Towards Adapting Deep Visuomotor Representations from Simulated to Real Environments
CoRR(2015)
摘要
We address the problem of adapting robotic perception from simulated to real-world environments. For many robotic control tasks, real training imagery is expensive to obtain, but a large number of synthetic images is easy to generate through simulation. We propose a method that adapts visual representations using a small number of paired synthetic and real views of the same scene. Our model generalizes prior approaches and combines a standard in-domain loss, a cross-domain adaptation loss, and a contrastive loss explicitly designed to align pairs of images in feature space. We presume a synthetic dataset comprised of views that are a superset of a small number of real views, where the alignment may be either explicit or latent. We evaluate our approach on a manipulation task and show that by exploiting the presence of synthetic-real image pairs, our model is able to compensate for domain shift more effectively than conventional initialization techniques. Our results serve as an initial step toward pretraining deep visuomotor policies entirely in simulation, significantly reducing physical demands when learning complex policies.
更多查看译文
AI 理解论文
溯源树
样例
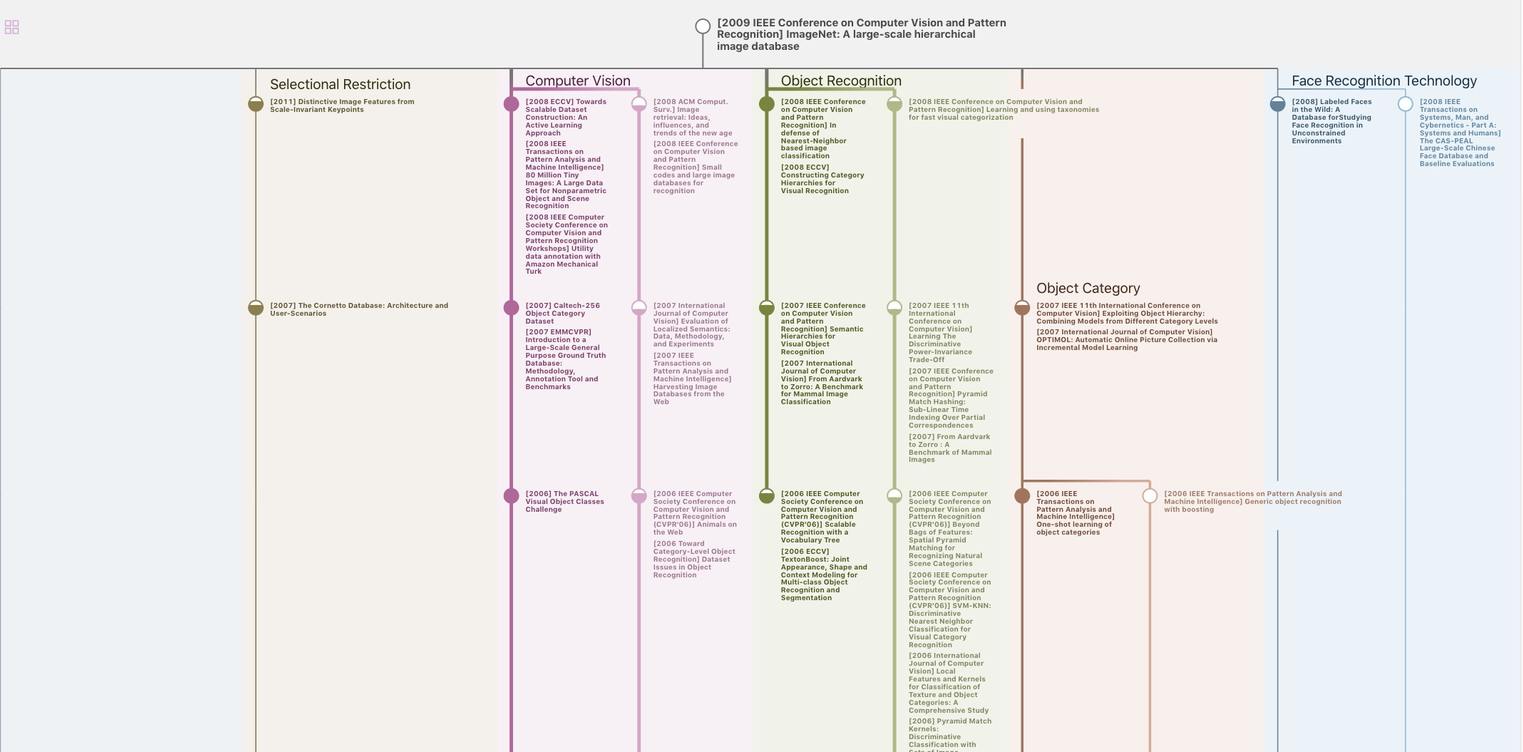
生成溯源树,研究论文发展脉络
Chat Paper
正在生成论文摘要