Measuring Temporal Patterns in Dynamic Social Networks
ACM Transactions on Knowledge Discovery from Data(2015)
摘要
Given social networks over time, how can we measure network activities across different timesteps with a limited number of metrics? We propose two classes of dynamic metrics for assessing temporal evolution patterns of agents in terms of persistency and emergence. For each class of dynamic metrics, we implement it using three different temporal aggregation models ranging from the most commonly used Average Aggregation Model to more the complex models such as the Exponential Aggregation Model. We argue that the problem of measuring temporal patterns can be formulated using Recency and Primacy effect, which is a concept used to characterize human cognitive processes. Experimental results show that the way metrics model Recency--Primacy effect is closely related to their abilities to measure temporal patterns. Furthermore, our results indicate that future network agent activities can be predicted based on history information using dynamic metrics. By conducting multiple experiments, we are also able to find an optimal length of history information that is most relevant to future activities. This optimal length is highly consistent within a dataset and can be used as an intrinsic metric to evaluate a dynamic social network.
更多查看译文
关键词
Measurement,Algorithms,Performance,Social network analysis,aggregating method,temporal analysis,Primacy and Recency effects
AI 理解论文
溯源树
样例
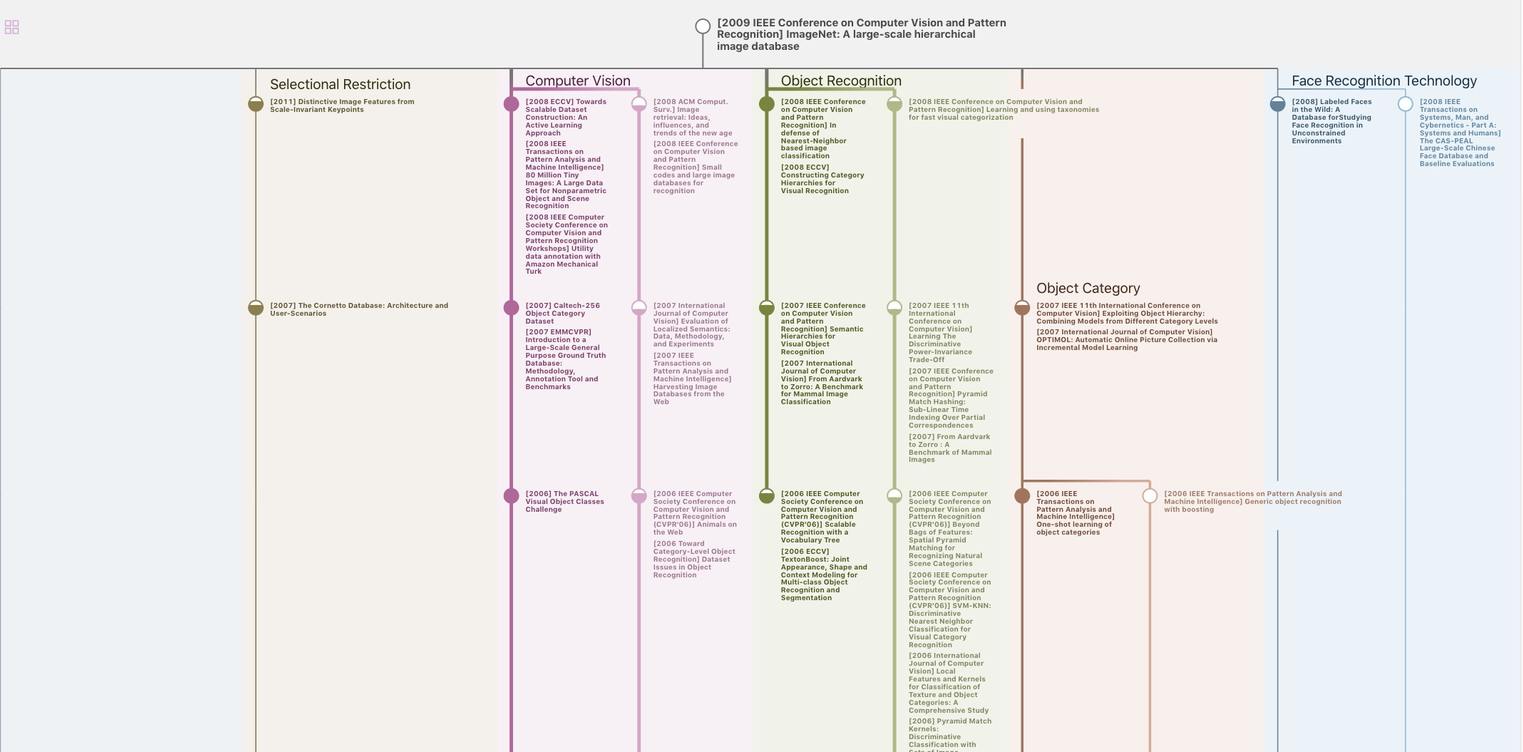
生成溯源树,研究论文发展脉络
Chat Paper
正在生成论文摘要