Accelerated Stochastic Gradient Descent for Minimizing Finite Sums
JMLR Workshop and Conference Proceedings(2016)
摘要
We propose an optimization method for minimizing the finite sums of smooth convex functions. Our method incorporates an accelerated gradient descent (AGD) and a stochastic variance reduction gradient (SVRG) in a mini-batch setting. An important feature of the method is that it can be directly applied to general convex and optimal strongly convex problems that is a weaker condition than strong convexity. We show that our method achieves a better overall complexity for the general convex problems and linear convergence for optimal strongly convex problems. Moreover we prove the fast iteration complexity of our method. Our experiments show the effectiveness of our method.
更多查看译文
AI 理解论文
溯源树
样例
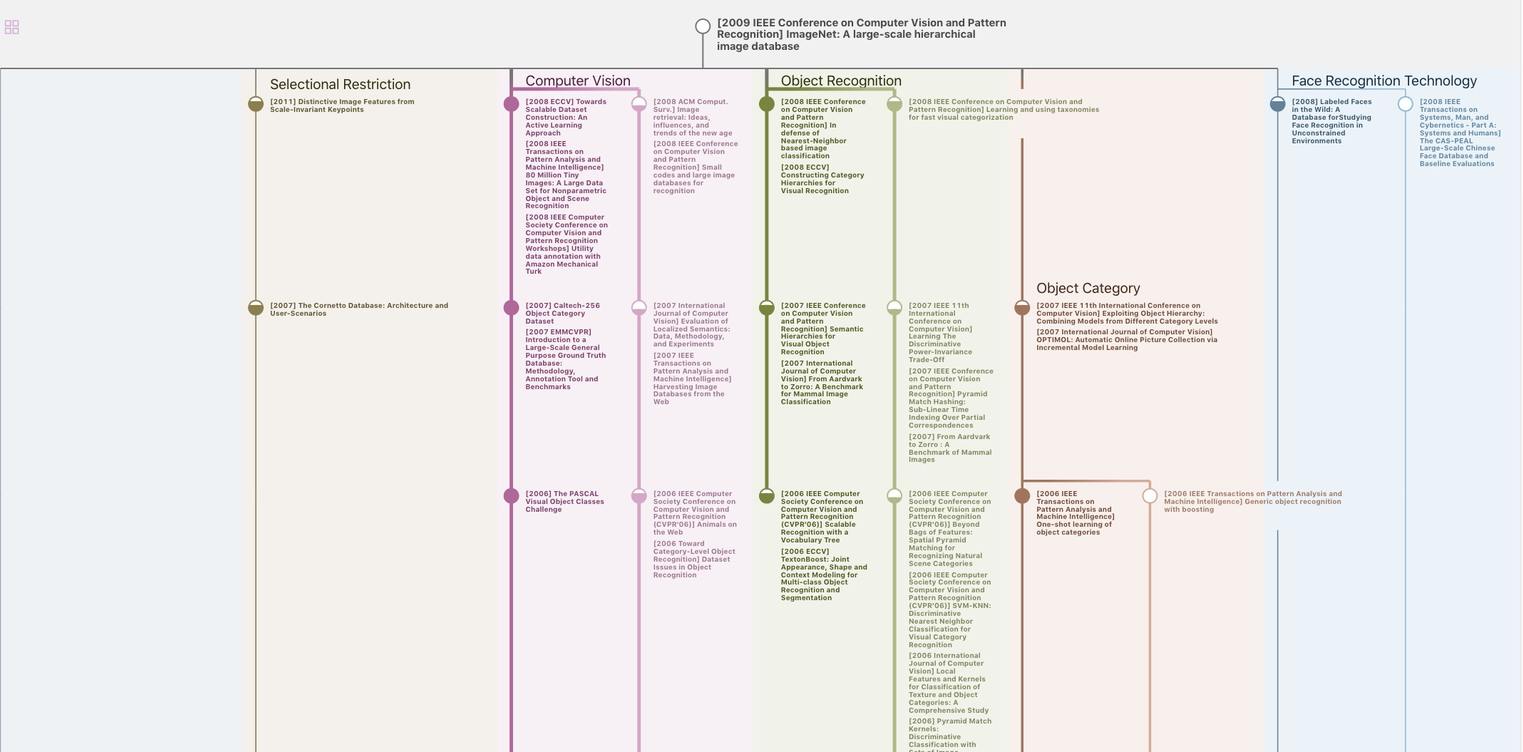
生成溯源树,研究论文发展脉络
Chat Paper
正在生成论文摘要