High precision grasp pose detection in dense clutter
2016 IEEE/RSJ International Conference on Intelligent Robots and Systems (IROS)(2016)
摘要
This paper considers the problem of grasp pose detection in point clouds. We follow a general algorithmic structure that first generates a large set of 6-DOF grasp candidates and then classifies each of them as a good or a bad grasp. Our focus in this paper is on improving the second step by using depth sensor scans from large online datasets to train a convolutional neural network. We propose two new representations of grasp candidates, and we quantify the effect of using prior knowledge of two forms: instance or category knowledge of the object to be grasped, and pretraining the network on simulated depth data obtained from idealized CAD models. Our analysis shows that a more informative grasp candidate representation as well as pretraining and prior knowledge significantly improve grasp detection. We evaluate our approach on a Baxter Research Robot and demonstrate an average grasp success rate of 93% in dense clutter. This is a 20% improvement compared to our prior work.
更多查看译文
关键词
high precision grasp pose detection,point clouds,grasp classification,depth sensor scan,convolutional neural network training,instance knowledge,category knowledge,CAD models,computer aided design models,Baxter Research Robot
AI 理解论文
溯源树
样例
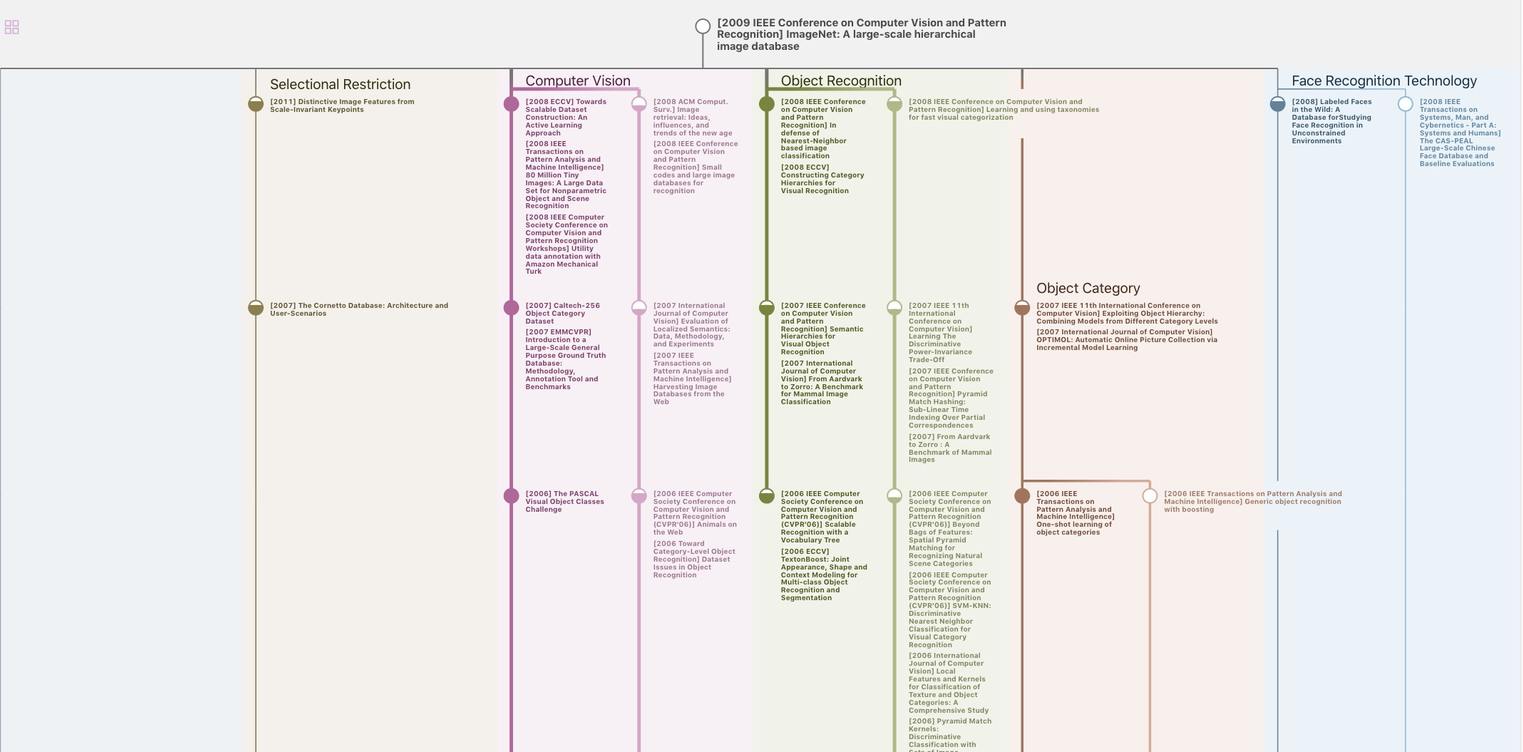
生成溯源树,研究论文发展脉络
Chat Paper
正在生成论文摘要