A deep matrix factorization method for learning attribute representations
IEEE transactions on pattern analysis and machine intelligence(2017)
摘要
Semi-Non-negative Matrix Factorization is a technique that learns a low-dimensional representation of a dataset that lends itself to a clustering interpretation. It is possible that the mapping between this new representation and our original data matrix contains rather complex hierarchical information with implicit lower-level hidden attributes, that classical one level clustering methodologies cannot interpret. In this work we propose a novel model, Deep Semi-NMF, that is able to learn such hidden representations that allow themselves to an interpretation of clustering according to different, unknown attributes of a given dataset. We also present a semi-supervised version of the algorithm, named Deep WSF, that allows the use of (partial) prior information for each of the known attributes of a dataset, that allows the model to be used on datasets with mixed attribute knowledge. Finally, we show that our models are able to learn low-dimensional representations that are better suited for clustering, but also classification, outperforming Semi-Non-negative Matrix Factorization, but also other state-of-the-art methodologies variants.
更多查看译文
关键词
Face,Clustering algorithms,Matrix decomposition,Data models,Algorithm design and analysis,Feature extraction,Face recognition
AI 理解论文
溯源树
样例
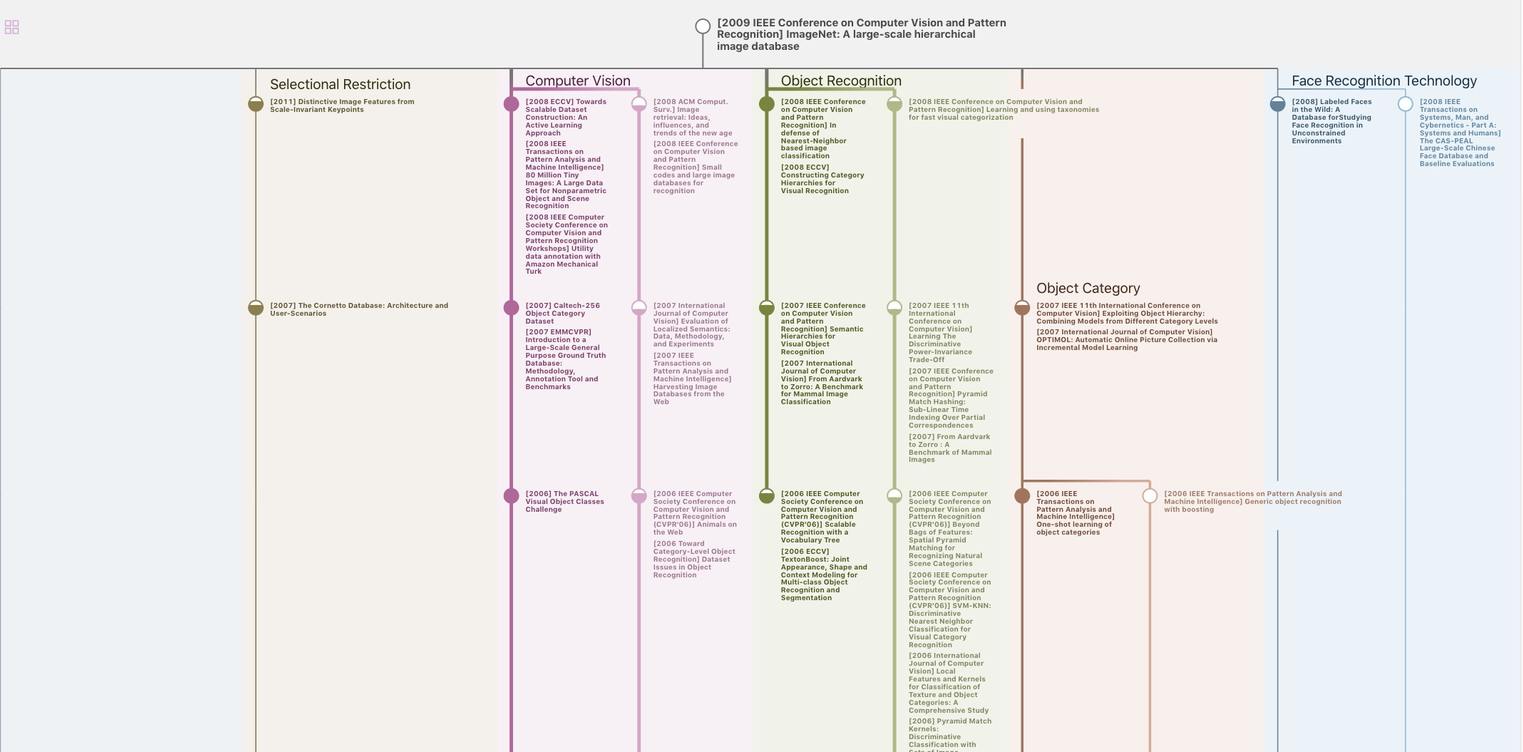
生成溯源树,研究论文发展脉络
Chat Paper
正在生成论文摘要