A Comparison between Deep Neural Nets and Kernel Acoustic Models for Speech Recognition
2016 IEEE International Conference on Acoustics, Speech and Signal Processing (ICASSP)(2016)
摘要
We study large-scale kernel methods for acoustic modeling and compare to DNNs on performance metrics related to both acoustic modeling and recognition. Measuring perplexity and frame-level classification accuracy, kernel-based acoustic models are as effective as their DNN counterparts. However, on token-error-rates DNN models can be significantly better. We have discovered that this might be attributed to DNN's unique strength in reducing both the perplexity and the entropy of the predicted posterior probabilities. Motivated by our findings, we propose a new technique, entropy regularized perplexity, for model selection. This technique can noticeably improve the recognition performance of both types of models, and reduces the gap between them. While effective on Broadcast News, this technique could be also applicable to other tasks.
更多查看译文
关键词
deep neural networks,kernel methods,acoustic models,automatic speech recognition
AI 理解论文
溯源树
样例
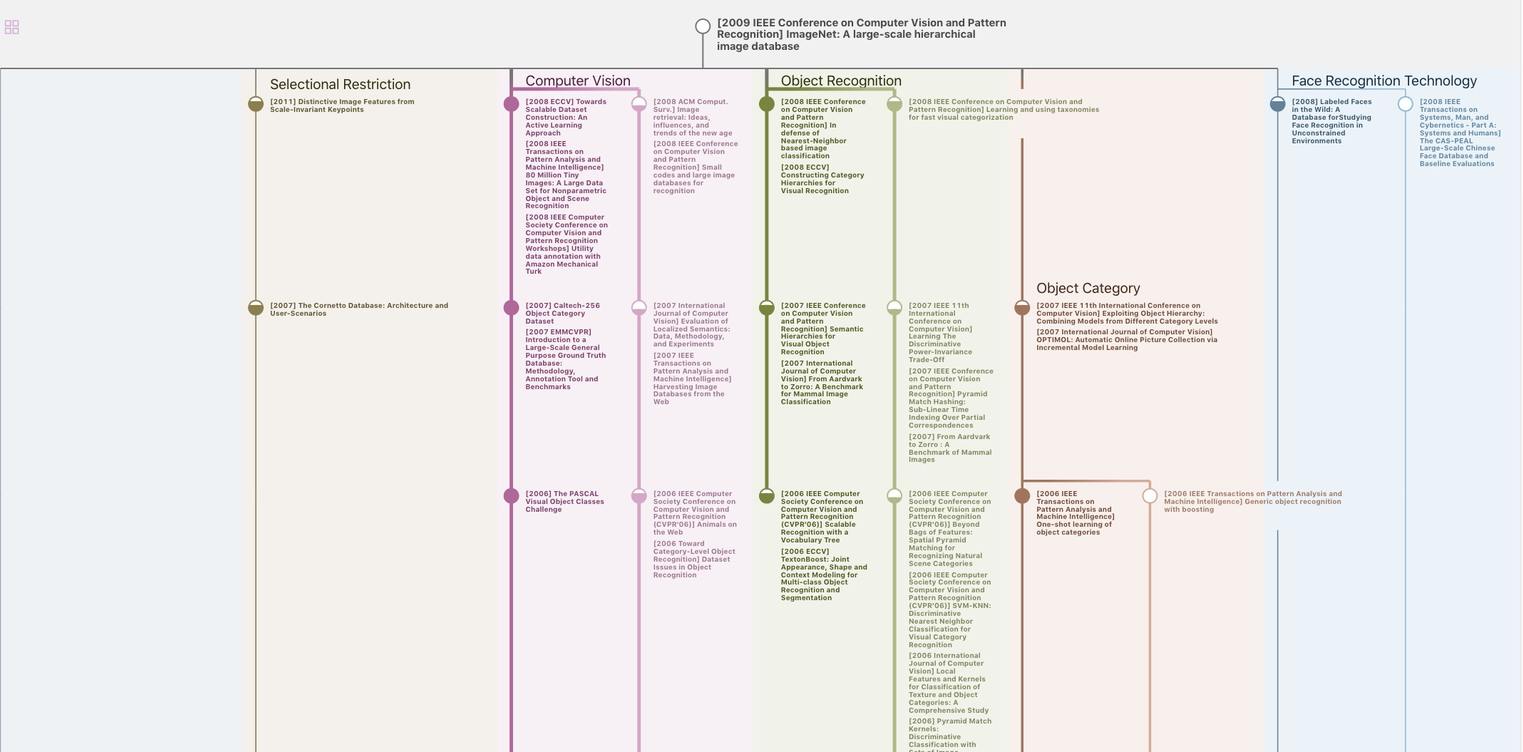
生成溯源树,研究论文发展脉络
Chat Paper
正在生成论文摘要