Iterative Refinement of Approximate Posterior for Training Directed Belief Networks
CoRR(2015)
摘要
Recent advances in variational inference that make use of an inference or recognition network for training and evaluating deep directed graphical models have advanced well beyond traditional variational inference and Markov chain Monte Carlo methods. These techniques offer higher flexibility with simpler and faster inference; yet training and evaluation still remains a challenge. We propose a method for improving the per-example approximate posterior by iterative refinement, which can provide notable gains in maximizing the variational lower bound of the log likelihood and works with both continuous and discrete latent variables. We evaluate our approach as a method of training and evaluating directed graphical models. We show that, when used for training, iterative refinement improves the variational lower bound and can also improve the log-likelihood over related methods. We also show that iterative refinement can be used to get a better estimate of the log-likelihood in any directed model trained with mean-field inference.
更多查看译文
AI 理解论文
溯源树
样例
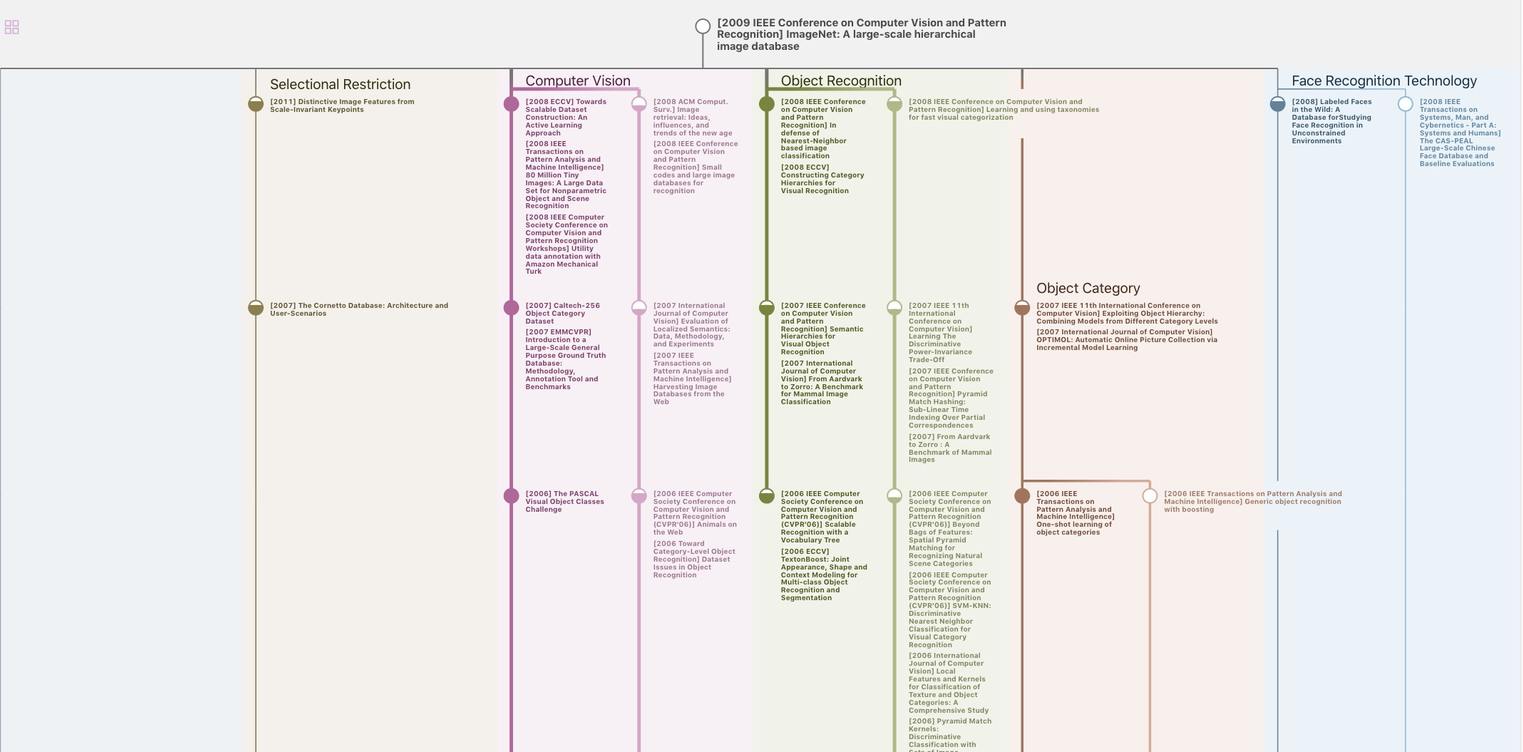
生成溯源树,研究论文发展脉络
Chat Paper
正在生成论文摘要