Scale-aware Pixel-wise Object Proposal Networks.
IEEE Transactions on Image Processing(2016)
摘要
Object proposal is essential for current state-of-the-art object detection pipelines. However, the existing proposal methods generally fail in producing results with satisfying localization accuracy. The case is even worse for small objects, which, however, are quite common in practice. In this paper, we propose a novel scale-aware pixelwise object proposal network (SPOP-net) to tackle the challenges. The SPOP-net can generate proposals with high recall rate and average best overlap, even for small objects. In particular, in order to improve the localization accuracy, a fully convolutional network is employed which predicts locations of object proposals for each pixel. The produced ensemble of pixelwise object proposals enhances the chance of hitting the object significantly without incurring heavy extra computational cost. To solve the challenge of localizing objects at small scale, two localization networks, which are specialized for localizing objects with different scales are introduced, following the divide-and-conquer philosophy. Location outputs of these two networks are then adaptively combined to generate the final proposals by a large-/small-size weighting network. Extensive evaluations on PASCAL VOC 2007 and COCO 2014 show the SPOP network is superior over the state-of-the-art models. The high-quality proposals from SPOP-net also significantly improve the mean average precision of object detection with Fast-Regions with CNN features framework. Finally, the SPOP-net (trained on PASCAL VOC) shows great generalization performance when testing it on ILSVRC 2013 validation set.
更多查看译文
关键词
Proposals,Image segmentation,Computational efficiency,Object detection,Neural networks,Image edge detection,Training
AI 理解论文
溯源树
样例
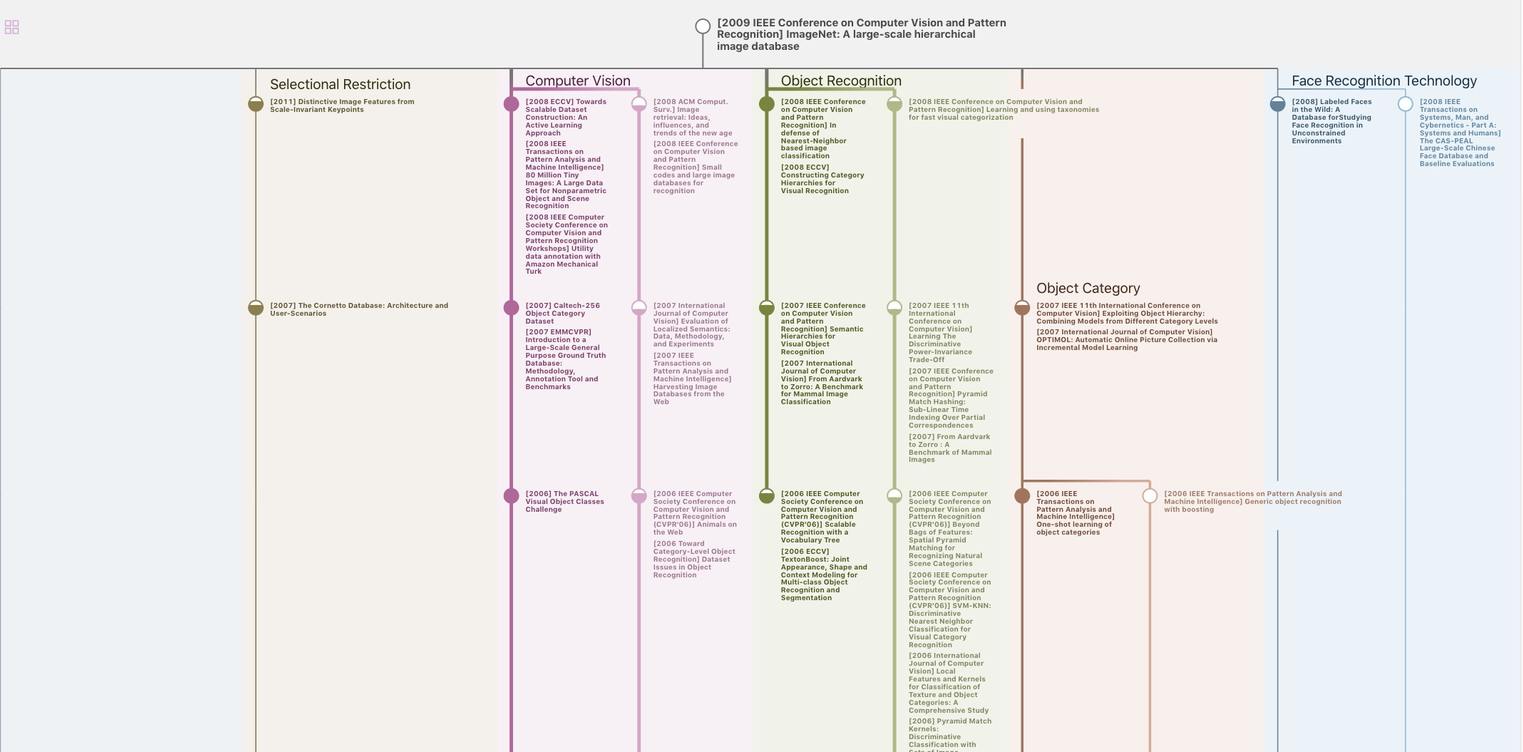
生成溯源树,研究论文发展脉络
Chat Paper
正在生成论文摘要