On sparsity by NUV-EM, Gaussian message passing, and Kalman smoothing
2016 Information Theory and Applications Workshop (ITA)(2016)
摘要
Normal priors with unknown variance (NUV) have long been known to promote sparsity and to blend well with parameter learning by expectation maximization (EM). In this paper, we advocate this approach for linear state space models for applications such as the estimation of impulsive signals, the detection of localized events, smoothing with occasional jumps in the state space, and the detection and removal of outliers. The actual computations boil down to multivariate-Gaussian message passing algorithms that are closely related to Kalman smoothing. We give improved tables of Gaussian-message computations from which such algorithms are easily synthesized, and we point out two preferred such algorithms.
更多查看译文
关键词
Gaussian message passing,Kalman smoothing,normal priors with unknown variance,NUV,parameter learning,expectation maximization,EM,linear state space models
AI 理解论文
溯源树
样例
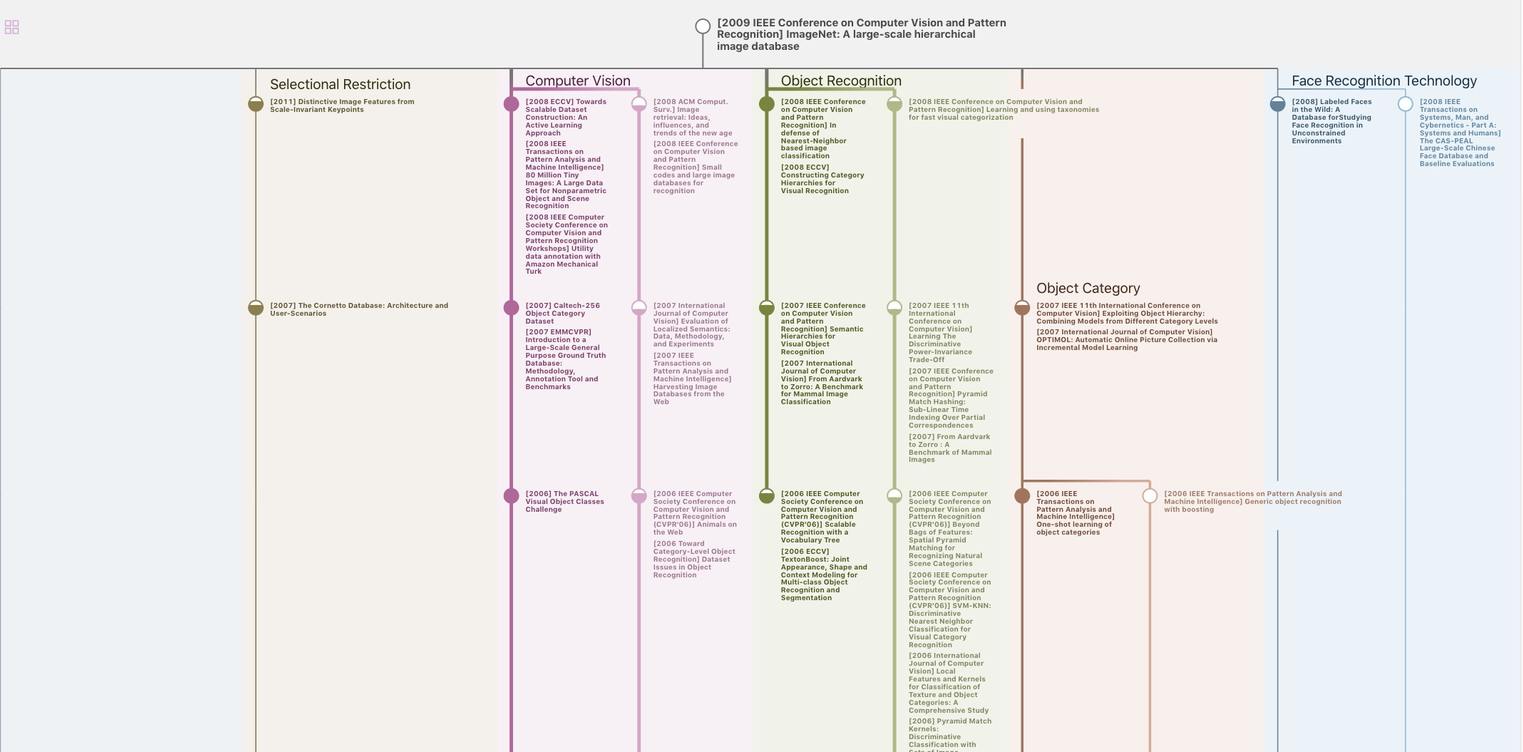
生成溯源树,研究论文发展脉络
Chat Paper
正在生成论文摘要