Graph-based predictable feature analysis
Machine Learning(2017)
摘要
We propose graph-based predictable feature analysis (GPFA), a new method for unsupervised learning of predictable features from high-dimensional time series, where high predictability is understood very generically as low variance in the distribution of the next data point given the previous ones. We show how this measure of predictability can be understood in terms of graph embedding as well as how it relates to the information-theoretic measure of predictive information in special cases. We confirm the effectiveness of GPFA on different datasets, comparing it to three existing algorithms with similar objectives—namely slow feature analysis, forecastable component analysis, and predictable feature analysis—to which GPFA shows very competitive results.
更多查看译文
关键词
Unsupervised learning,Dimensionality reduction,Feature learning,Representation learning,Graph embedding,Predictability
AI 理解论文
溯源树
样例
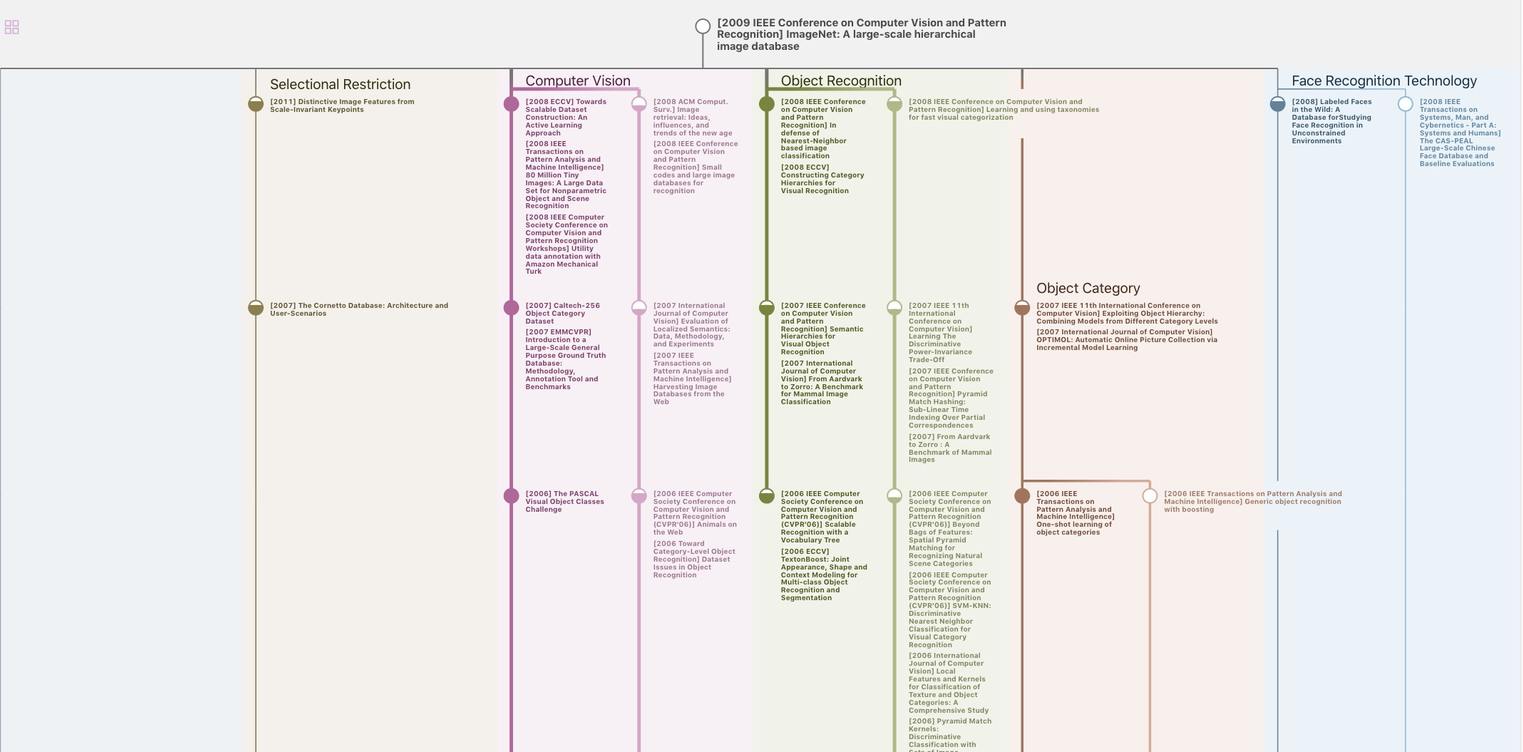
生成溯源树,研究论文发展脉络
Chat Paper
正在生成论文摘要