Compatible Value Gradients for Reinforcement Learning of Continuous Deep Policies
CoRR(2015)
摘要
This paper proposes GProp, a deep reinforcement learning algorithm for continuous policies with compatible function approximation. The algorithm is based on two innovations. Firstly, we present a temporal-difference based method for learning the gradient of the value-function. Secondly, we present the deviator-actor-critic (DAC) model, which comprises three neural networks that estimate the value function, its gradient, and determine the actor's policy respectively. We evaluate GProp on two challenging tasks: a contextual bandit problem constructed from nonparametric regression datasets that is designed to probe the ability of reinforcement learning algorithms to accurately estimate gradients; and the octopus arm, a challenging reinforcement learning benchmark. GProp is competitive with fully supervised methods on the bandit task and achieves the best performance to date on the octopus arm.
更多查看译文
关键词
continuous deep,reinforcement learning,value,policies
AI 理解论文
溯源树
样例
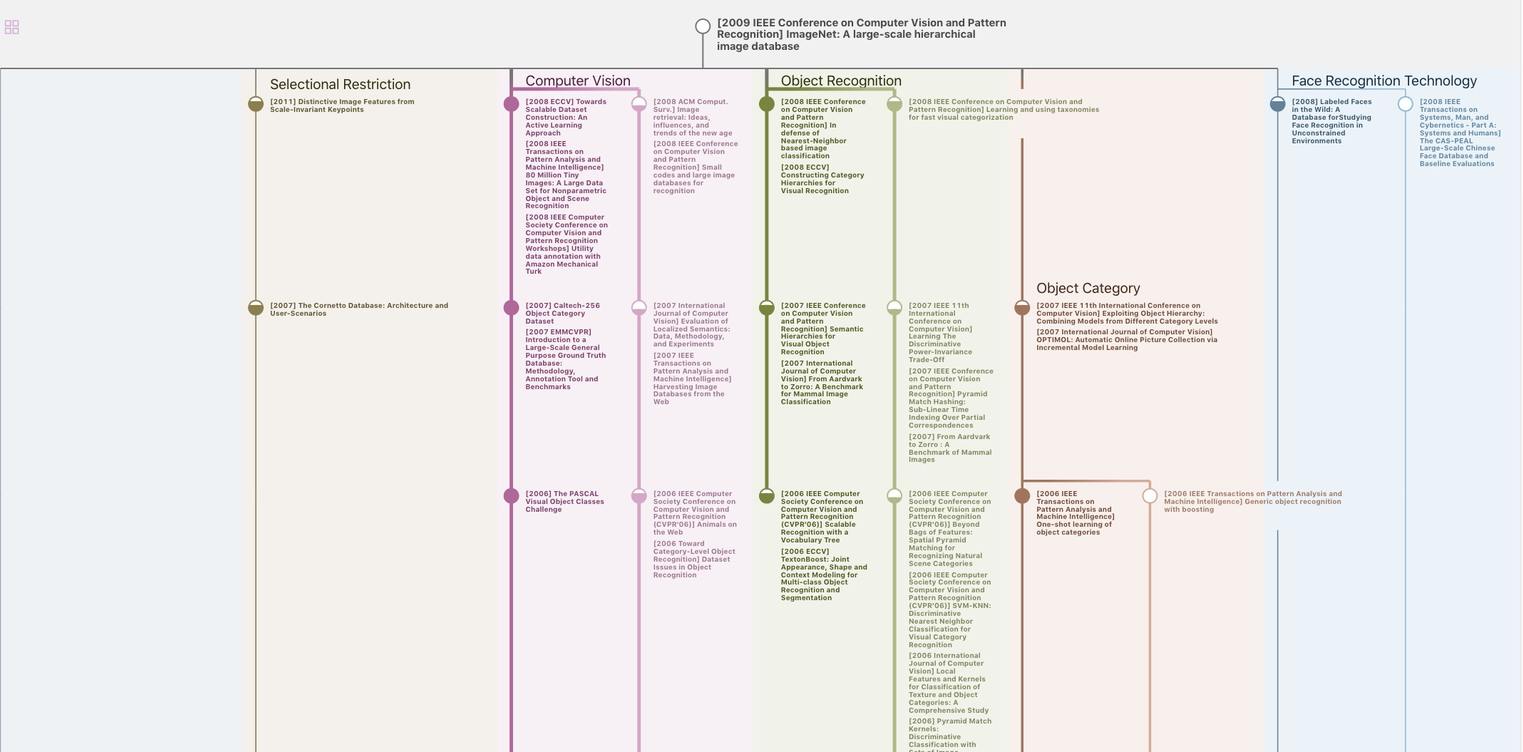
生成溯源树,研究论文发展脉络
Chat Paper
正在生成论文摘要