Learning $\ell^{0}$-Graph for Data Clustering
CoRR(2015)
摘要
$\ell^{1}$-graph \cite{YanW09,ChengYYFH10}, a sparse graph built by reconstructing each datum with all the other data using sparse representation, has been demonstrated to be effective in clustering high dimensional data and recovering independent subspaces from which the data are drawn. It is well known that $\ell^{1}$-norm used in $\ell^{1}$-graph is a convex relaxation of $\ell^{0}$-norm for enforcing the sparsity. In order to handle general cases when the subspaces are not independent and follow the original principle of sparse representation, we propose a novel $\ell^{0}$-graph that employs $\ell^{0}$-norm to encourage the sparsity of the constructed graph, and develop a proximal method to solve the associated optimization problem with the proved guarantee of convergence. Extensive experimental results on various data sets demonstrate the superiority of $\ell^{0}$-graph compared to other competing clustering methods including $\ell^{1}$-graph.
更多查看译文
关键词
data clustering,learning
AI 理解论文
溯源树
样例
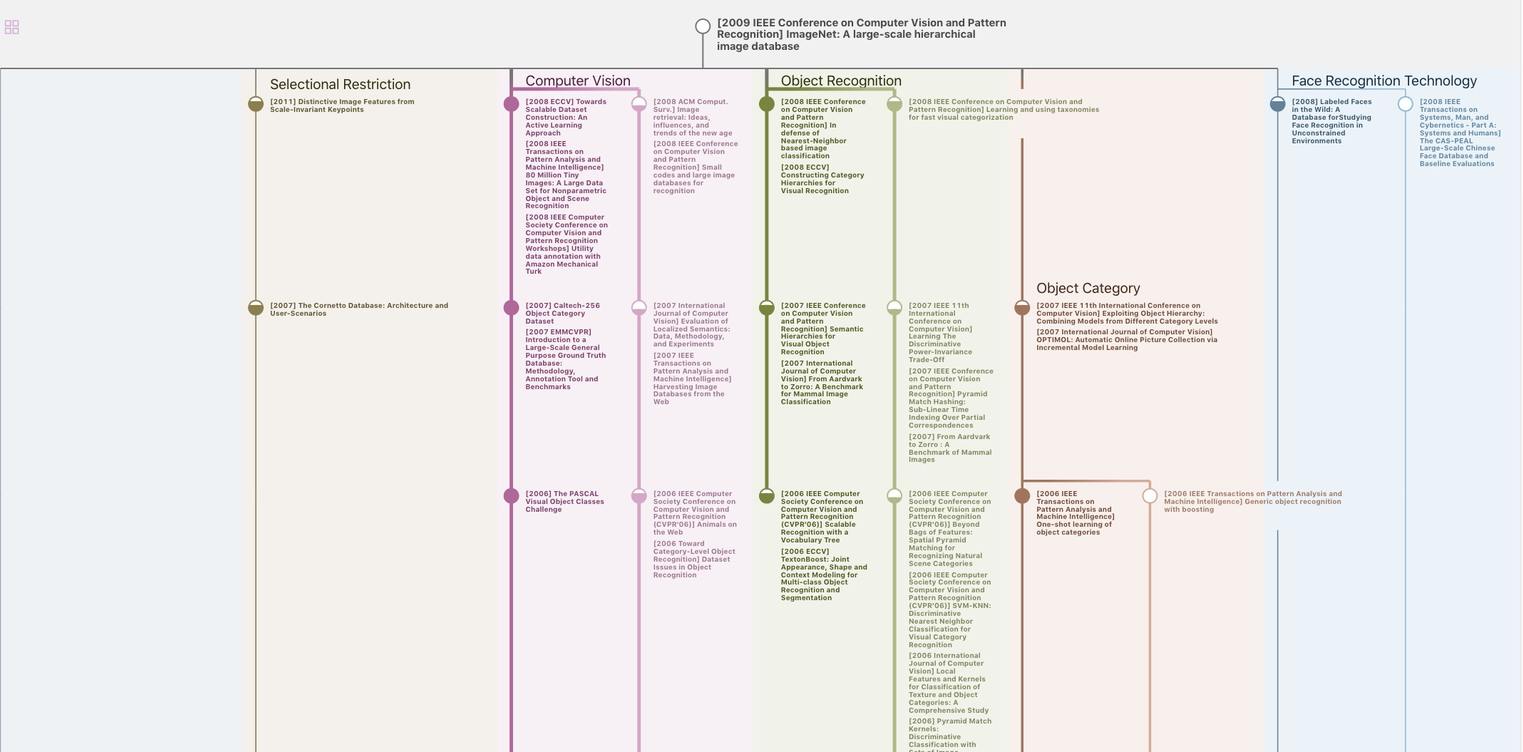
生成溯源树,研究论文发展脉络
Chat Paper
正在生成论文摘要