Graph Dependency Construction Based On Interval-Event Dependencies Detection In Data Streams
Intelligent Data Analysis(2016)
摘要
Pattern mining over data streams is critical to a variety of applications such as understanding and predicting weather phenomena or outdoor surveillance. Most of the current techniques attempt to discover relationships between time-point events but are not practical for discovering dependencies over interval-based events. In this work, we present a new approach to mine dependencies between streams of interval-based events that links two events if they occur in a similar manner, one being often followed by the other one after a certain time interval in the data. The proposed method is robust to temporal variability of events and determines the most appropriate time intervals whose validity is assessed by a. 2 test. As several intervals may redundantly describe the same dependency, the approach retrieves only the most specific intervals with respect to a dominance relationship over temporal dependencies, and thus avoids the classical problem of pattern flooding in data mining. The TEDDY algorithm, TEmporal Dependency DiscoverY, prunes the search space while guaranteeing the discovery of all valid and significant temporal dependencies. We present empirical results on simulated and real-life data to show the scalability and the robustness of our approach. The dependency relationships define a graph that supports intelligent analysis as illustrated by two case studies: Outdoor surveillance of a building via video camera and motion sensors, and assistance for road deicing operations based on the humidity and temperature measurements at the urban scale. These applications demonstrate the efficiency and the effectiveness of our approach.
更多查看译文
关键词
Temporal dependencies,data streams,sensor network analysis
AI 理解论文
溯源树
样例
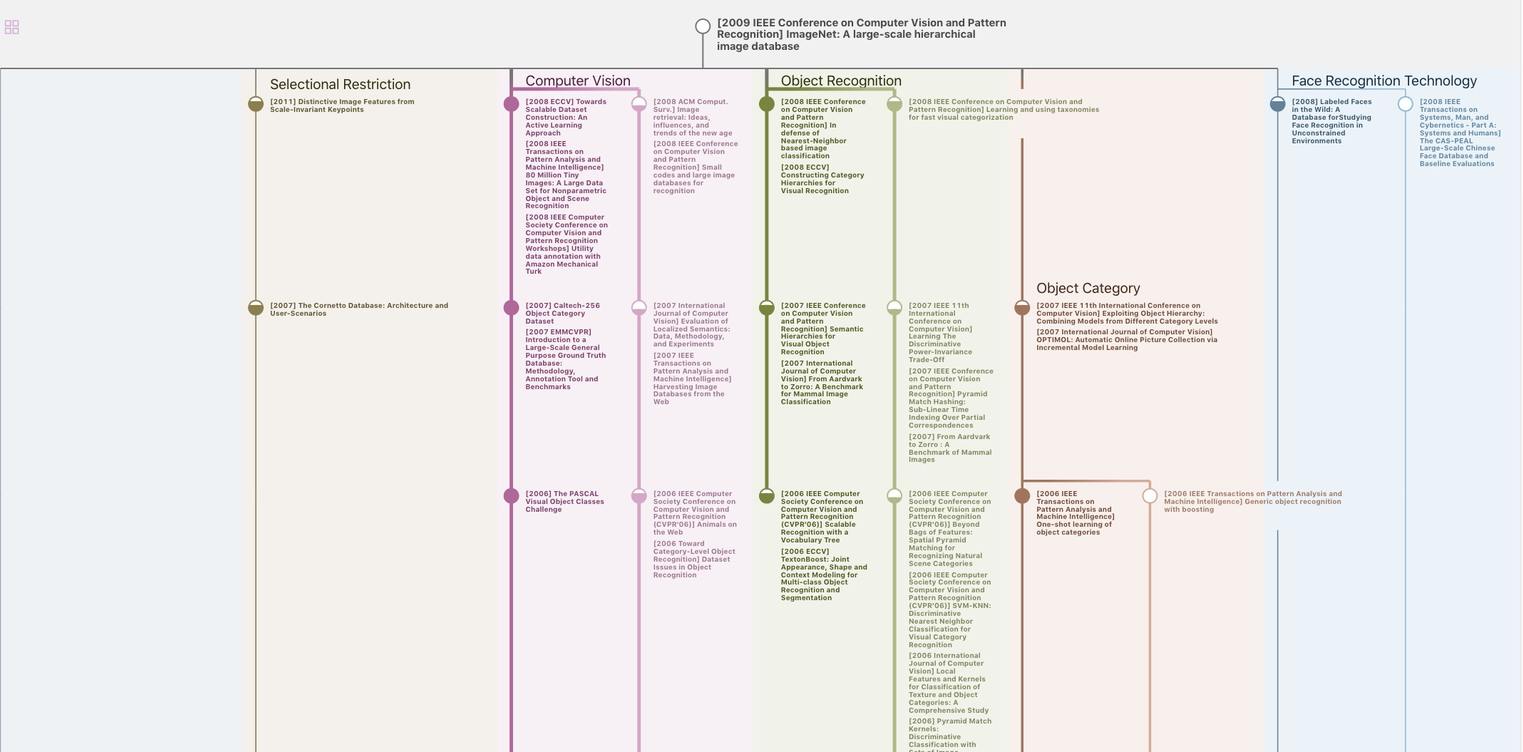
生成溯源树,研究论文发展脉络
Chat Paper
正在生成论文摘要