Learning multi-faceted representations of individuals from heterogeneous evidence using neural networks
CoRR(2015)
摘要
Inferring latent attributes of people online is an important social computing task, but requires integrating the many heterogeneous sources of information available on the web. We propose to learn individual representations of people using neural nets to integrate information from social media. The algorithm is able to combine any kind of cues, such as the text a person writes, the person's attributes (e.g. gender, employer, school, location) and social relations to other people (e.g., friendship, marriage), using global inference to infer missing attributes from noisy cues. The resulting latent representations capture homophily: people who have similar attributes, are related socially, or write similar text are closer in vector space. We show that these learned representations offer good performance at solving four important tasks in social media inference on Twitter: predicting (1) gender, (2) occupation, (3) location, and (4) friendships for users, and that we achieve the best performance by integrating all these signals. Our approach scales to large datasets, using parallel stochastic gradient descent for learning. The resulting representations can be used as general features in and have the potential to benefit a large number of downstream tasks like link prediction, community detection, or reasoning over social networks, discovering for example the high probability that a New York City resident is a fan of the New York Knicks, or the greater preference for iPhones by computer professionals than legal professionals.
更多查看译文
AI 理解论文
溯源树
样例
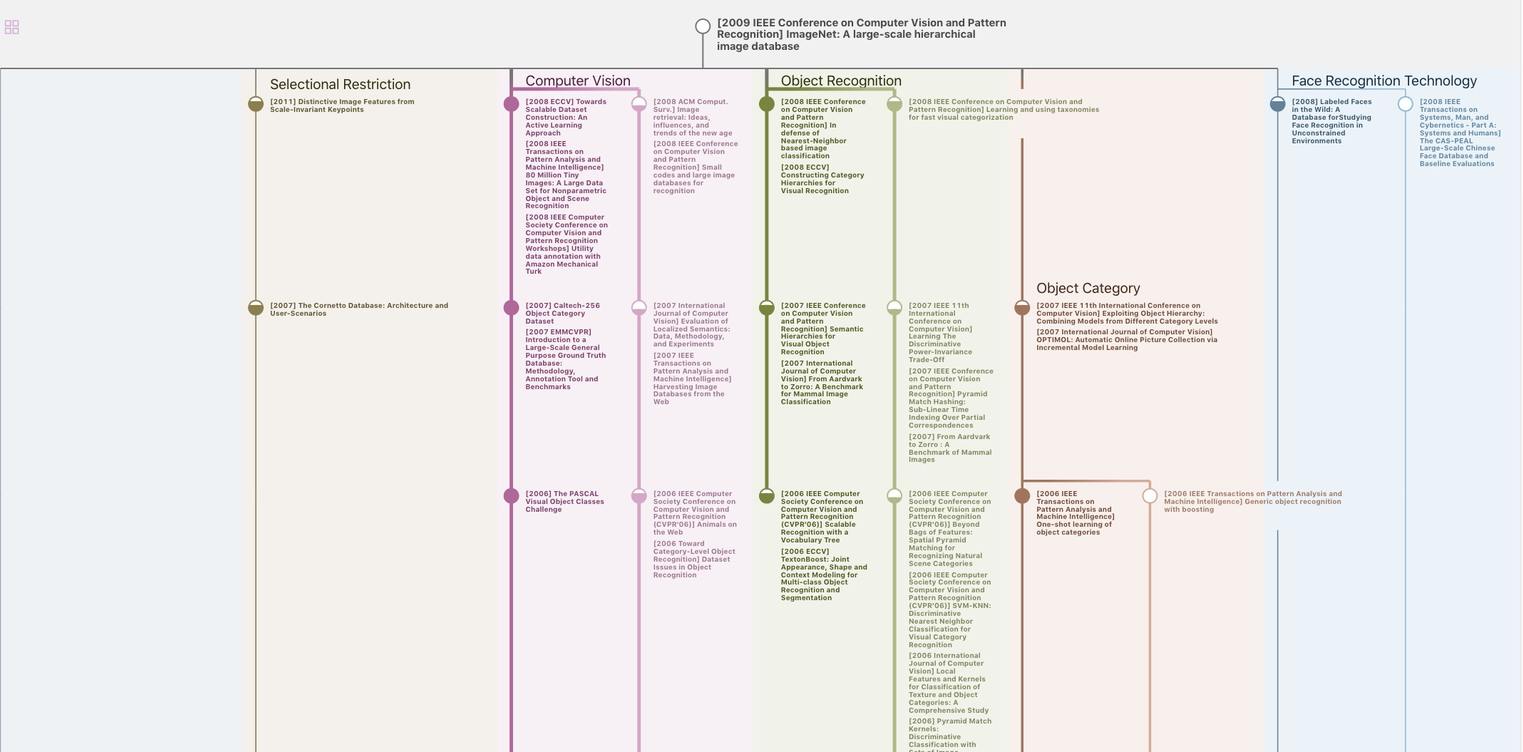
生成溯源树,研究论文发展脉络
Chat Paper
正在生成论文摘要