Mutual Information and Diverse Decoding Improve Neural Machine Translation
CoRR(2016)
摘要
Sequence-to-sequence neural translation models learn semantic and syntactic relations between sentence pairs by optimizing the likelihood of the target given the source, i.e., $p(y|x)$, an objective that ignores other potentially useful sources of information. We introduce an alternative objective function for neural MT that maximizes the mutual information between the source and target sentences, modeling the bi-directional dependency of sources and targets. We implement the model with a simple re-ranking method, and also introduce a decoding algorithm that increases diversity in the N-best list produced by the first pass. Applied to the WMT German/English and French/English tasks, the proposed models offers a consistent performance boost on both standard LSTM and attention-based neural MT architectures.
更多查看译文
AI 理解论文
溯源树
样例
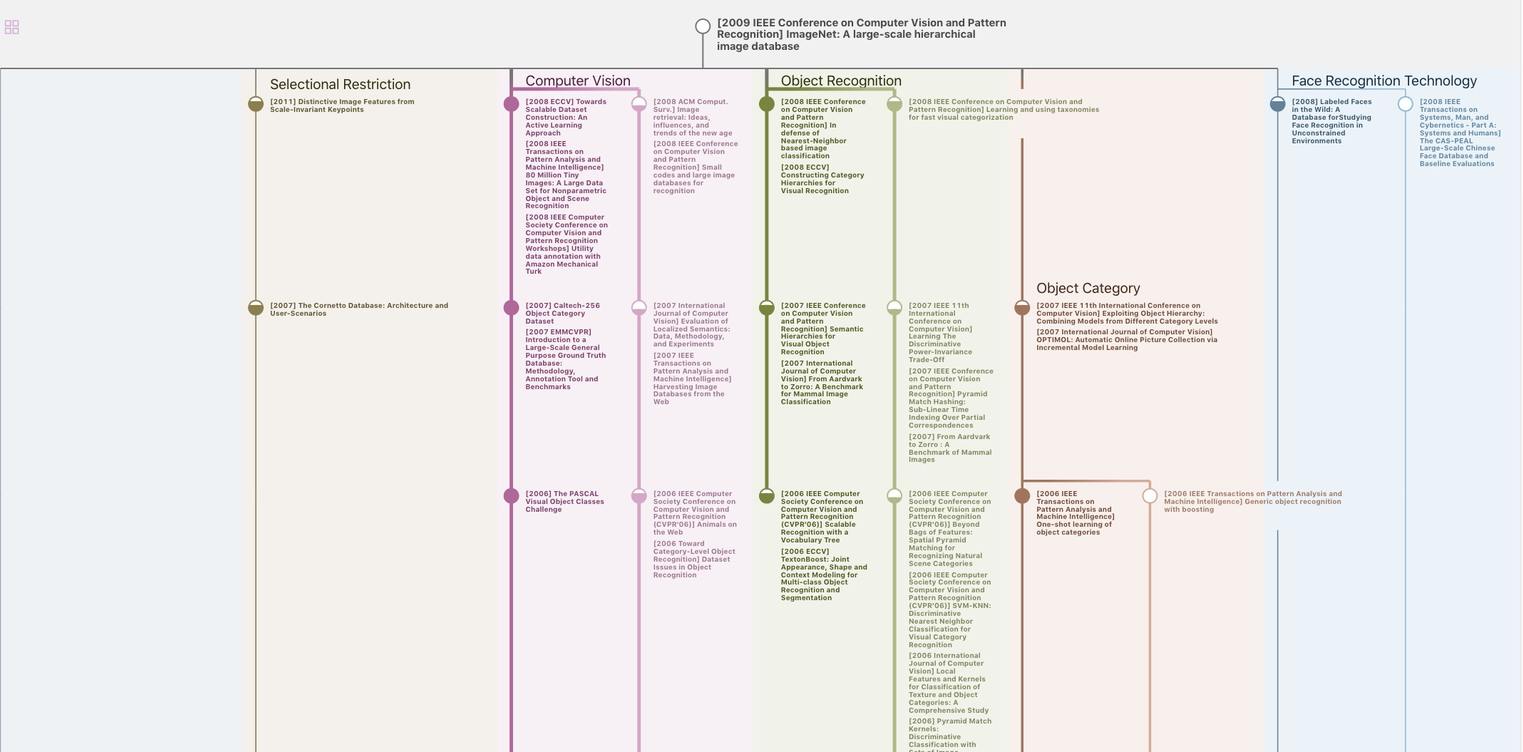
生成溯源树,研究论文发展脉络
Chat Paper
正在生成论文摘要