Deep Active Object Recognition by Joint Label and Action Prediction
Computer Vision and Image Understanding(2017)
摘要
A deep convolutional neural network is used for active object recognition.The network outputs object label and the action to be performed on the object.Cost function includes reinforcement learning and label prediction costs.A generative Dirichlet model is embedded in the network for state encoding.Training is done by tuning the visual features for label and action prediction. An active object recognition system has the advantage of acting in the environment to capture images that are more suited for training and lead to better performance at test time. In this paper, we utilize deep convolutional neural networks for active object recognition by simultaneously predicting the object label and the next action to be performed on the object with the aim of improving recognition performance. We treat active object recognition as a reinforcement learning problem and derive the cost function to train the network for joint prediction of the object label and the action. A generative model of object similarities based on the Dirichlet distribution is proposed and embedded in the network for encoding the state of the system. The training is carried out by simultaneously minimizing the label and action prediction errors using gradient descent. We empirically show that the proposed network is able to predict both the object label and the actions on GERMS, a dataset for active object recognition. We compare the test label prediction accuracy of the proposed model with Dirichlet and Naive Bayes state encoding. The results of experiments suggest that the proposed model equipped with Dirichlet state encoding is superior in performance, and selects images that lead to better training and higher accuracy of label prediction at test time.
更多查看译文
关键词
Active object recognition,Deep learning,Q-learning
AI 理解论文
溯源树
样例
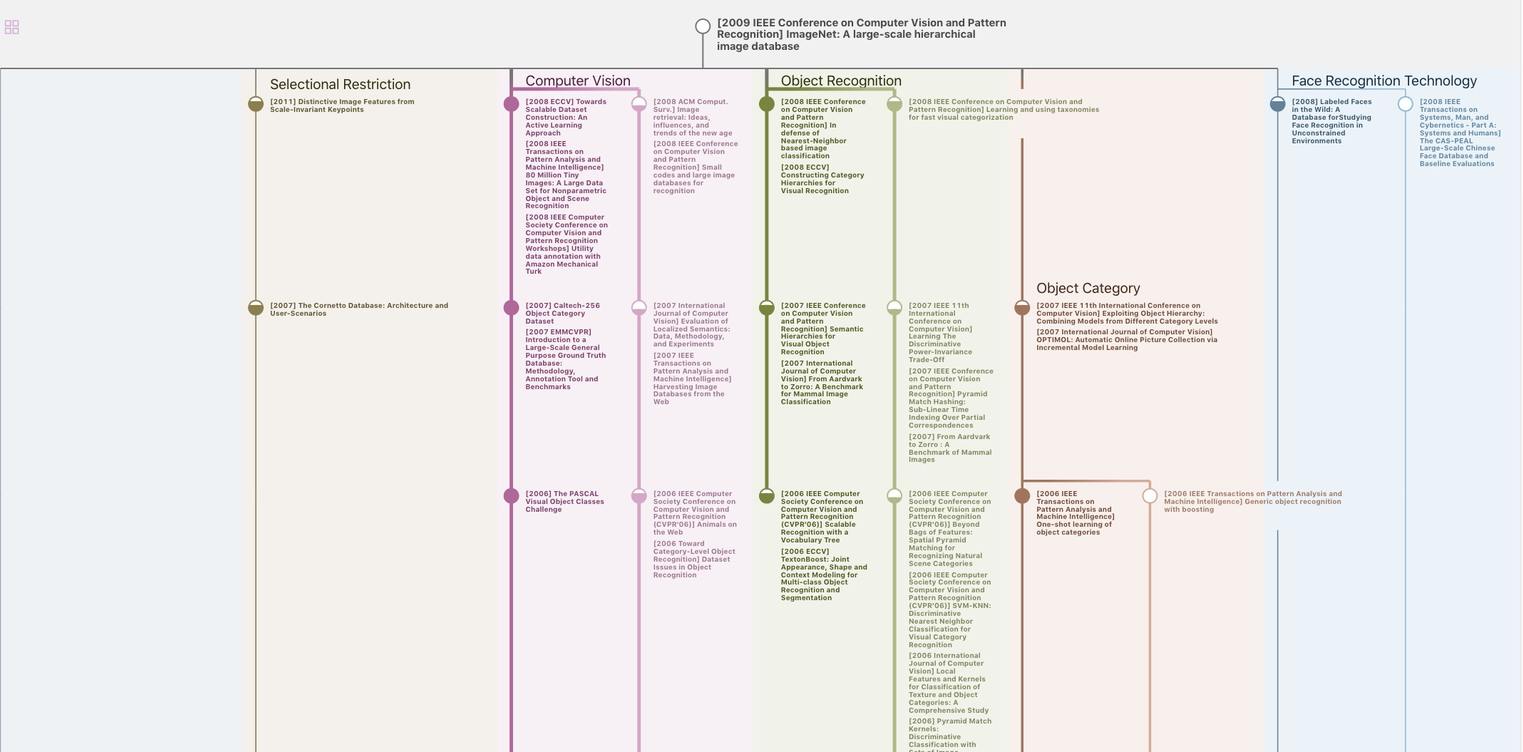
生成溯源树,研究论文发展脉络
Chat Paper
正在生成论文摘要