Convexity Shape Constraints for Image Segmentation
2016 IEEE Conference on Computer Vision and Pattern Recognition (CVPR)(2016)
摘要
Segmenting an image into multiple components is a central task in computer vision. In many practical scenarios, prior knowledge about plausible components is available. Incorporating such prior knowledge into models and algorithms for image segmentation is highly desirable, yet can be non-trivial. In this work, we introduce a new approach that allows, for the first time, to constrain some or all components of a segmentation to have convex shapes. Specifically, we extend the Minimum Cost Multicut Problem by a class of constraints that enforce convexity. To solve instances of this NP-hard integer linear program to optimality, we separate the proposed constraints in the branch-and-cut loop of a state-of-the-art ILP solver. Results on photographs and micrographs demonstrate the effectiveness of the approach as well as its advantages over the state-of-the-art heuristic.
更多查看译文
关键词
convexity shape constraints,image segmentation,computer vision,plausible components,minimum cost multicut problem,NP-hard integer linear program,branch-and-cut loop
AI 理解论文
溯源树
样例
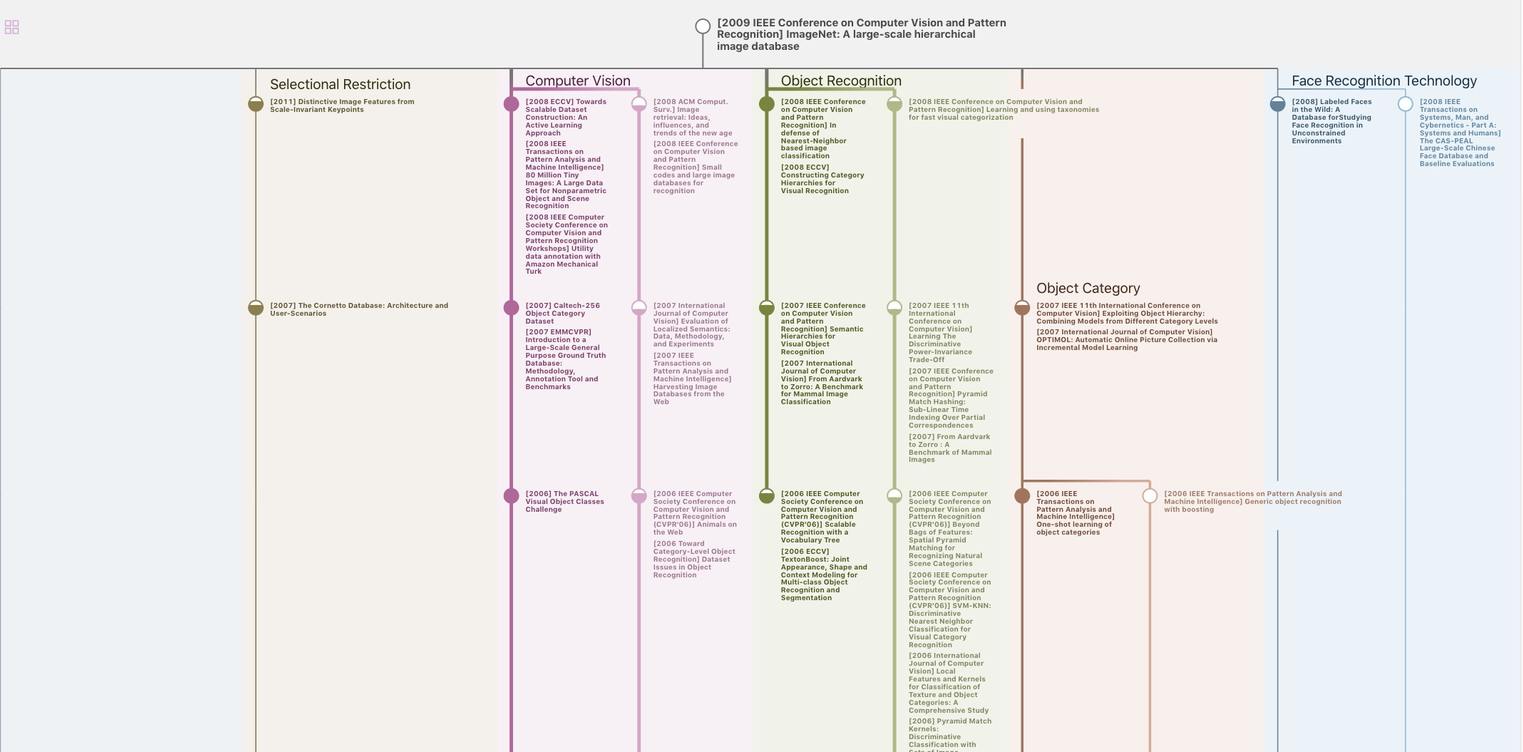
生成溯源树,研究论文发展脉络
Chat Paper
正在生成论文摘要