Multimodal video classification with stacked contractive autoencoders
Signal Processing(2016)
摘要
In this paper we propose a multimodal feature learning mechanism based on deep networks (i.e., stacked contractive autoencoders) for video classification. Considering the three modalities in video, i.e., image, audio and text, we first build one Stacked Contractive Autoencoder (SCAE) for each single modality, whose outputs will be joint together and fed into another Multimodal Stacked Contractive Autoencoder (MSCAE). The first stage preserves intra-modality semantic relations and the second stage discovers inter-modality semantic correlations. Experiments on real world dataset demonstrate that the proposed approach achieves better performance compared with the state-of-the-art methods. HighlightsA two-stage framework for multimodal video classification is proposed.The model is built based on stacked contractive autoencoders.The first stage is single modal pre-training.The second stage is multimodal fine-tuning.The objective functions are optimized by stochastic gradient descent.
更多查看译文
关键词
Multimodal,Video classification,Deep learning,Stacked contractive autoencoder
AI 理解论文
溯源树
样例
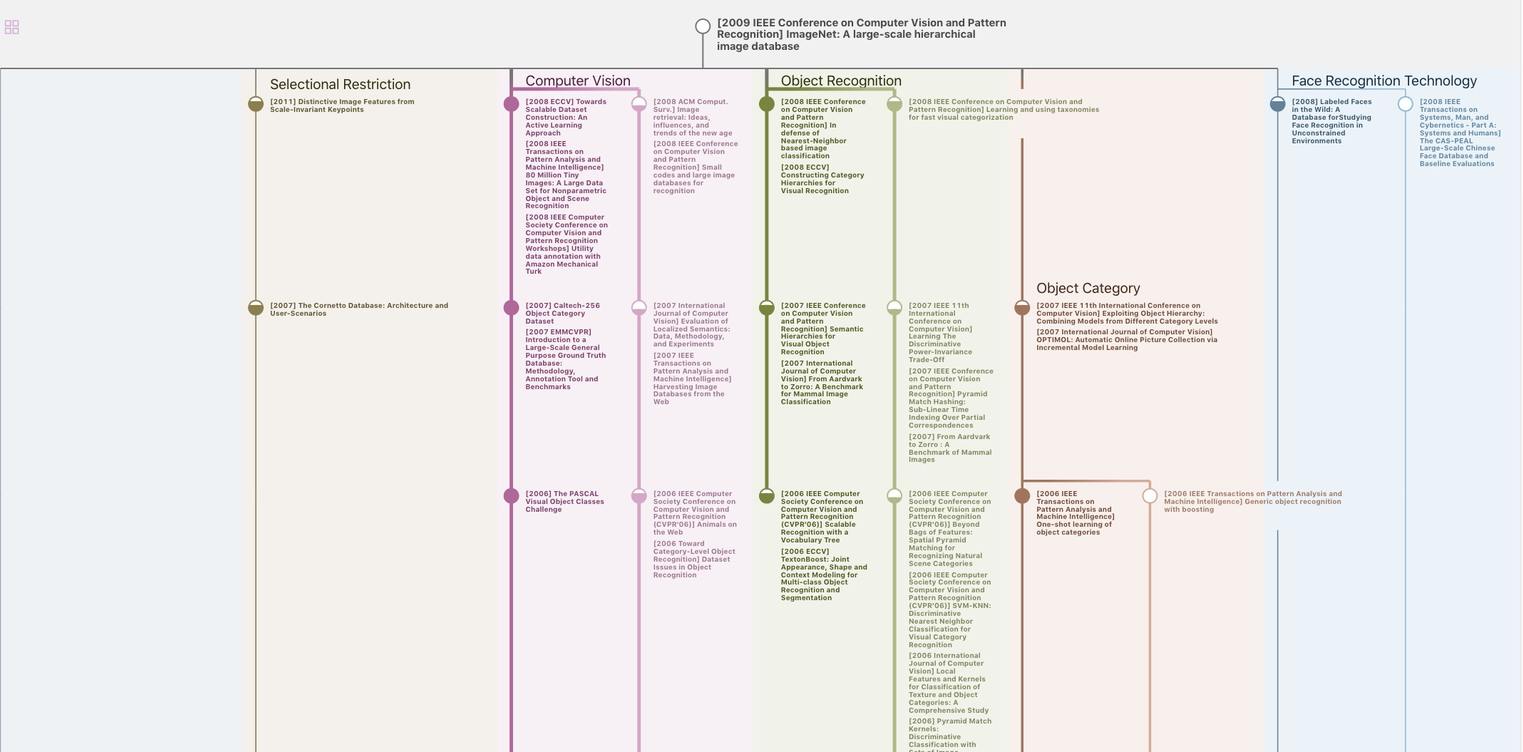
生成溯源树,研究论文发展脉络
Chat Paper
正在生成论文摘要