Clustering Subgaussian Mixtures By Semidefinite Programming
INFORMATION AND INFERENCE-A JOURNAL OF THE IMA(2017)
摘要
We introduce a model-free relax-and-round algorithm for k-means clustering based on a semidefinite relaxation due to Peng and Wei (2007, SIAM J. Optim., 18, 186-205). The algorithm interprets the output of the semidefinite program as a denoised version of the original data and then rounds this output to a hard clustering. We provide a generic method for proving performance guarantees for this algorithm, and we analyse the algorithm in the context of subgaussian mixture models. We also study the fundamental limits of estimating Gaussian centers by k-means clustering to compare our approximation guarantee to the theoretically optimal k-means clustering solution.
更多查看译文
关键词
clustering, machine learning, semidefinite programming, approximation algorithm
AI 理解论文
溯源树
样例
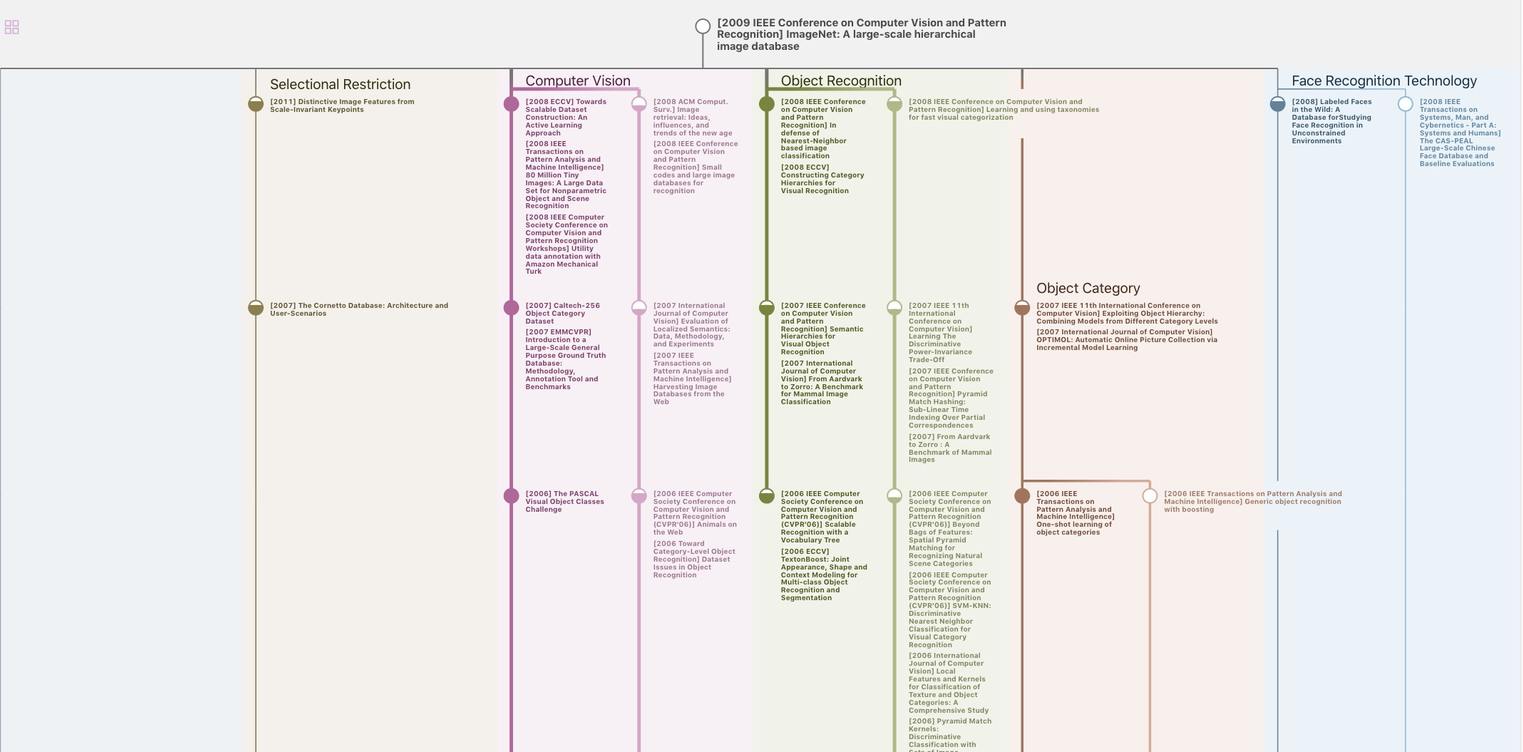
生成溯源树,研究论文发展脉络
Chat Paper
正在生成论文摘要