Segmental Recurrent Neural Networks for End-to-end Speech Recognition
17TH ANNUAL CONFERENCE OF THE INTERNATIONAL SPEECH COMMUNICATION ASSOCIATION (INTERSPEECH 2016), VOLS 1-5: UNDERSTANDING SPEECH PROCESSING IN HUMANS AND MACHINES(2016)
摘要
We study the segmental recurrent neural network for end-to-end acoustic modelling. This model connects the segmental conditional random field (CRF) with a recurrent neural network (RNN) used for feature extraction. Compared to most previous CRF-based acoustic models, it does not rely on an external system to provide features or segmentation boundaries. Instead, this model marginalises out all the possible segmentations, and features are extracted from the RNN trained together with the segmental CRF. In essence, this model is self-contained and can be trained end-to-end. In this paper, we discuss practical training and decoding issues as well as the method to speed up the training in the context of speech recognition. We performed experiments on the TIMIT dataset. We achieved 17.3 phone error rate (PER) from the first-pass decoding --- the best reported result using CRFs, despite the fact that we only used a zeroth-order CRF and without using any language model.
更多查看译文
关键词
end-to-end speech recognition, segmental CRF, recurrent neural networks
AI 理解论文
溯源树
样例
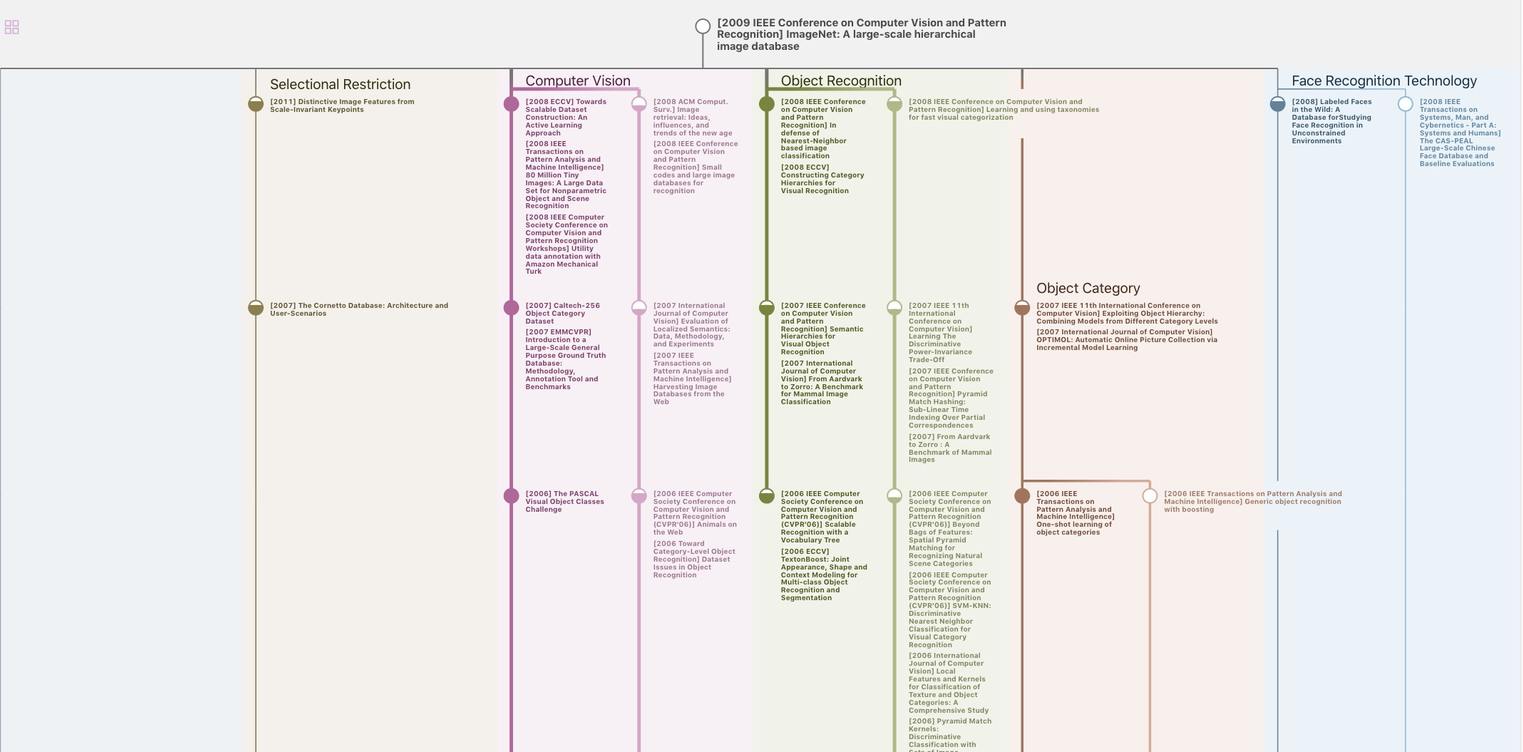
生成溯源树,研究论文发展脉络
Chat Paper
正在生成论文摘要