Model-Based Reinforcement Learning With Parametrized Physical Models And Optimism-Driven Exploration
2016 IEEE International Conference on Robotics and Automation (ICRA)(2016)
摘要
In this paper, we present a robotic model-based reinforcement learning method that combines ideas from model identification and model predictive control. We use a feature-based representation of the dynamics that allows the dynamics model to be fitted with a simple least squares procedure, and the features are identified from a high-level specification of the robot's morphology, consisting of the number and connectivity structure of its links. Model predictive control is then used to choose the actions under an optimistic model of the dynamics, which produces an efficient and goal-directed exploration strategy. We present real time experimental results on standard benchmark problems involving the pendulum, cartpole, and double pendulum systems. Experiments indicate that our method is able to learn a range of benchmark tasks substantially faster than the previous best methods. To evaluate our approach on a realistic robotic control task, we also demonstrate real time control of a simulated 7 degree of freedom arm.
更多查看译文
关键词
model-based reinforcement learning,model-based RL,model identification,model predictive control,optimism-driven exploration,feature-based representation,robot morphology,connectivity structure,cartpole system,double pendulum system,robotic control task,7 degree of freedom arm
AI 理解论文
溯源树
样例
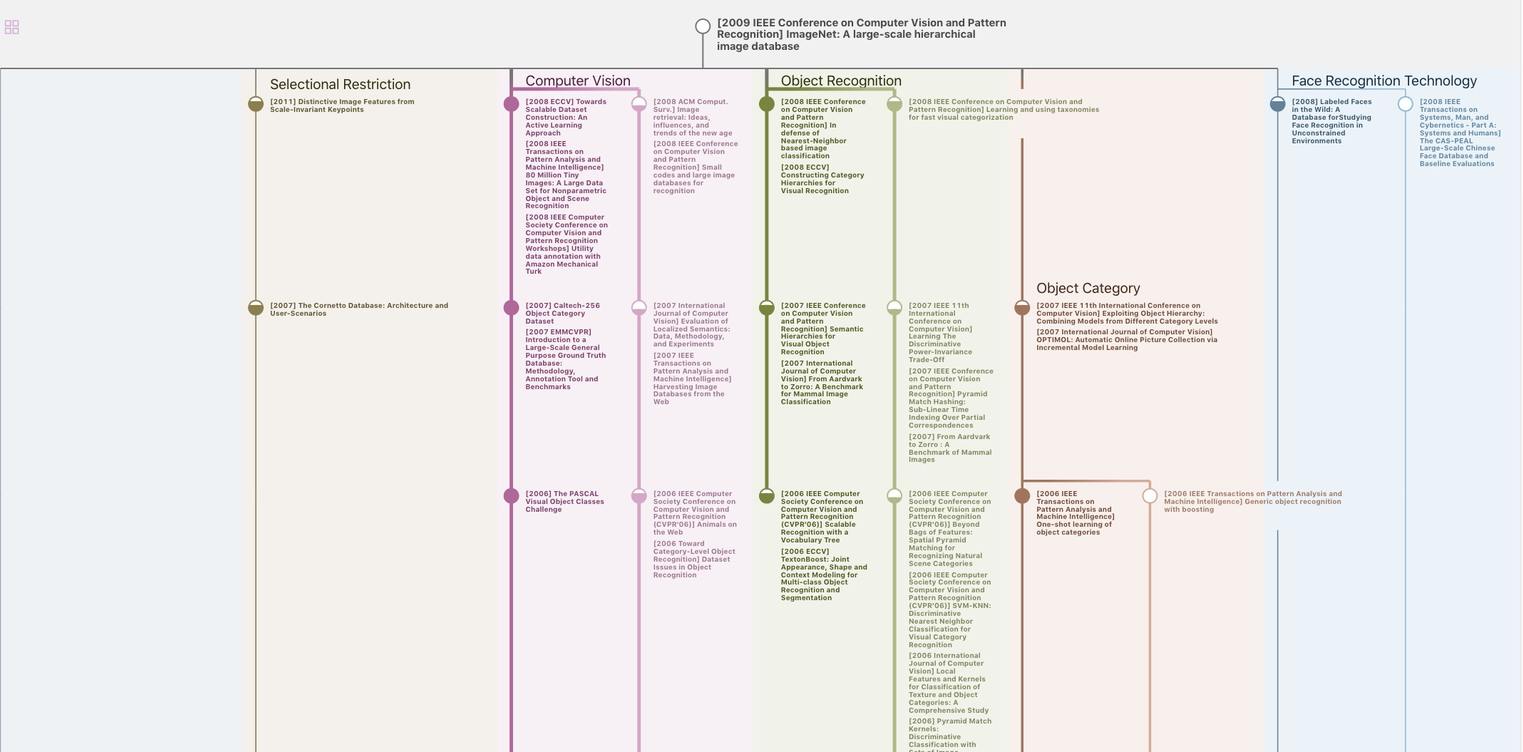
生成溯源树,研究论文发展脉络
Chat Paper
正在生成论文摘要