Learning Similarity with Probabilistic Latent Semantic Analysis for Image Retrieval.
KSII TRANSACTIONS ON INTERNET AND INFORMATION SYSTEMS(2015)
摘要
It is a challenging problem to search the intended images from a large number of candidates. Content based image retrieval (CBIR) is the most promising way to tackle this problem, where the most important topic is to measure the similarity of images so as to cover the variance of shape, color, pose, illumination etc. While previous works made significant progresses, their adaption ability to dataset is not fully explored. In this paper, we propose a similarity learning method on the basis of probabilistic generative model, i.e., probabilistic latent semantic analysis (PLSA). It first derives Fisher kernel, a function over the parameters and variables, based on PLSA. Then, the parameters are determined through simultaneously maximizing the log likelihood function of PLSA and the retrieval performance over the training dataset. The main advantages of this work are twofold: (1) deriving similarity measure based on PLSA which fully exploits the data distribution and Bayes inference; (2) learning model parameters by maximizing the fitting of model to data and the retrieval performance simultaneously. The proposed method (PLSA-FK) is empirically evaluated over three datasets, and the results exhibit promising performance.
更多查看译文
关键词
Image retrieval,PLSA,Fisher kernel,similarity measure learning
AI 理解论文
溯源树
样例
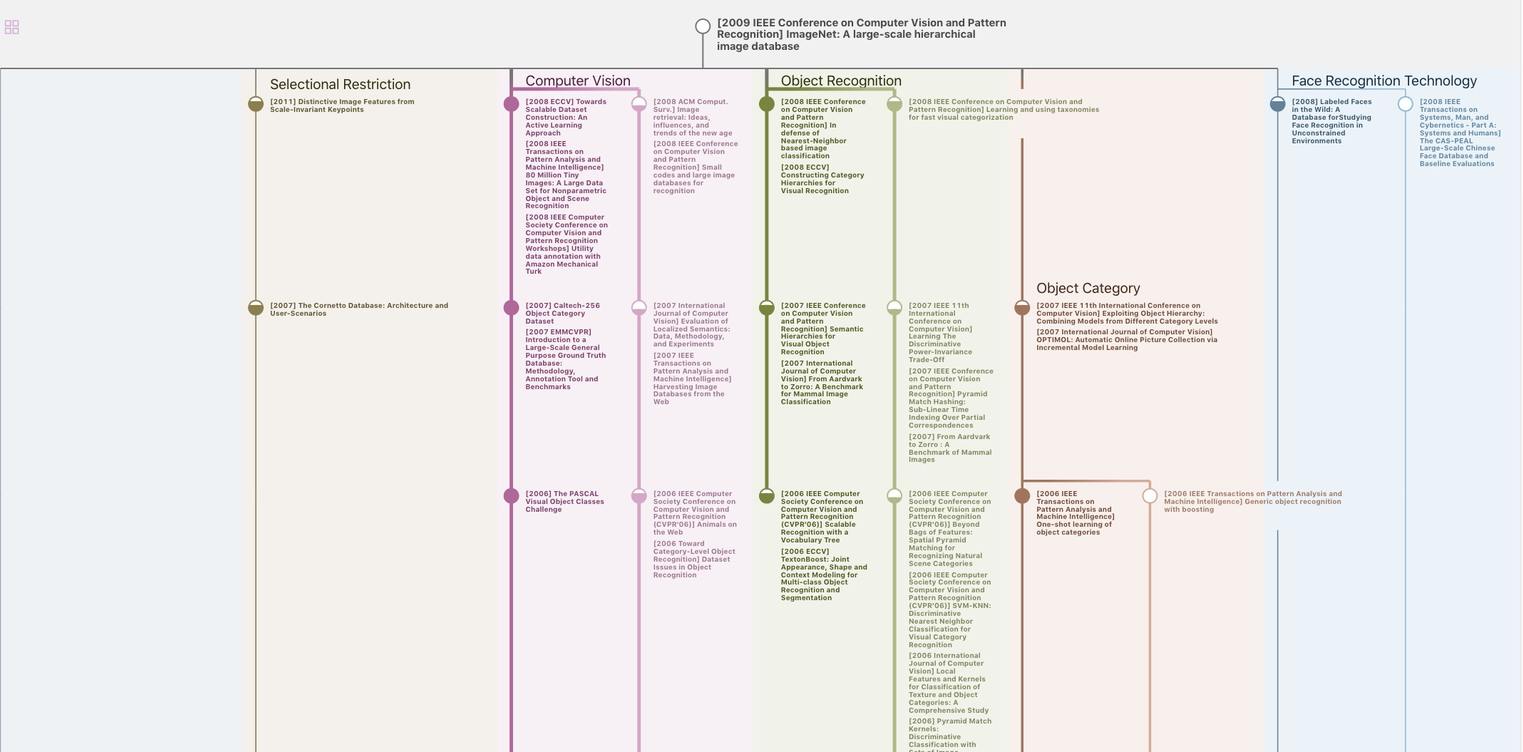
生成溯源树,研究论文发展脉络
Chat Paper
正在生成论文摘要