Peak criterion for choosing Gaussian kernel bandwidth in Support Vector Data Description
2017 IEEE International Conference on Prognostics and Health Management (ICPHM)(2017)
摘要
Support Vector Data Description (SVDD) is a machine learning technique used for single class classification and outlier detection. SVDD formulation with kernel function provides a flexible boundary around data. The value of kernel function parameters affects the nature of data boundary. For example, it is observed that with Gaussian kernel, as the value of kernel bandwidth is lowered, the data boundary changes from spherical to wiggly. The spherical data boundary leads to underfitting and extremely wiggly data boundary leads to overfitting. In this paper we propose an empirical criteria to obtain a good value of Gaussian kernel bandwidth which provides a smooth boundary capturing the essential visual features of the data.
更多查看译文
关键词
Gaussian kernel bandwidth,support vector data description,machine learning,single class classification,outlier detection,SVDD formulation,flexible boundary,kernel function parameters,spherical data boundary,visual features,smooth boundary
AI 理解论文
溯源树
样例
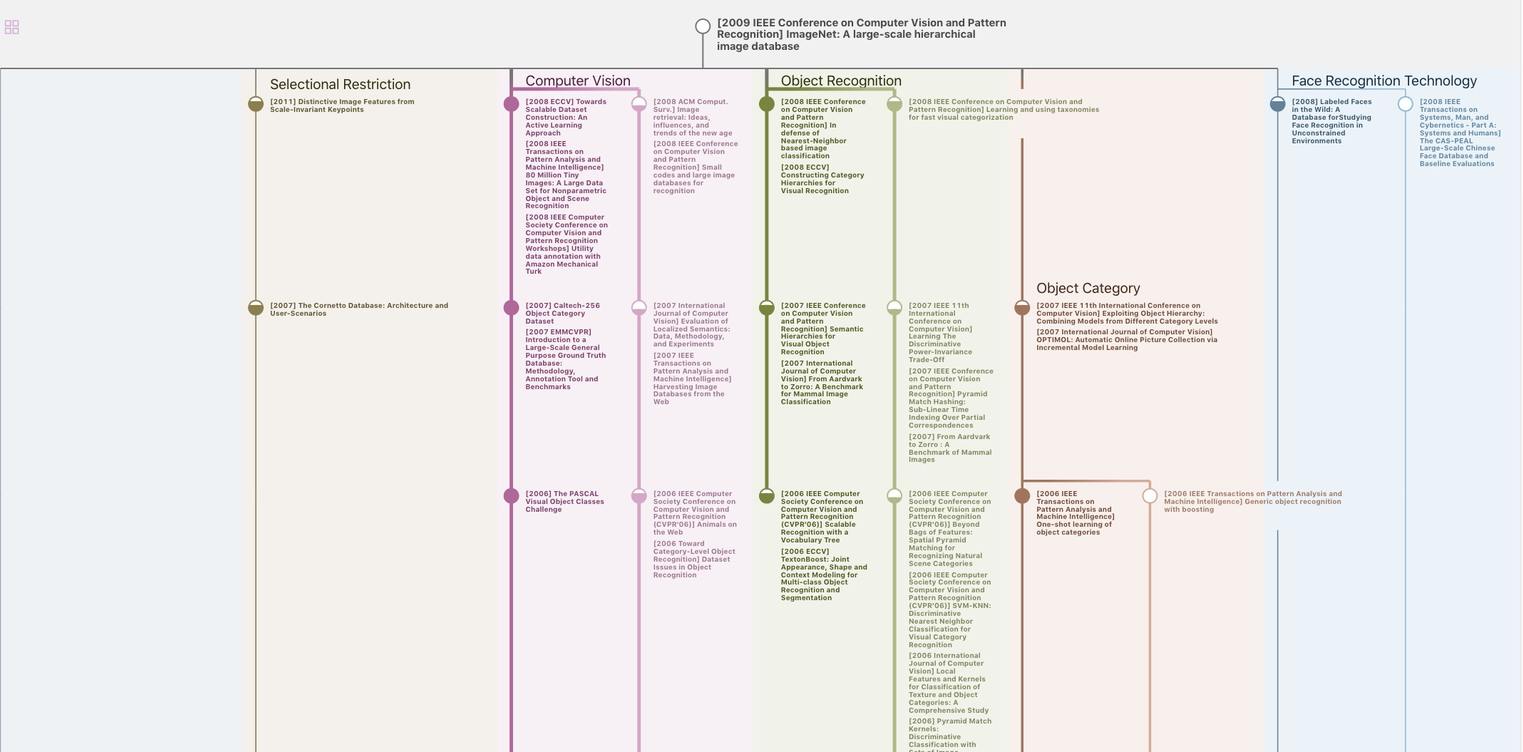
生成溯源树,研究论文发展脉络
Chat Paper
正在生成论文摘要