Finding Active Membership Functions For Genetic-Fuzzy Data Mining
INTERNATIONAL JOURNAL OF INFORMATION TECHNOLOGY & DECISION MAKING(2015)
摘要
Since transactions may contain quantitative values, many approaches have been proposed to derive membership functions for mining fuzzy association rules using genetic algorithms (GAs), a process known as genetic-fuzzy data mining. However, existing approaches assume that the number of linguistic terms is predefined. Thus, this study proposes a genetic-fuzzy mining approach for extracting an appropriate number of linguistic terms and their membership functions used in fuzzy data mining for the given items. The proposed algorithm adjusts membership functions using GAs and then uses them to fuzzify the quantitative transactions. Each individual in the population represents a possible set of membership functions for the items and is divided into two parts, control genes (CGs) and parametric genes (PGs). CGs are encoded into binary strings and used to determine whether membership functions are active. Each set of membership functions for an item is encoded as PGs with real-number schema. In addition, seven fitness functions are proposed, each of which is used to evaluate the goodness of the obtained membership functions and used as the evolutionary criteria in GA. After the GA process terminates, a better set of association rules with a suitable set of membership functions is obtained. Experiments are made to show the effectiveness of the proposed approach.
更多查看译文
关键词
Data mining, fuzzy sets, fuzzy association rules, genetic algorithms, membership functions
AI 理解论文
溯源树
样例
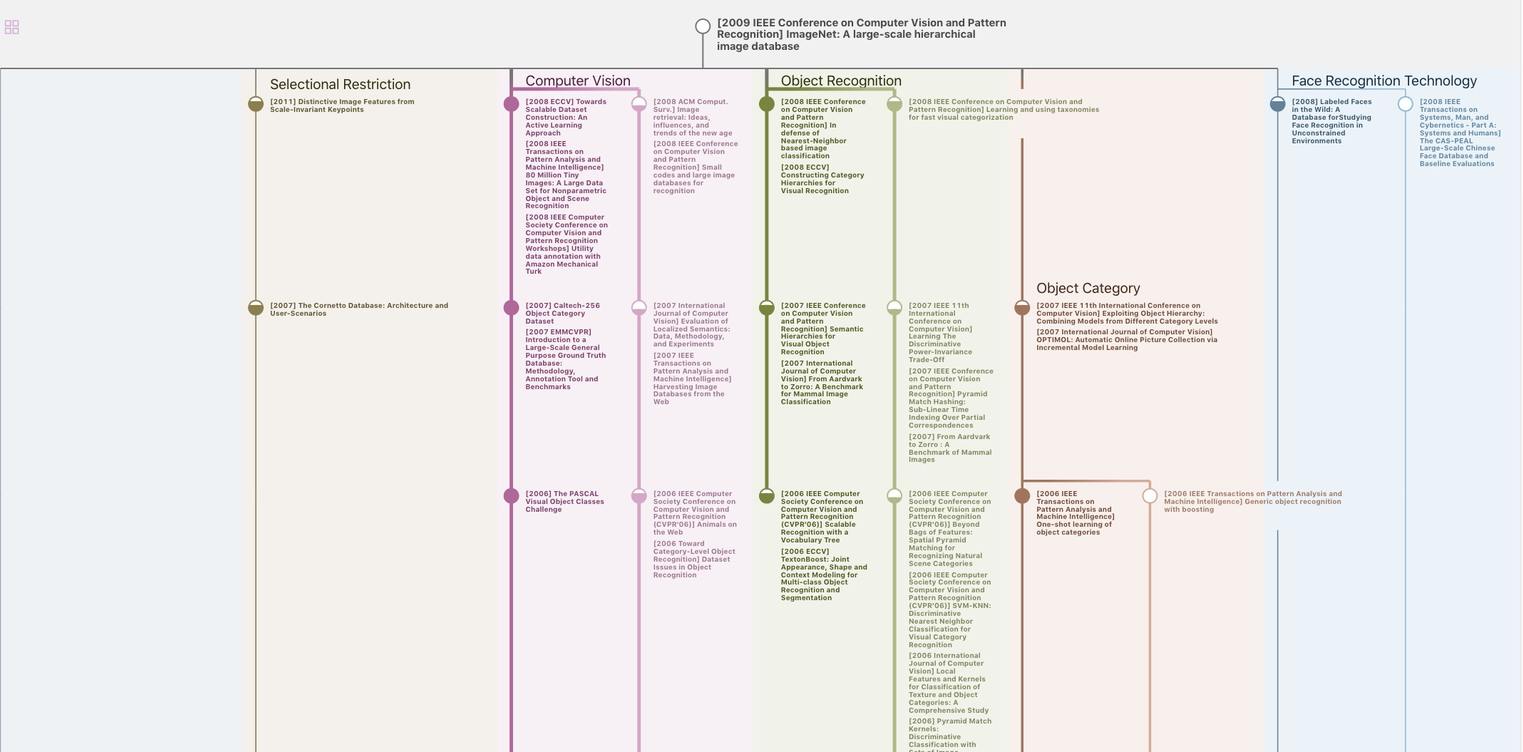
生成溯源树,研究论文发展脉络
Chat Paper
正在生成论文摘要