shape models (SSMs) have been widely employed in cardiac image segmentation. However, in conditions that induce severe shape abnormality and remodeling, such as in the case of pulmonary hypertension (PH) or hypertrophic cardiomyopathy (HCM), a single SSM is rarely capable of capturing the anatomical variability in the extremes of the distribution. This work presents a new algorithm for the segmentation of severely abnormal hearts. The algorithm is highly flexible, as it does not require a priori knowledge of the involved pathology or any specific parameter tuning to be applied to the cardiac image under analysis. The fundamental idea is to approximate the gross effect of the abnormality with a virtual remodeling transformation between the patient-specific geometry and the average shape of the reference model (e.g., average normal morphology). To define this mapping, a set of landmark points are automatically identified during boundary point search, by estimating the reliability of the candidate points. With the obtained transformation, the feature points extracted from the patient image volume are then projected onto the space of the reference SSM, where the model is used to effectively constrain and guide the segmentation process. The extracted shape in the reference space is finally propagated back to the original image of the abnormal heart to obtain the final segmentation. Detailed validation with patients diagnosed with PH and HCM shows the robustness and flexibility of the technique for the segmentation of highly abnormal hearts of different pathologies.
更多查看译文
关键词
Abnormal hearts,cardiac segmentation,hypertrophic cardiomyopathy,magnetic resonance imaging,pulmonary hypertension,statistical shape models
AI 理解论文
溯源树
样例
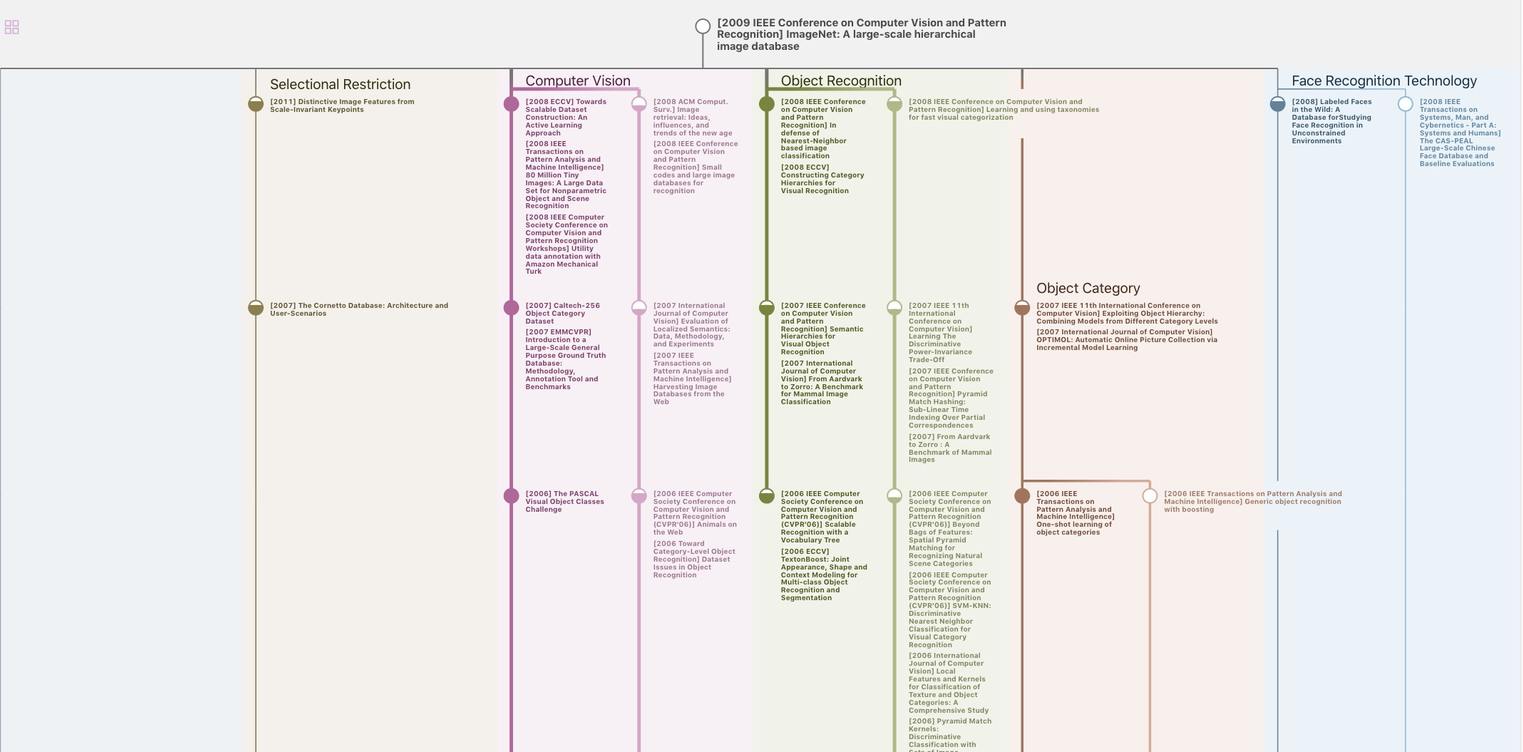
生成溯源树,研究论文发展脉络
Chat Paper
正在生成论文摘要