Predictability of Popularity: Gaps between Prediction and Understanding.
ICWSM(2016)
摘要
Can we predict the future popularity of a song, movie or tweet? Recent work suggests that although it may be hard to predict an itemu0027s popularity when it is first introduced, peeking into its early adopters and properties of their social network makes the problem easier. We test the robustness of such claims by using data from social networks spanning music, books, photos, and URLs. We find a stronger result: not only do predictive models with peeking achieve high accuracy on all datasets, they also generalize well, so much so that models trained on any one dataset perform with comparable accuracy on items from other datasets. Though practically useful, our models (and those in other work) are intellectually unsatisfying because common formulations of the problem, which involve peeking at the first small-k adopters and predicting whether items end up in the top half of popular items, are both too sensitive to the speed of early adoption and too easy. Most of the predictive power comes from looking at how quickly items reach their first few adopters, while for other features of early adopters and their networks, even the direction of correlation with popularity is not consistent across domains. Problem formulations that examine items that reach k adopters in about the same amount of time reduce the importance of temporal features, but also overall accuracy, highlighting that we understand little about why items become popular while providing a context in which we might build that understanding.
更多查看译文
关键词
popularity,prediction
AI 理解论文
溯源树
样例
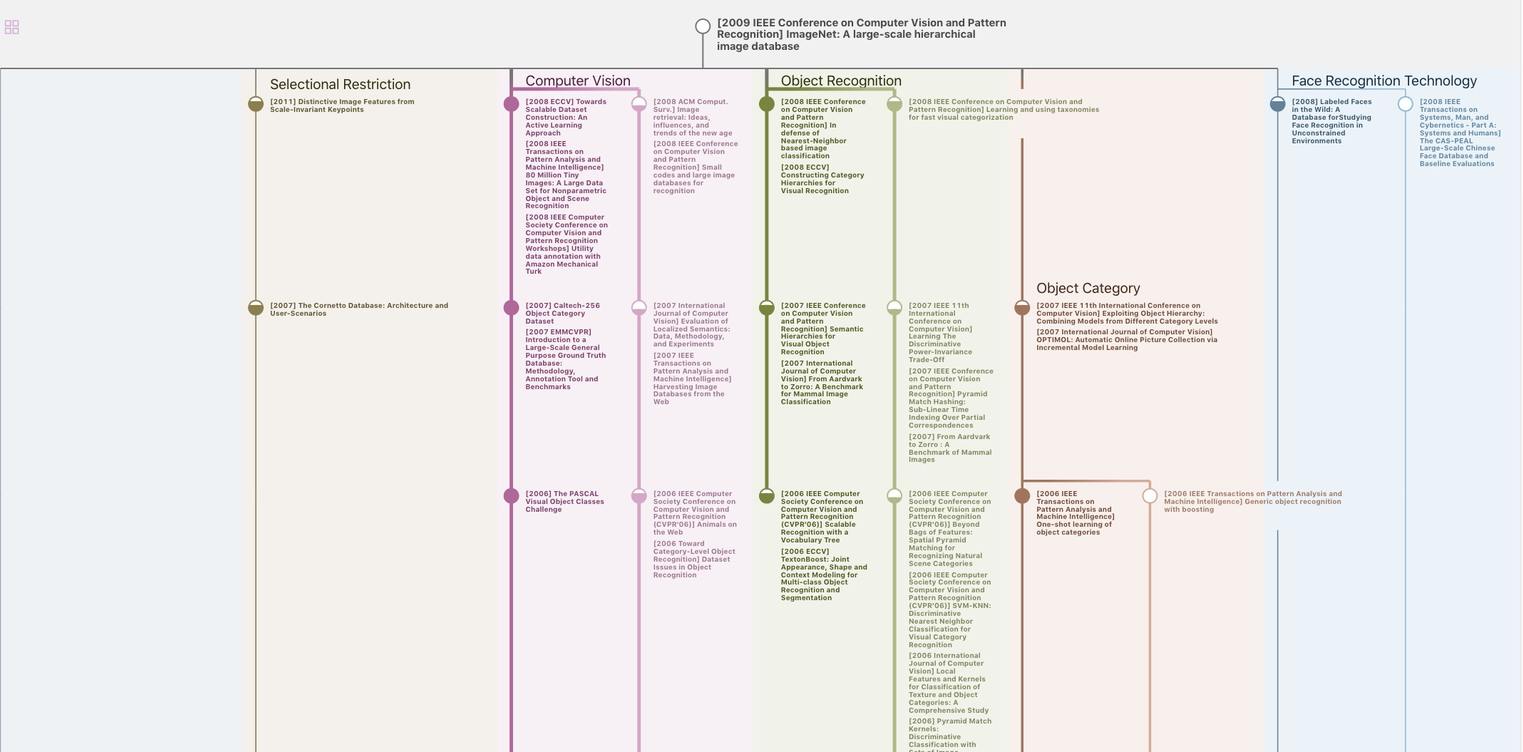
生成溯源树,研究论文发展脉络
Chat Paper
正在生成论文摘要