Extreme Extraction: Only One Hour per Relation
CoRR(2015)
摘要
Information Extraction (IE) aims to automatically generate a large knowledge base from natural language text, but progress remains slow. Supervised learning requires copious human annotation, while unsupervised and weakly supervised approaches do not deliver competitive accuracy. As a result, most fielded applications of IE, as well as the leading TAC-KBP systems, rely on significant amounts of manual engineering. Even "Extreme" methods, such as those reported in Freedman et al. 2011, require about 10 hours of expert labor per relation. This paper shows how to reduce that effort by an order of magnitude. We present a novel system, InstaRead, that streamlines authoring with an ensemble of methods: 1) encoding extraction rules in an expressive and compositional representation, 2) guiding the user to promising rules based on corpus statistics and mined resources, and 3) introducing a new interactive development cycle that provides immediate feedback --- even on large datasets. Experiments show that experts can create quality extractors in under an hour and even NLP novices can author good extractors. These extractors equal or outperform ones obtained by comparably supervised and state-of-the-art distantly supervised approaches.
更多查看译文
AI 理解论文
溯源树
样例
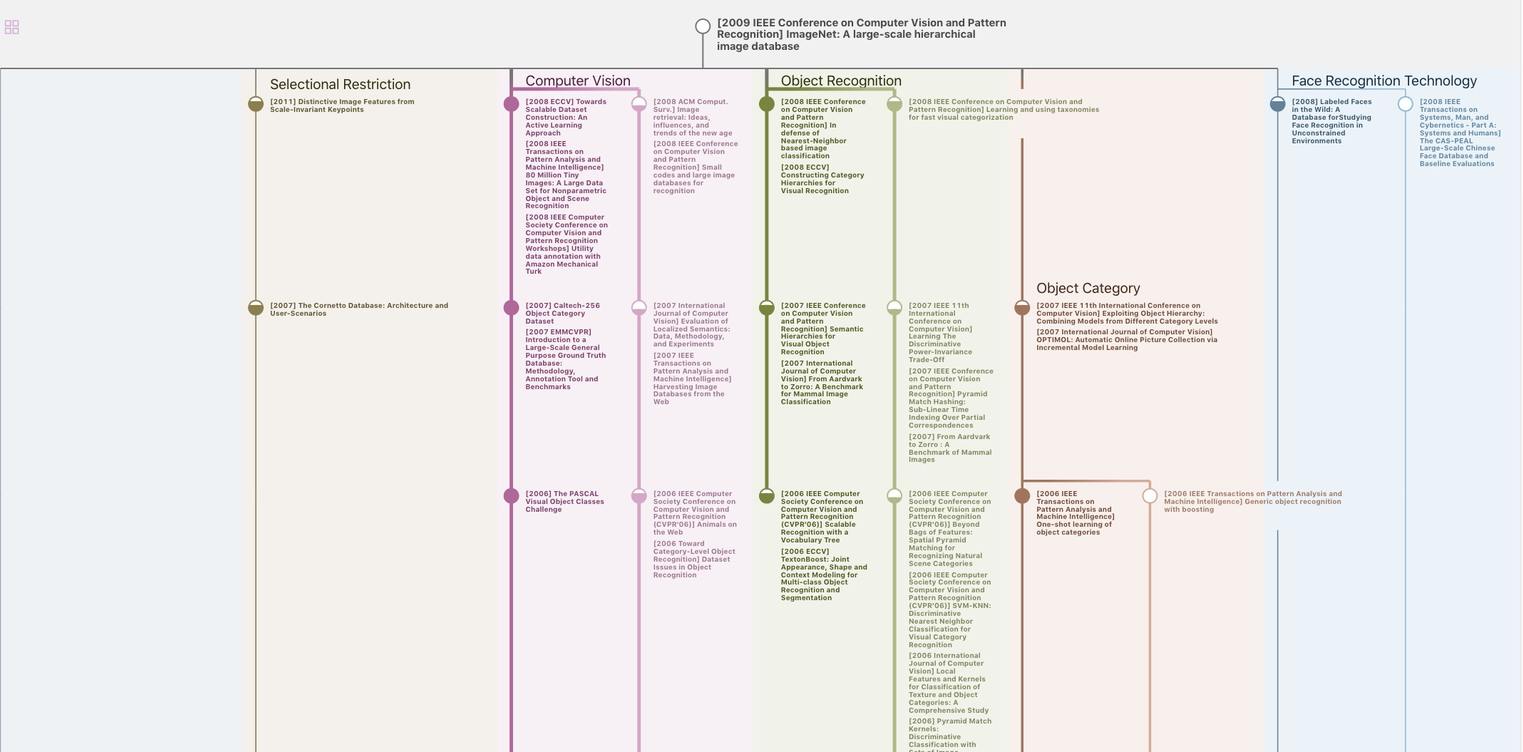
生成溯源树,研究论文发展脉络
Chat Paper
正在生成论文摘要