Minimum Conditional Description Length Estimation for Markov Random Fields
Deep Blue (University of Michigan)(2016)
摘要
In this paper we discuss a method, which we call Minimum Conditional Description Length (MCDL), for estimating the parameters of a subset of sites within a Markov random field. We assume that the edges are known for the entire graph $G=(V,E)$. Then, for a subset $U\subset V$, we estimate the parameters for nodes and edges in $U$ as well as for edges incident to a node in $U$, by finding the exponential parameter for that subset that yields the best compression conditioned on the values on the boundary $\partial U$. Our estimate is derived from a temporally stationary sequence of observations on the set $U$. We discuss how this method can also be applied to estimate a spatially invariant parameter from a single configuration, and in so doing, derive the Maximum Pseudo-Likelihood (MPL) estimate.
更多查看译文
关键词
minimum conditional description length estimation,Markov random fields,MCDL,parameter estimation,graph,maximum pseudo-likelihood,MPL estimate
AI 理解论文
溯源树
样例
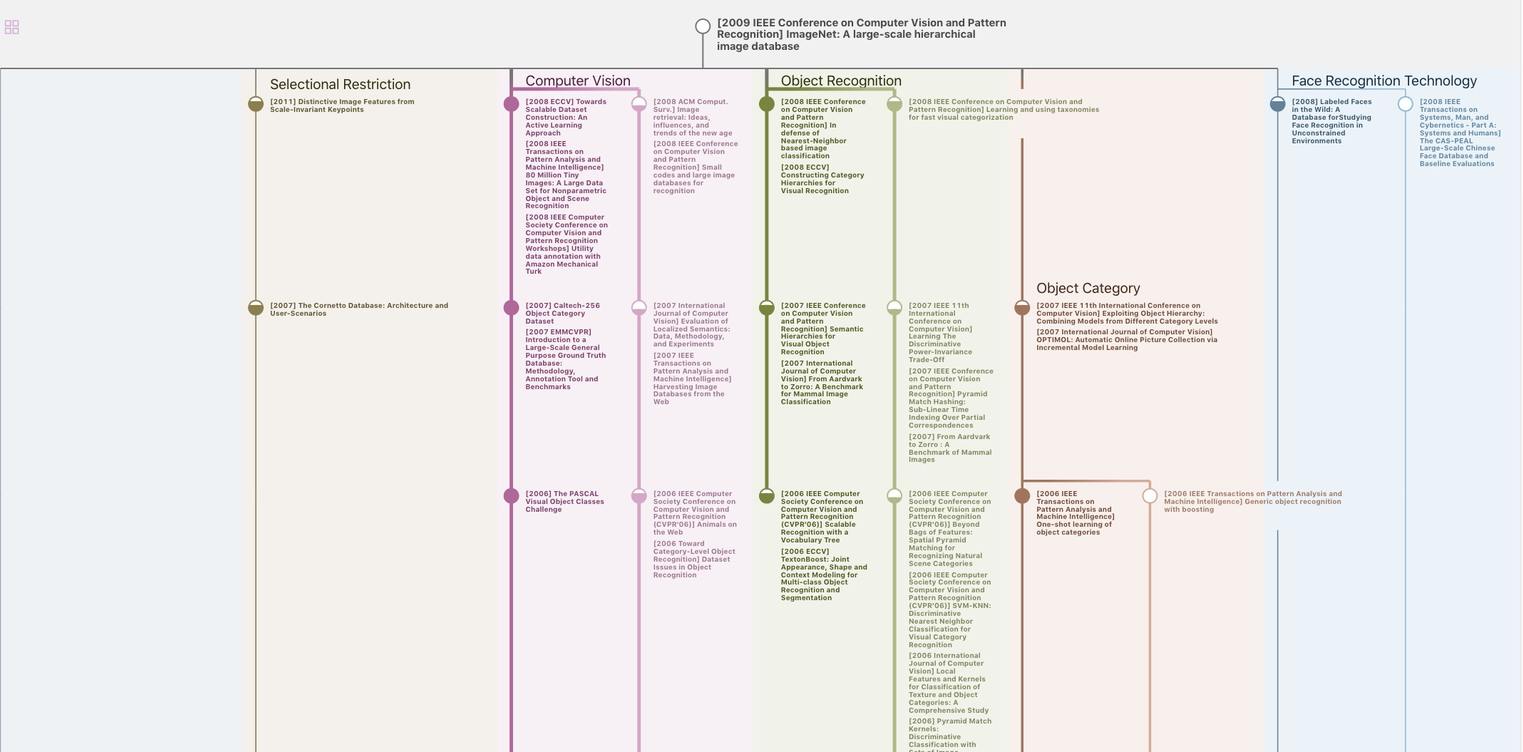
生成溯源树,研究论文发展脉络
Chat Paper
正在生成论文摘要