Minimax Subsampling for Estimation and Prediction in Low-Dimensional Linear Regression.
arXiv: Machine Learning(2016)
摘要
Subsampling strategies are derived to sample a small portion of design (data) points in a low-dimensional linear regression model $y=Xbeta+varepsilon$ with near-optimal statistical rates. Our results apply to both problems of estimation of the underlying linear model $beta$ and predicting the real-valued response $y$ of a new data point $x$. The derived subsampling strategies are minimax optimal under the fixed design setting, up to a small $(1+epsilon)$ relative factor. We also give interpretable subsampling probabilities for the random design setting and demonstrate explicit gaps in statistial rates between optimal and baseline (e.g., uniform) subsampling methods.
更多查看译文
AI 理解论文
溯源树
样例
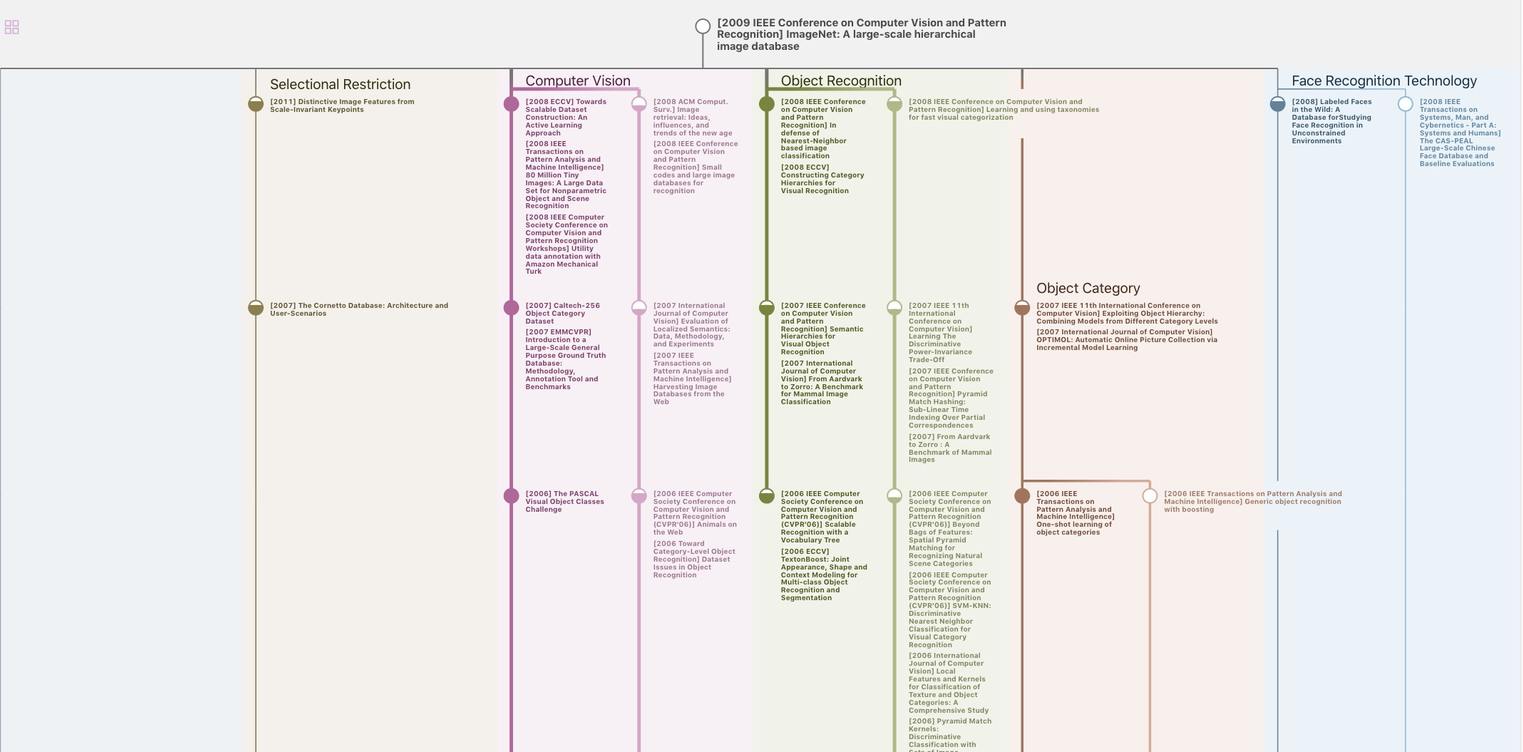
生成溯源树,研究论文发展脉络
Chat Paper
正在生成论文摘要